Mutual information-MOORA based feature weighting on naive bayes classifier for stunting data
Indonesian Journal of Electrical Engineering and Computer Science
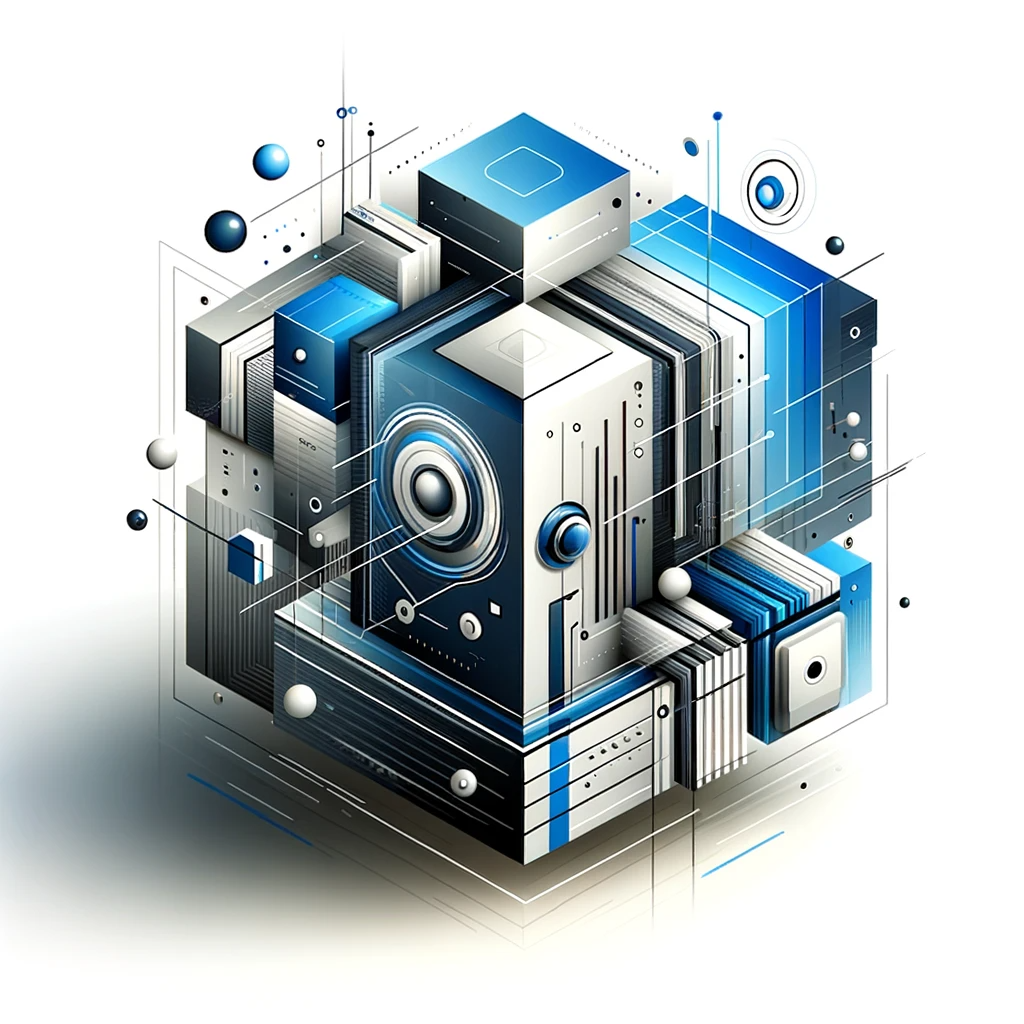
Abstract
One effort to reduce stunting rates is to predict stunting status early in toddlers. This study applies Naive Bayes (NB) to build a stunting prediction model because it is simple and easy to use. This study proposes a filter-based feature weighting technique to overcome the NB assumption, which states that each feature has the same contribution to the target. The frequency of an event in a dataset influences the feature weighting using mutual information criteria. This is the gap in the filter-based ranking highlighted in this study. Therefore, this study proposes a feature-weighting method that combines mutual information with the MOORA (MI-MOORA) decision-making method. This technique makes it possible to include external factors as criteria for ranking important features. For stunting cases, the external consideration for ranking purposes is the assessment of nutrition experts based on their experience in dealing with stunted toddlers. The MI-MOORA technique makes the availability of clean water the most influential feature that contributes to the stunting status. In the ten best features, the MI-MOORA ranking results are dominated by family factors. Based on the performance evaluation results of NB and other classifiers, MI-MOORA can improve the performance of stunt prediction models.
