Optimizing intrusion detection in 5G networks using dimensionality reduction techniques
International Journal of Electrical and Computer Engineering
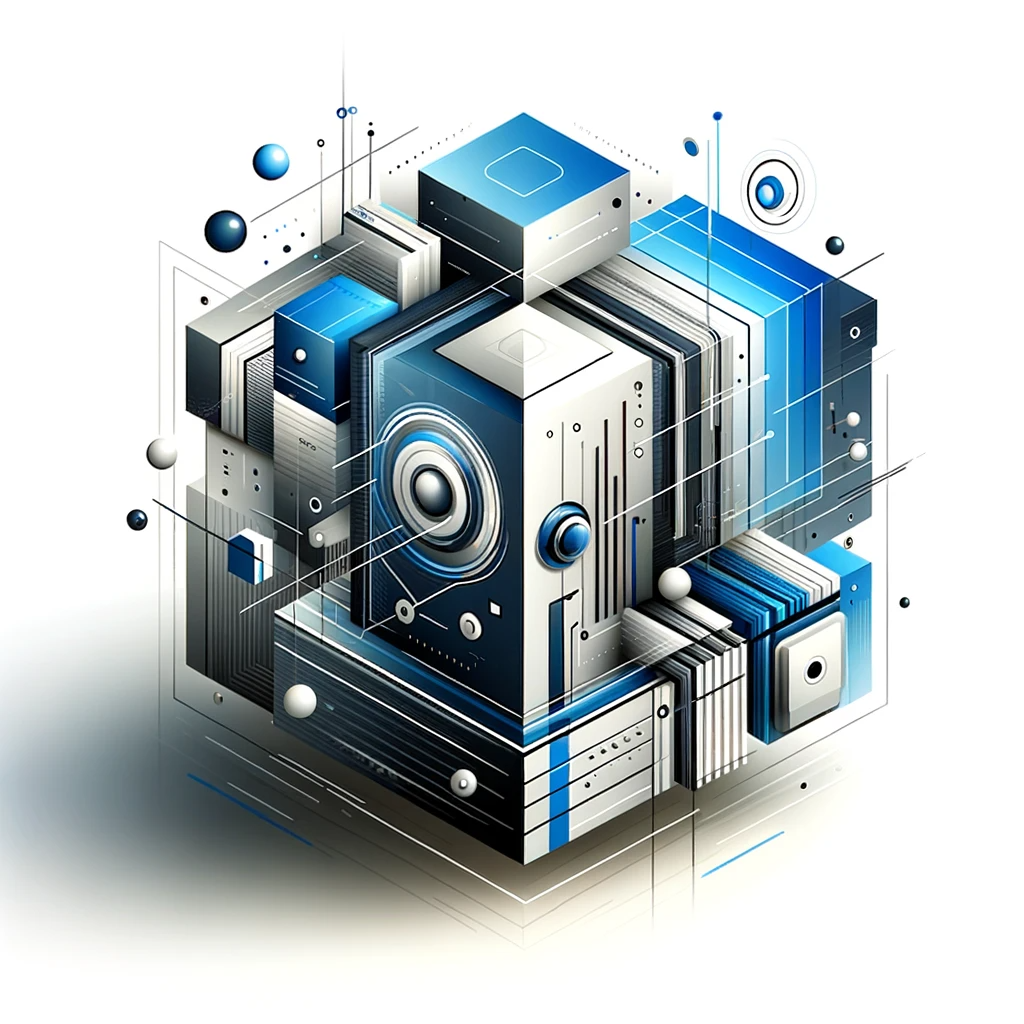
Abstract
The proliferation of internet of things (IoT) technologies has expanded the user base of the internet, but it has also exposed users to increased cyber threats. Intrusion detection systems (IDSs) play a vital role in safeguarding against cybercrimes by enabling early threat response. This research uniquely centers on the critical dimensionality aspects of wireless datasets. This study focuses on the intricate interplay between feature dimensionality and intrusion detection systems. We rely on the renowned IEEE 802.11 security-oriented AWID3 dataset to implement our experiments since AWID was the first dataset created from wireless network traffic and has been developed into AWID3 by capturing and studying traces of a wide variety of attacks sent into the IEEE 802.1X extensible authentication protocol (EAP) environment. This research unfolds in three distinct phases, each strategically designed to enhance the efficacy of our framework, using multi-nominal class, multi-numeric class, and binary class. The best accuracy achieved was 99% in the three phases, while the lowest accuracy was 89.1%, 60%, and 86.7% for the three phases consecutively. These results offer a comprehensive understanding of the intricate relationship between wireless dataset dimensionality and intrusion detection effectiveness.
