Enhancing stress detection in wearable IoT devices using federated learning and LSTM based hybrid model
Indonesian Journal of Electrical Engineering and Computer Science
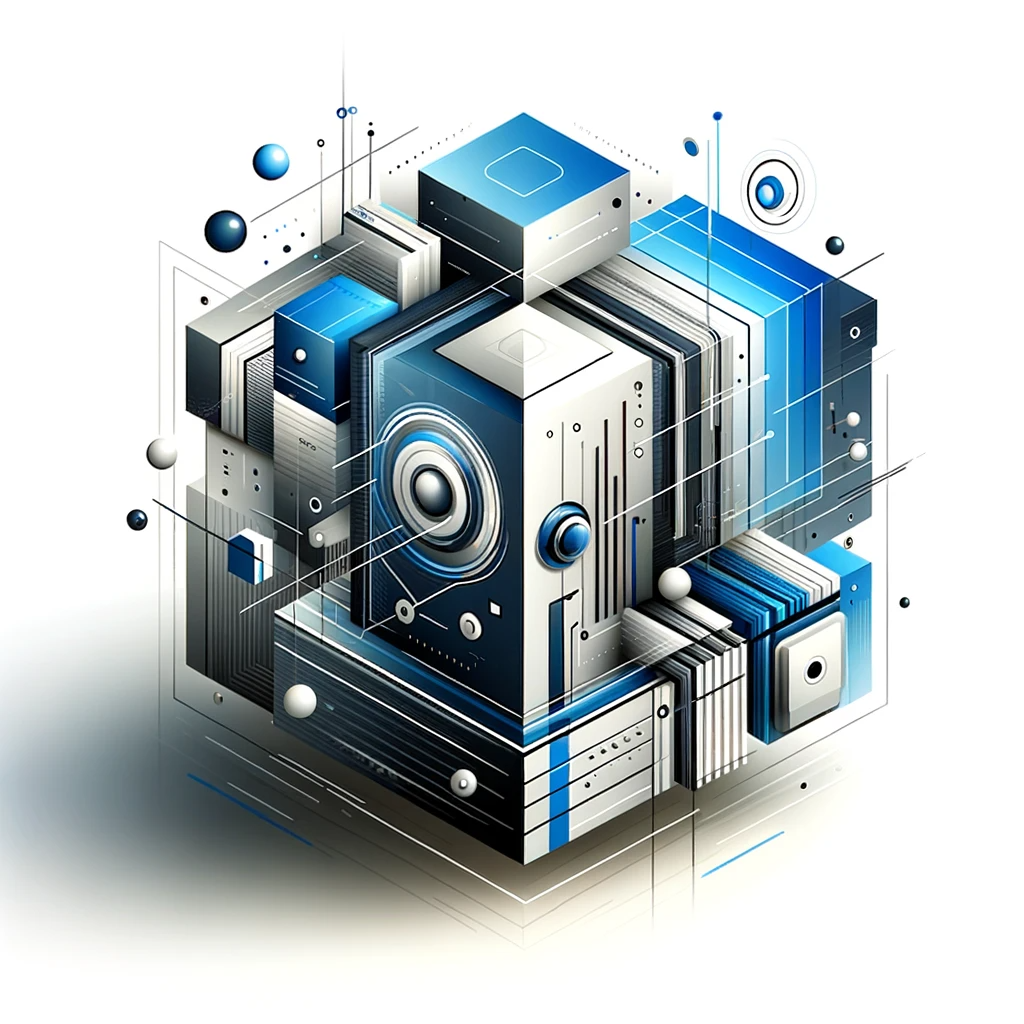
Abstract
In the domain of smart health devices, the accurate detection of physical indicators levels plays a crucial role in enhancing safety and well-being. This paper introduces a cross device federated learning framework using hybrid deep learning model. Specifically, the paper presents a comprehensive comparison of different combination of long short-term memory (LSTM), gated recurrent unit (GRU), convolutional neural network (CNN), random forest (RF), and extreme gradient boosting (XGBoost), in order to forecast stress levels by utilizing time series information derived from wearable smart gadgets. The LSTM-RF model demonstrated the highest level of accuracy, achieving 93.53% for user 1, 99.40% for user 2, and 97.88% for user 3. Similarly, the LSTM-XGBoost model yielded favorable outcomes, with accuracy rates of 85.88%, 98.55%, and 92.02% for users 1, 2, and 3, respectively. These findings highlight the efficacy of federated learning and the utilization of hybrid models in stress detection. Unlike traditional centralized learning paradigms, the presented federated approach ensures privacy preservation and reduces data transmission requirements by processing data locally on Edge devices.
