Clustering Fragments Metagenome Using Self-Organizing Map
Indonesian Journal of Electrical Engineering and Computer Science
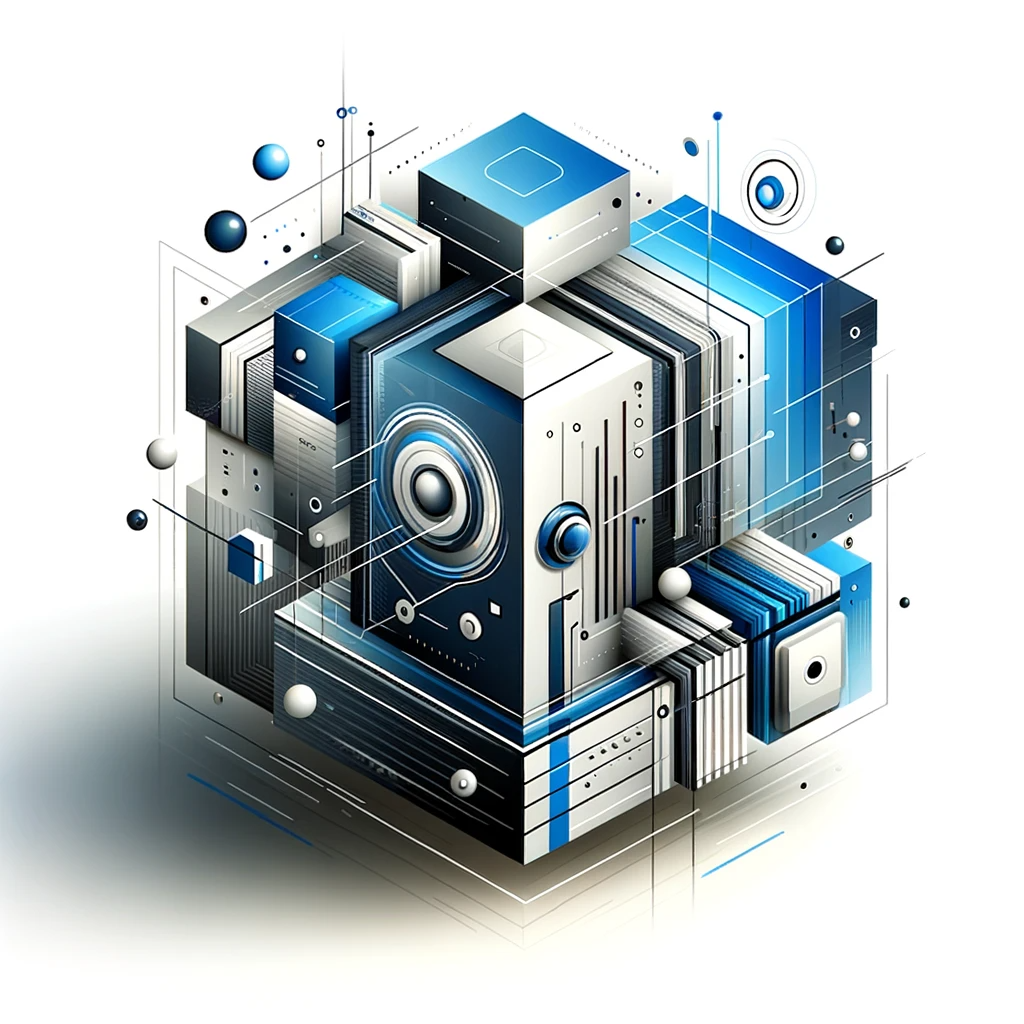
Abstract
Metagenome is a combination of several microorganisms collected from the environment. In metagenome analysis, it is required binning for grouping metagenome fragment yielded by sequencer. This research used the composition approach for conducting metagenome fragment binning. In this approach, binning could be implemented using unsupervised or supervised learning. We used Self-Organizing Map (SOM) for conducting binning used on unsupervised learning. We compared two techniques of training in SOM, namely sequential training and batch training for finding the best techniques. The results showed that the batch training could obtain 3.8% error valued on the map of [10 15]. This error value is smaller than that of sequential training. DOI: http://dx.doi.org/10.11591/telkomnika.v13i3.7115
Discover Our Library
Embark on a journey through our expansive collection of articles and let curiosity lead your path to innovation.
