Sensitivity of Support Vector Machine Classification to Various Training Features
Indonesian Journal of Electrical Engineering and Computer Science
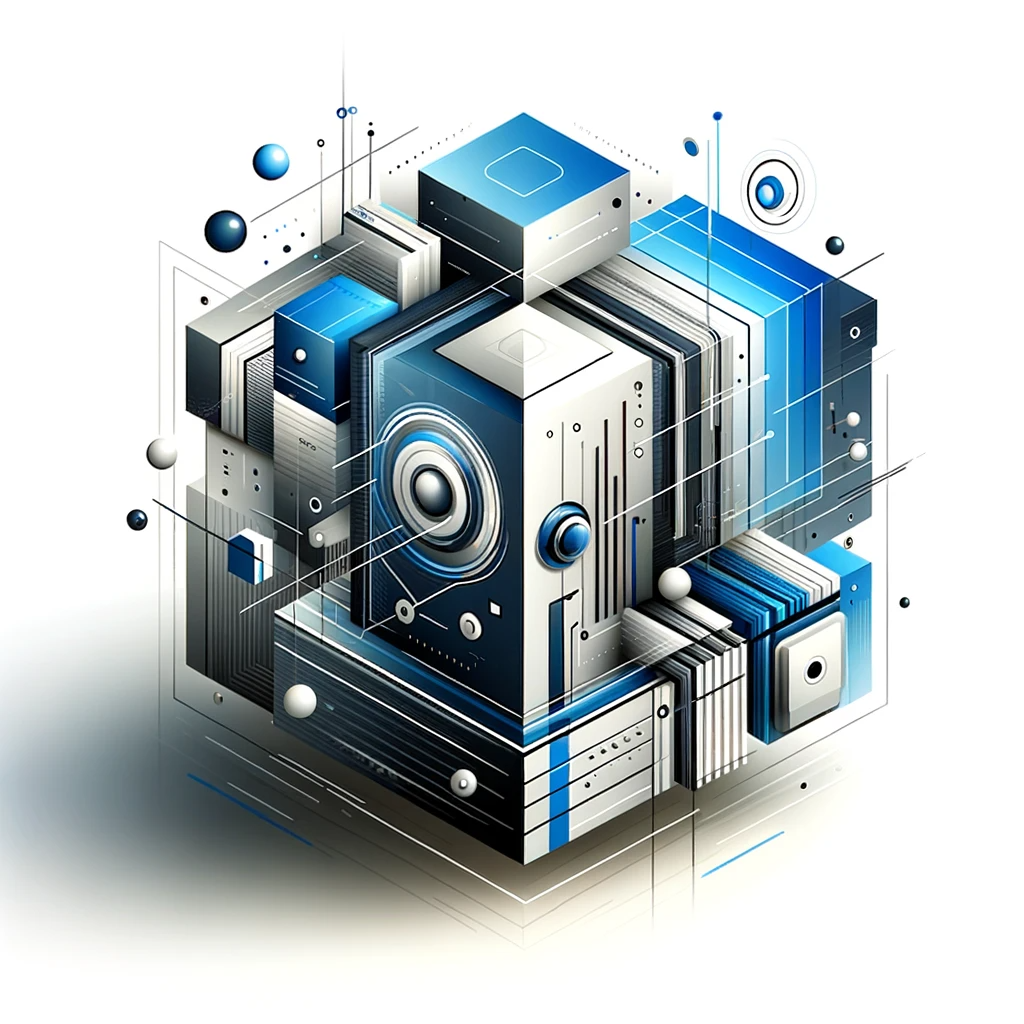
Abstract
Remote sensing image classification is one of the most important techniques in image interpretation, which can be used for environmental monitoring, evaluation and prediction. Many algorithms have been developed for image classification in the literature. Support vector machine (SVM) is a kind of supervised classification that has been widely used recently. The classification accuracy produced by SVM may show variation depending on the choice of training features. In this paper, SVM was used for land cover classification using Quickbird images. Spectral and textural features were extracted for the classification and the results were analyzed thoroughly. Results showed that the number of features employed in SVM was not the more the better. Different features are suitable for different type of land cover extraction. This study verifies the effectiveness and robustness of SVM in the classification of high spatial resolution remote sensing images. DOI : http://dx.doi.org/10.11591/telkomnika.v12i1.3969
Discover Our Library
Embark on a journey through our expansive collection of articles and let curiosity lead your path to innovation.
