Precision medicine in hepatology: harnessing IoT and machine learning for personalized liver disease stage prediction
International Journal of Reconfigurable and Embedded Systems
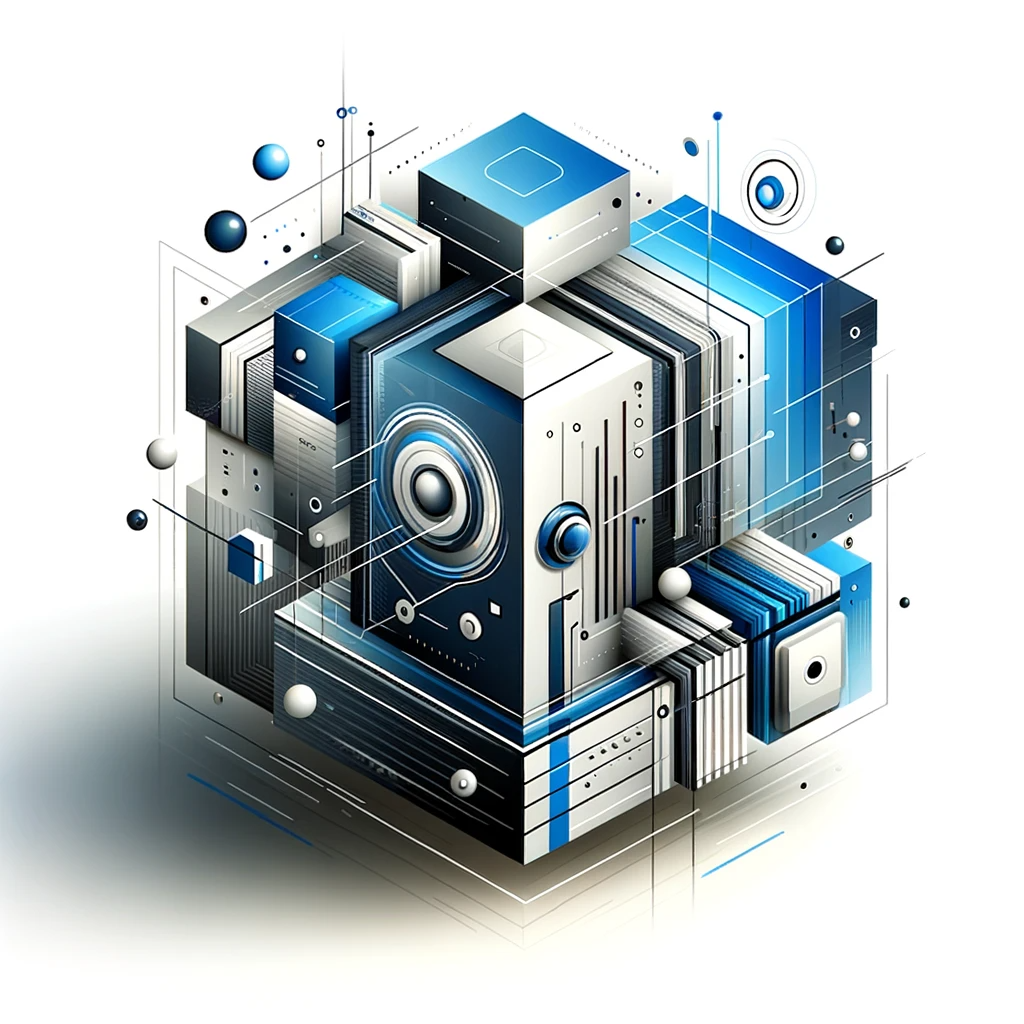
Abstract
In this research, we used a dataset from Siksha ‘O’ Anusandhan (S’O’A) University Medical Laboratory containing 6,780 samples collected manually and through internet of things (IoT) sensor sources from 6,780 patients to perform a thorough investigation into liver disease stage prediction. The dataset was carefully cleaned before being sent to the machine learning pipeline. We utilised a range of machine learning models, such as Naïve Bayes (NB), sequential minimal optimisation (SMO), K-STAR, random forest (RF), and multi-class classification (MCC), using Python to predict the stages of liver disease. The results of our simulations demonstrated how well the SMO model performed in comparison to other models. We then expanded our analysis using different machine learning boosting models with SMO as the base model: adaptive boosting (AdaBoost), gradient boost, extreme gradient boosting (XGBoost), CatBoost, and light gradient boosting model (LightGBM). Surprisingly, gradient boost proved to be the most successful, producing an astounding 96% accuracy. A closer look at the data showed that when AdaBoost was combined with the SMO base model, the accuracy results were 94.10%, XGBoost 90%, CatBoost 92%, and LightGBM 94%. These results highlight the effectiveness of proposed model i.e. gradient boosting in improving the prediction of liver disease stage and provide insightful information for improving clinical decision support systems in the field of medical diagnostics.
