Machine learning-driven stock price prediction for enhanced investment strategy
International Journal of Electrical and Computer Engineering
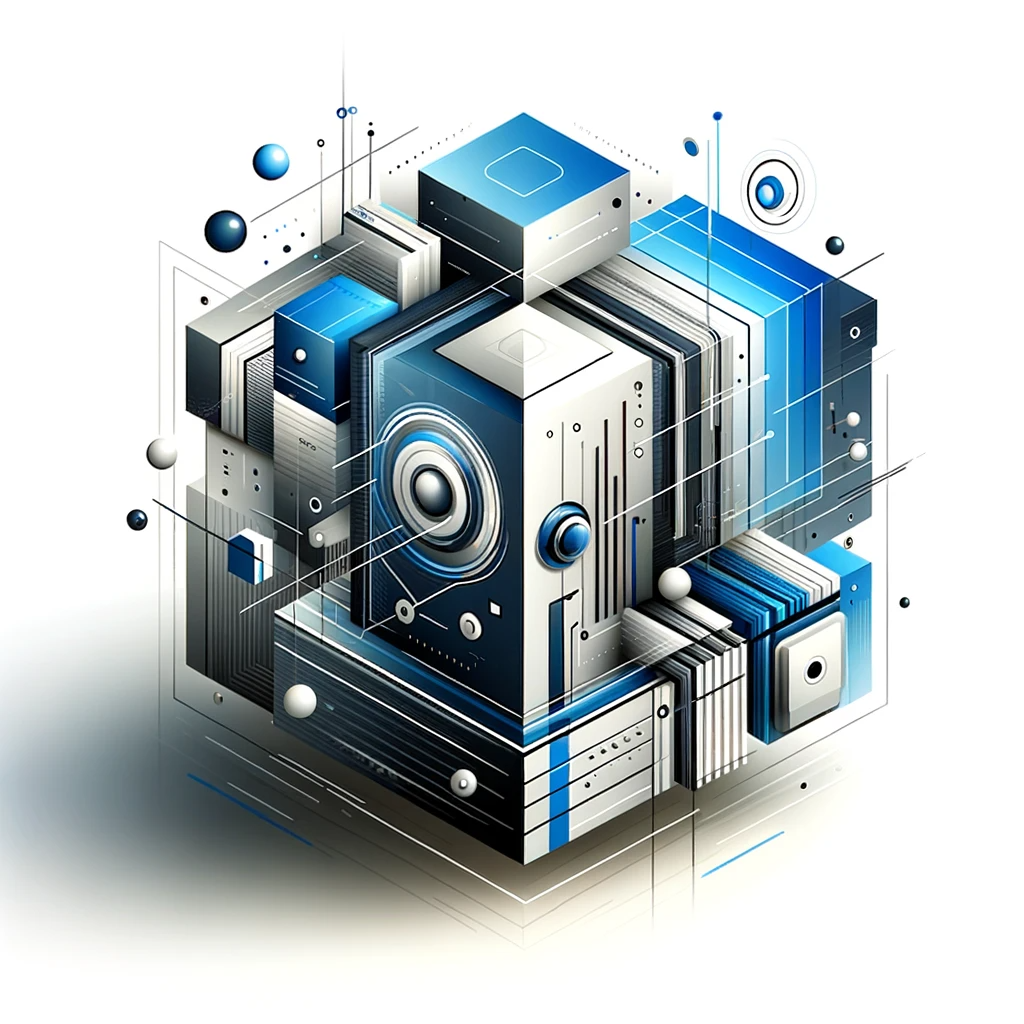
Abstract
Forecasting stock prices, a task complicated by the inherent volatility of the stock market, poses a significant challenge. The ability to accurately forecast stock prices is crucial, as it provides investors with crucial insights, enabling them to make informed strategic decisions. In this paper, we propose a novel investment strategy that relies on predicting stock prices. Our approach utilizes a hybrid predictive model that combines light gradient-boosting machine (LightGBM) and extreme gradient boosting (XGBoost). This model is designed to generate short to medium-term forecasts for a wide range of stocks. The strategy has shown promising results, surpassing the local market indices used as benchmarks in terms of both risk and return. Our findings demonstrate the strategy's effectiveness in both upward and downward market trends, underscoring its potential as a robust tool for portfolio management in diverse market conditions.
