Process Improvement of LSA for Semantic Relatedness Computing
Telecommunication Computing Electronics and Control
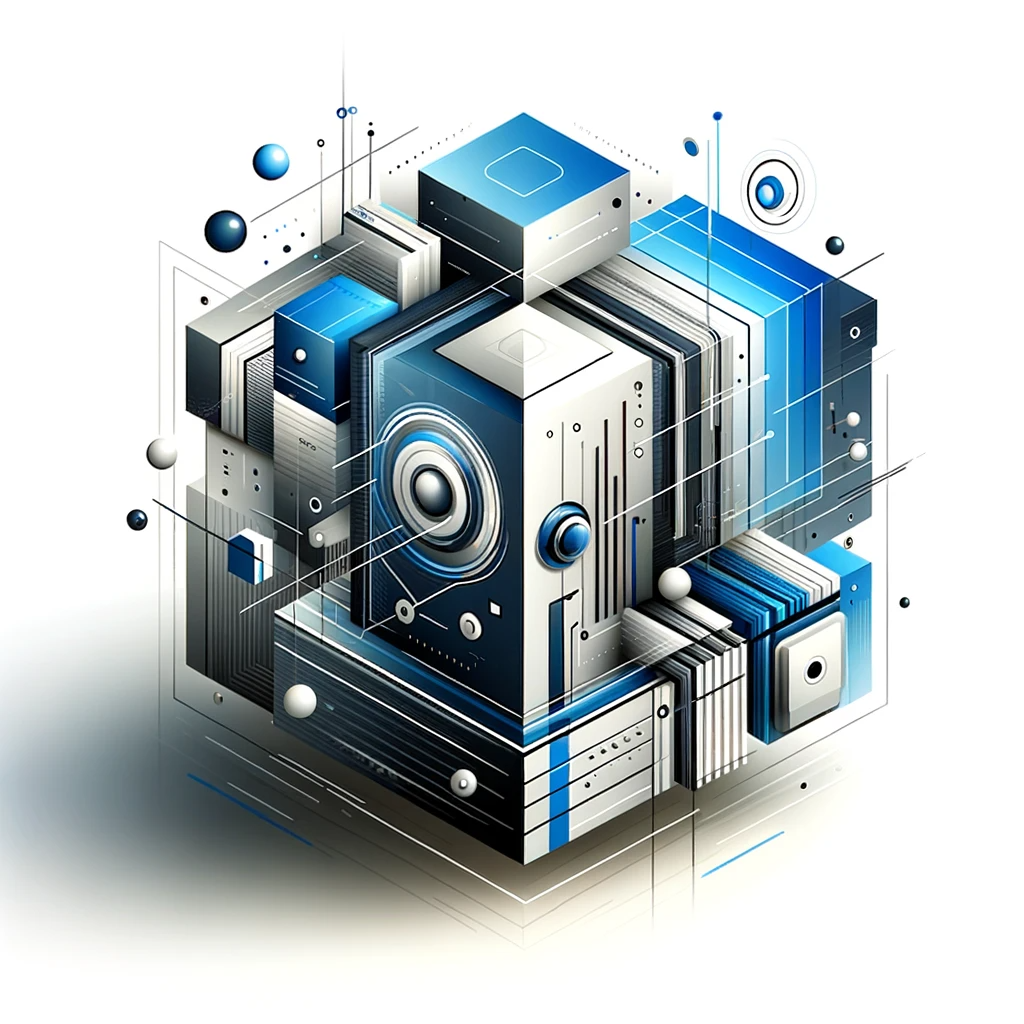
Abstract
Tang poetry semantic correlation computing is critical in many applications, such as searching, clustering, automatic generation of poetry and so on. Aiming to increase computing efficiency and accuracy of semantic relatedness, we improved the process of latent semantic analysis (LSA). In this paper, we adopted “representation of words semantic” instead of “words-by-poems” to represent the words semantic, which based on the finding that words having similar distribution in poetry categories are almost always semantically related. Meanwhile, we designed experiment which obtained segmentation words from more than 40000 poems, and computed relatedness by cosine value which calculated from decomposed co-occurrence matrix with Singular Value Decomposition (SVD) method. The experimental result shows that this method is good to analyze semantic and emotional relatedness of words in Tang poetry. We can find associated words and the relevance of poetry categories by matrix manipulation of the decomposing matrices as well.