Discover Our Library
Embark on a journey through our expansive collection of articles and let curiosity lead your path to innovation.
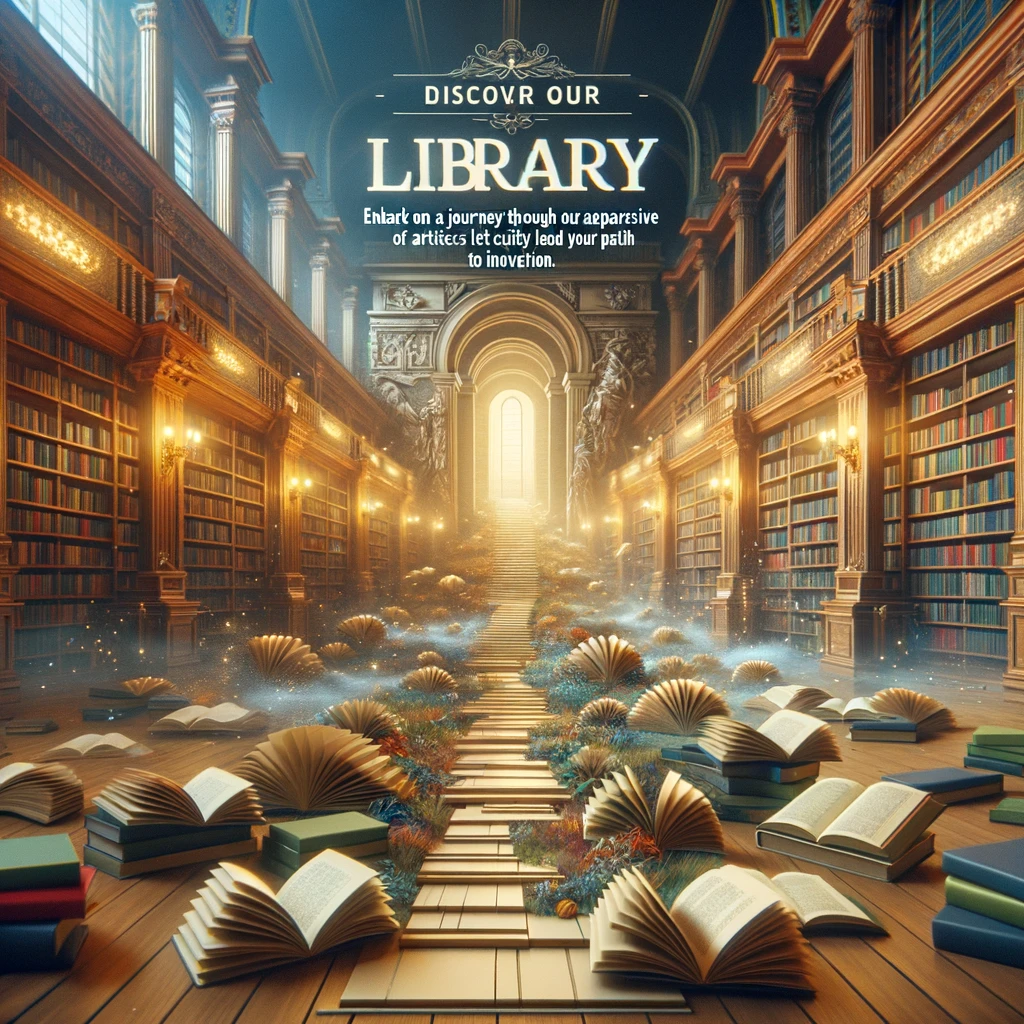