Detection of elements of personal safety for the prevention of accidents at work with convolutional neural networks
International Journal of Electrical and Computer Engineering
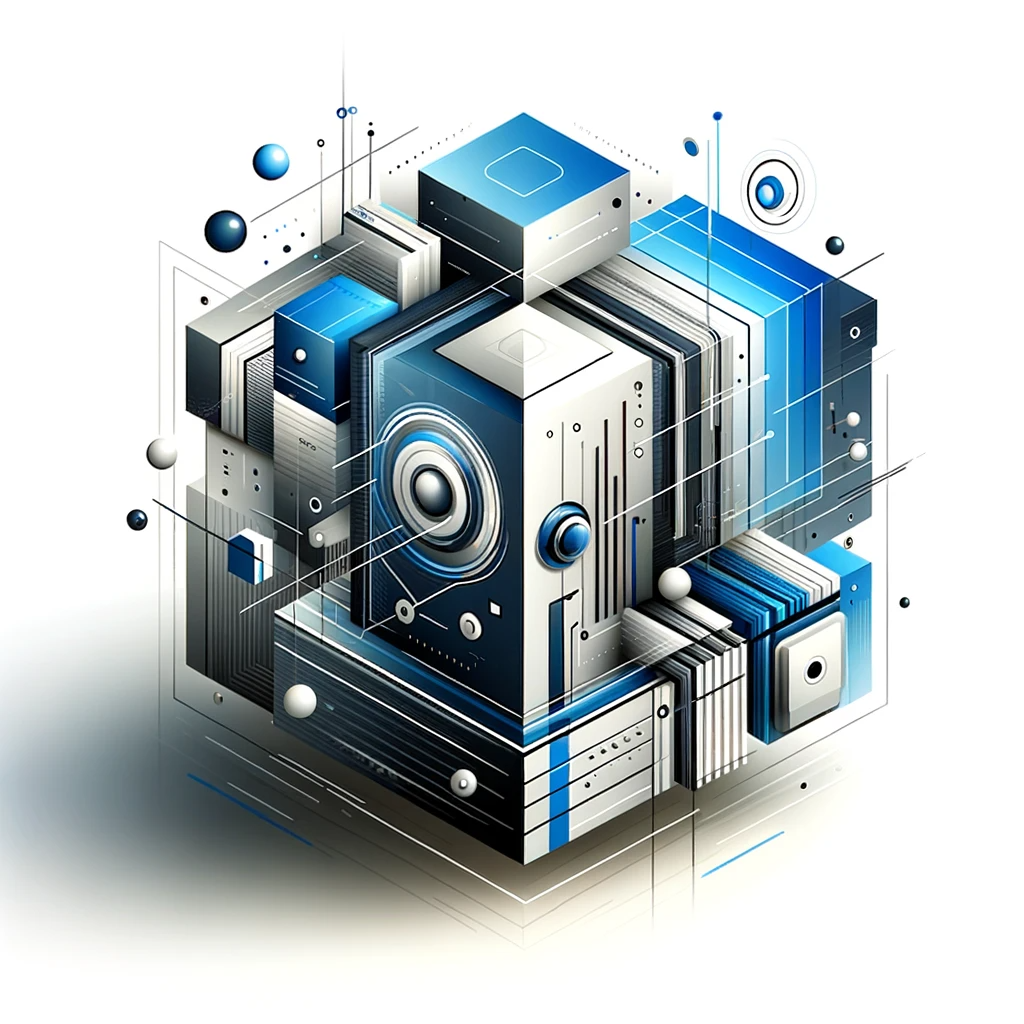
Abstract
The task of recognizing personal protective elements in workplace environments in real time is fundamental to protecting the employees in case of any accidents. This can be achieved by deploying a convolutional neural network (CNN) algorithm that can efficiently detect protective elements through surveillance devices. Therefore, this work proposes the construction of a model, implementing the you only look once (YOLO) detector, whose architecture has been one of the most tested according to literature review. YOLOv5 and YOLOv7 versions were used and a dataset of 2,000 images for four classes considered. This dataset was collection from various sources and labelled by the authors, of which 80% was used for training, 15% for testing and 5% for model validation. The most important metrics are presented, making a comparison between the models, and finally it was identified that YOLOv7 achieved a higher success rate, which could be considered a more complete solution for occupational health and safety management in companies.