Discover Our Library
Embark on a journey through our expansive collection of articles and let curiosity lead your path to innovation.
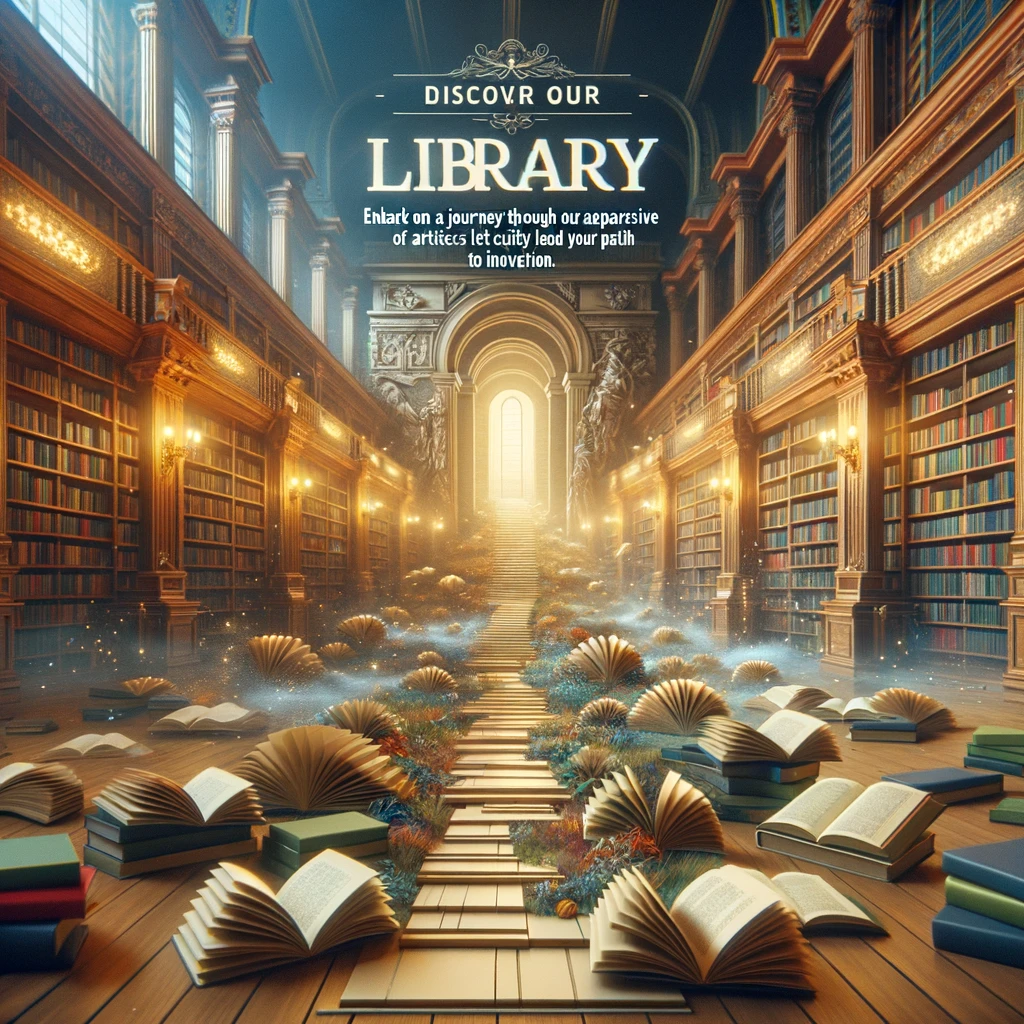