Wavelet Neural Network-based Short-Term Passenger Flow Forecasting on Urban Rail Transit
Indonesian Journal of Electrical Engineering and Computer Science
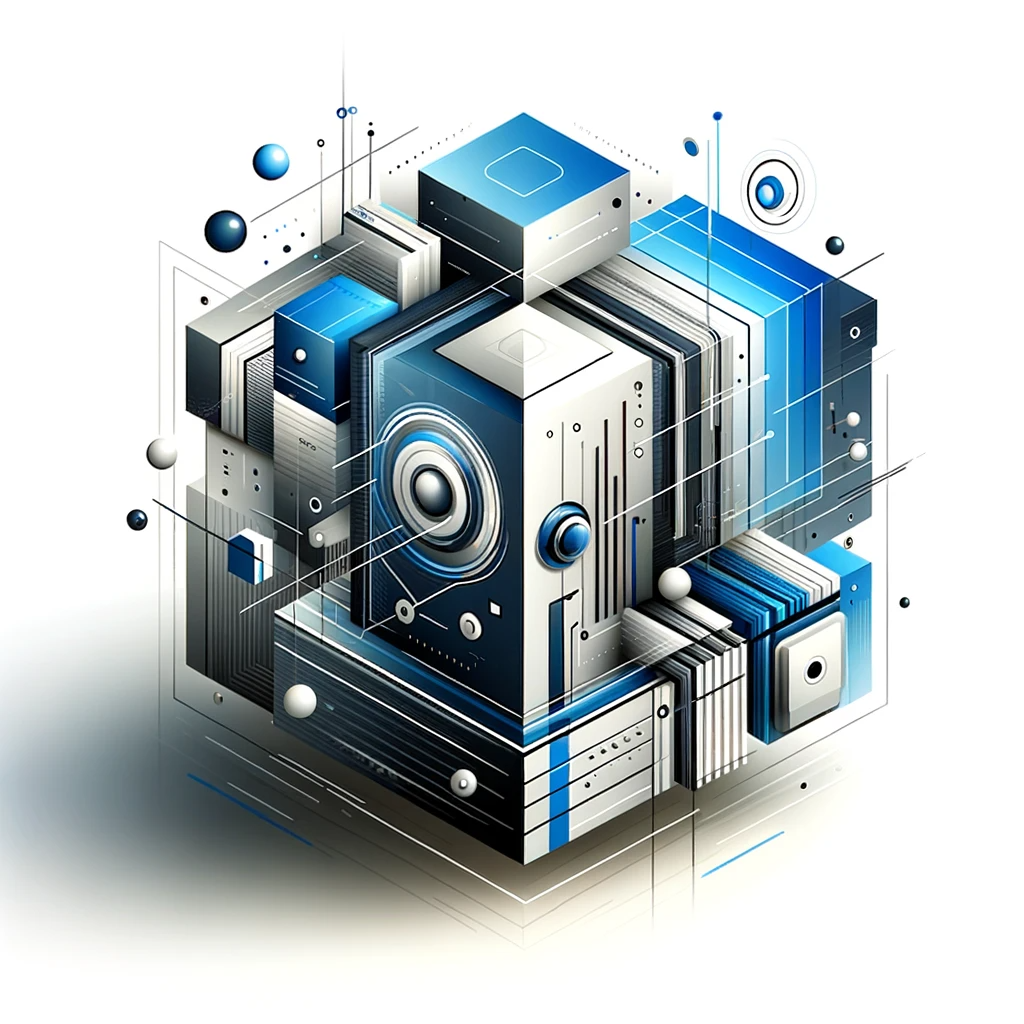
Abstract
Accurate forecasting of short-term passenger flow has been one of the most important issues in urban rail transit planning and operation. Considering the shortcomings of traditional forecasting methods, and in order to improve forecasting accuracy of passenger flow, this paper presents a wavelet neural network (WNN) for short-term passenger flow forecasting. One real urban rail transit station with large and significantly changed passenger flow is chosen to be the example. The proposed method and BP neural network have been compared with the results, which show that the WNN model has more advantages. The WNN model features higher learning speed and drastically less convergence time, showing that it is meaningful in practical application. Furthermore, the calculated relative errors using BP neural network are nearly in the range [-0.4, 0.3] and the calculated relative errors are in the range [-0.25, 0.1] using the WNN, which demonstrate the superior accuracy of using this approach. DOI: http://dx.doi.org/10.11591/telkomnika.v11i12.3096
Discover Our Library
Embark on a journey through our expansive collection of articles and let curiosity lead your path to innovation.