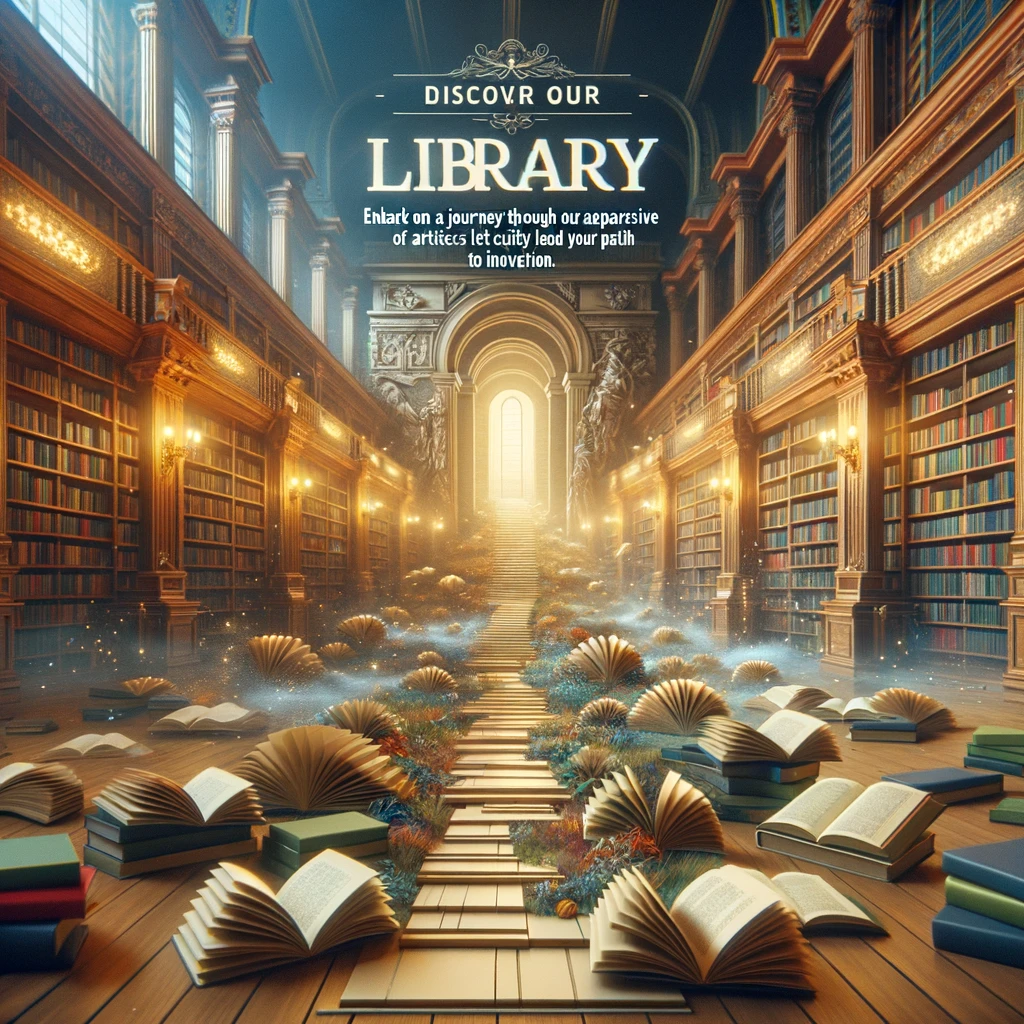