Discover Our Library
Embark on a journey through our expansive collection of articles and let curiosity lead your path to innovation.
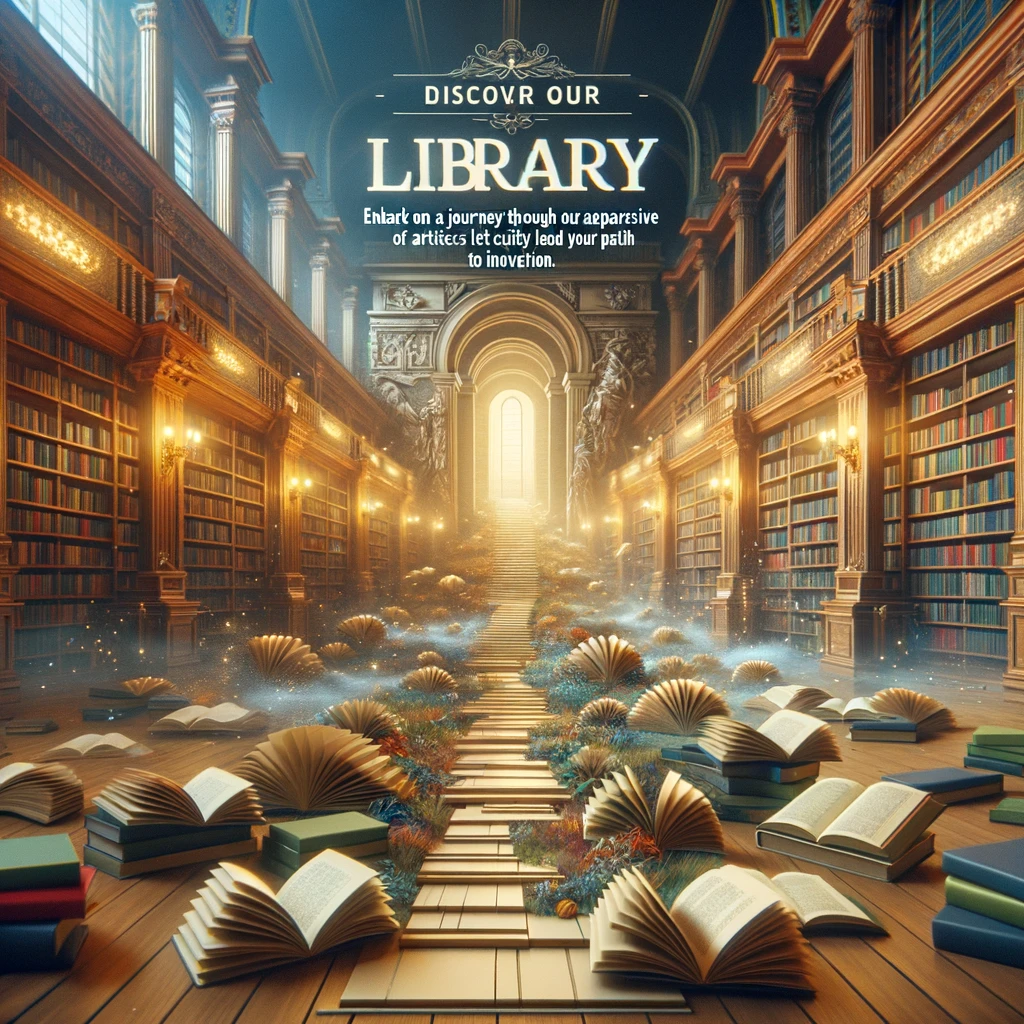