Discover Our Library
Embark on a journey through our expansive collection of articles and let curiosity lead your path to innovation.
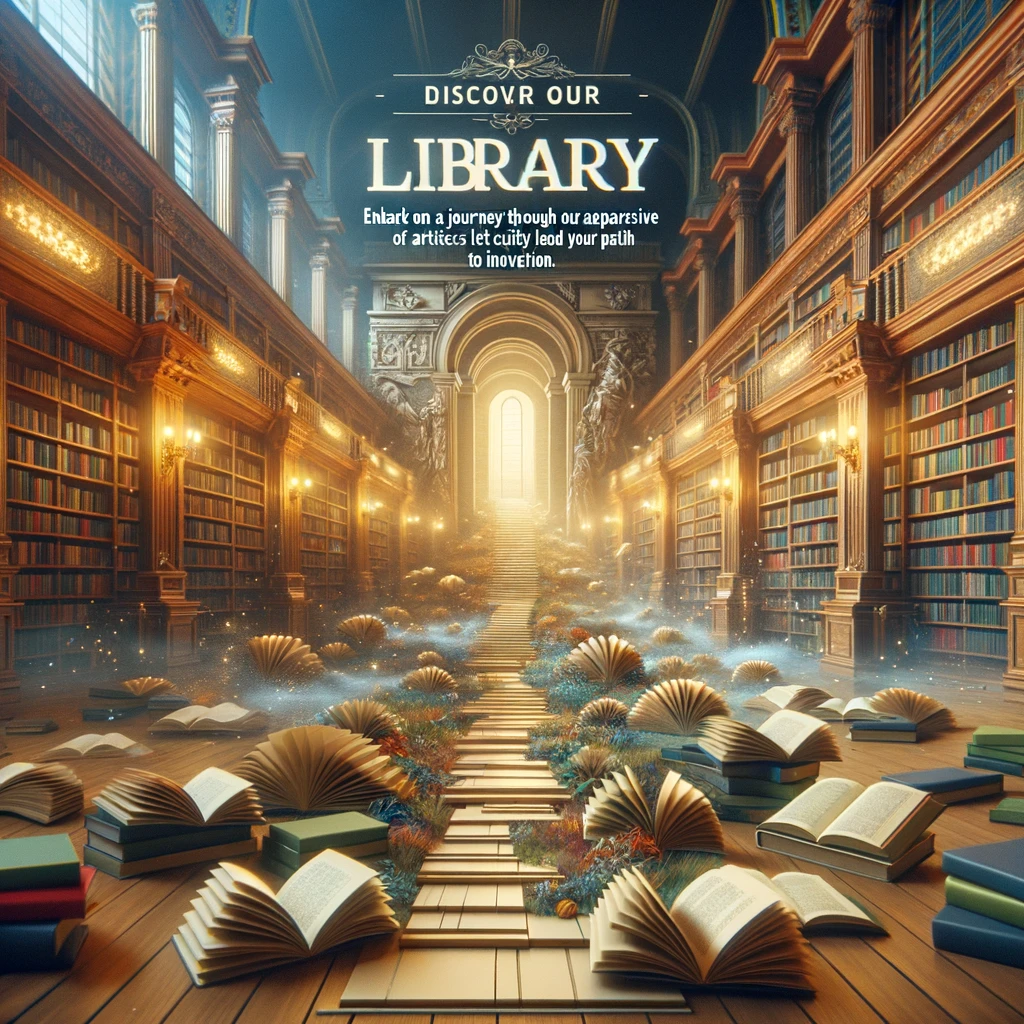