Discover Our Library
Embark on a journey through our expansive collection of articles and let curiosity lead your path to innovation.
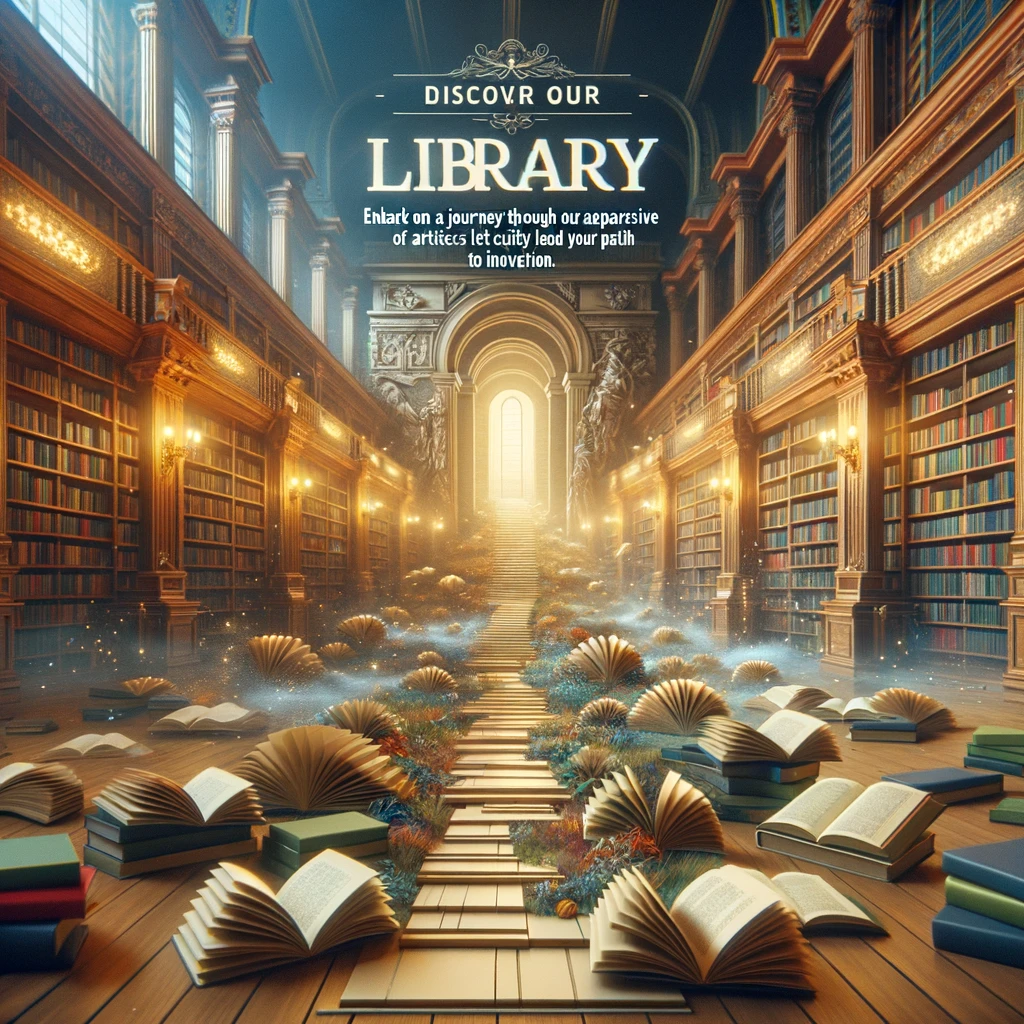