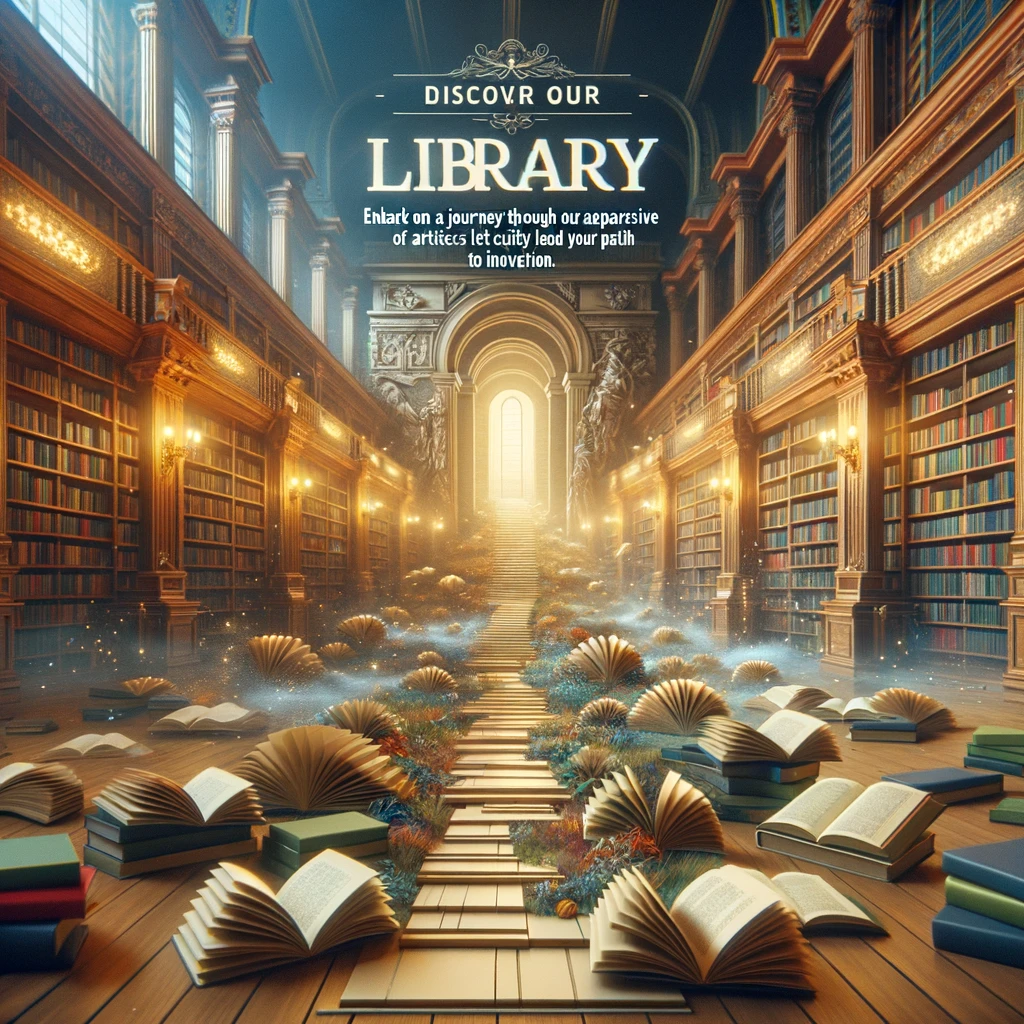