Discover Our Library
Embark on a journey through our expansive collection of articles and let curiosity lead your path to innovation.
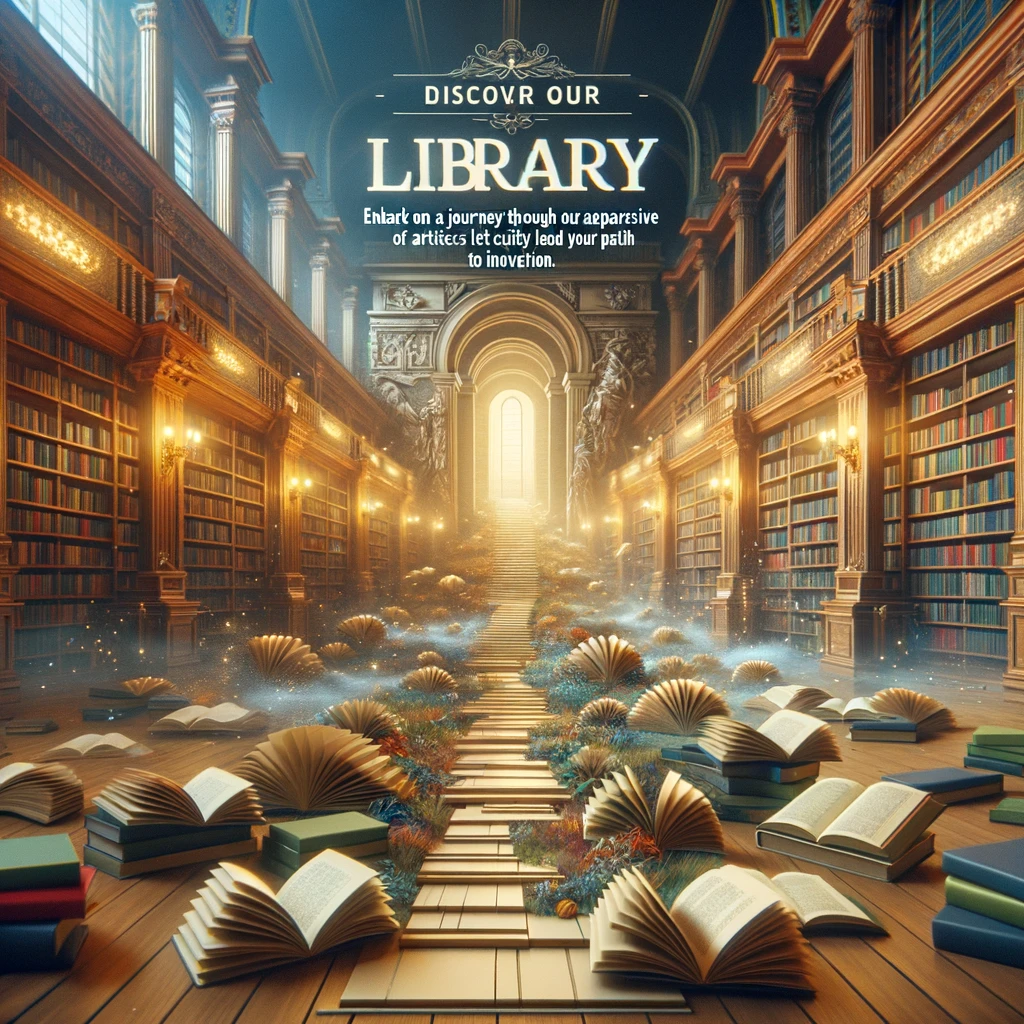