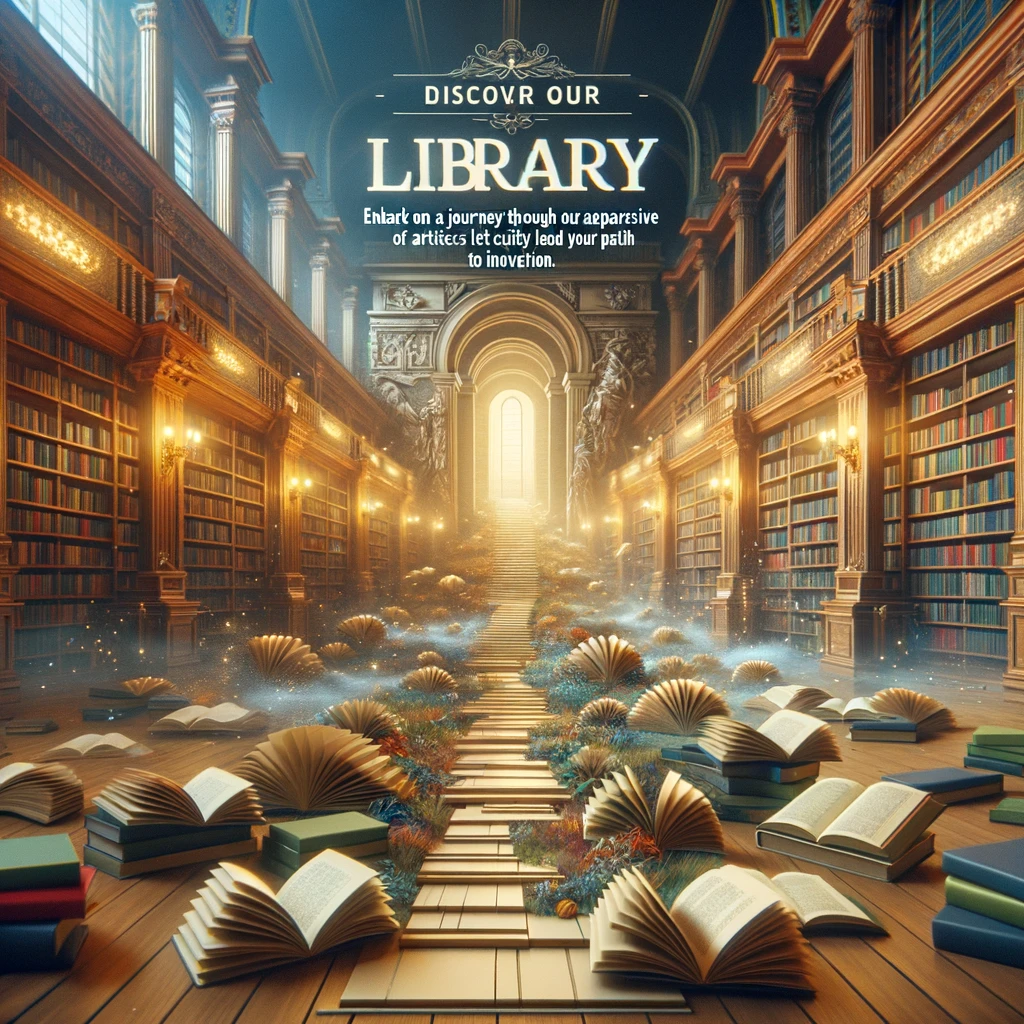