Discover Our Library
Embark on a journey through our expansive collection of articles and let curiosity lead your path to innovation.
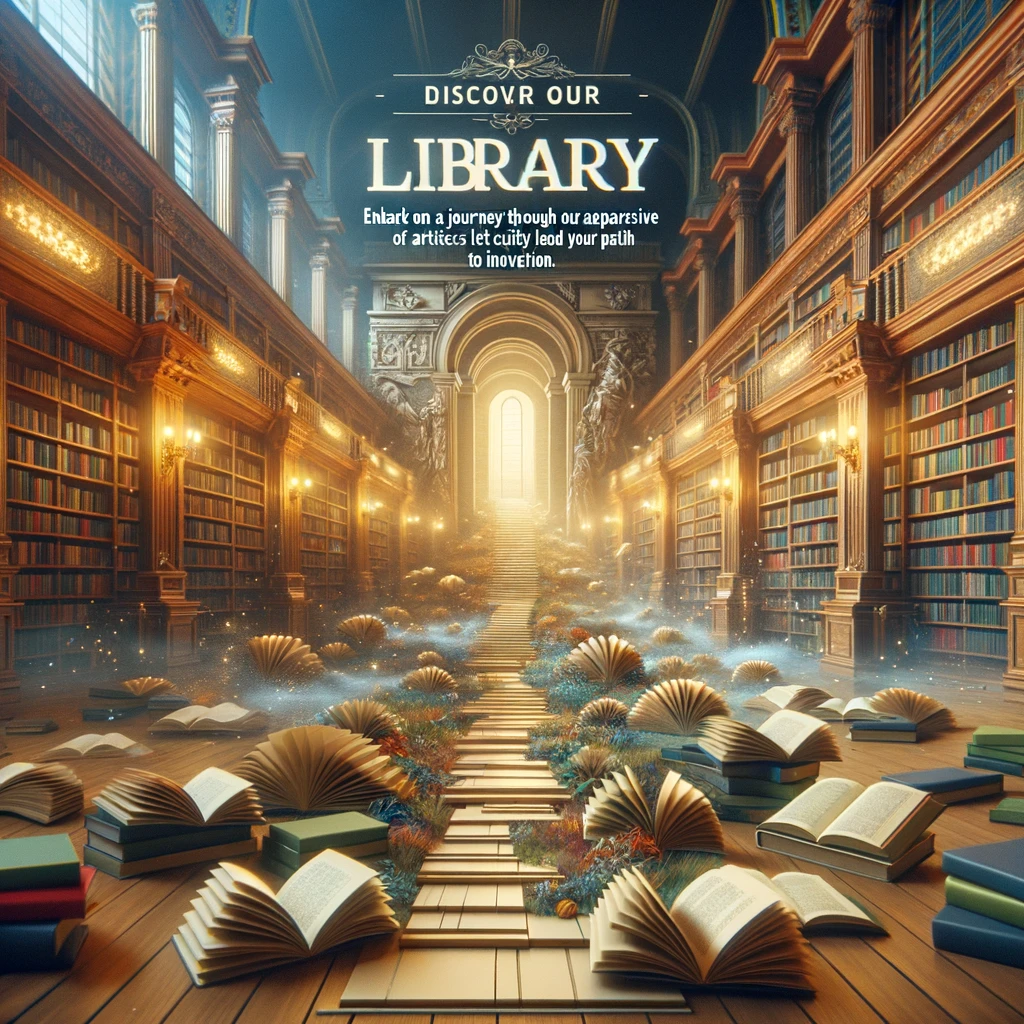