Handling class imbalance in education using data-level and deep learning methods
International Journal of Electrical and Computer Engineering
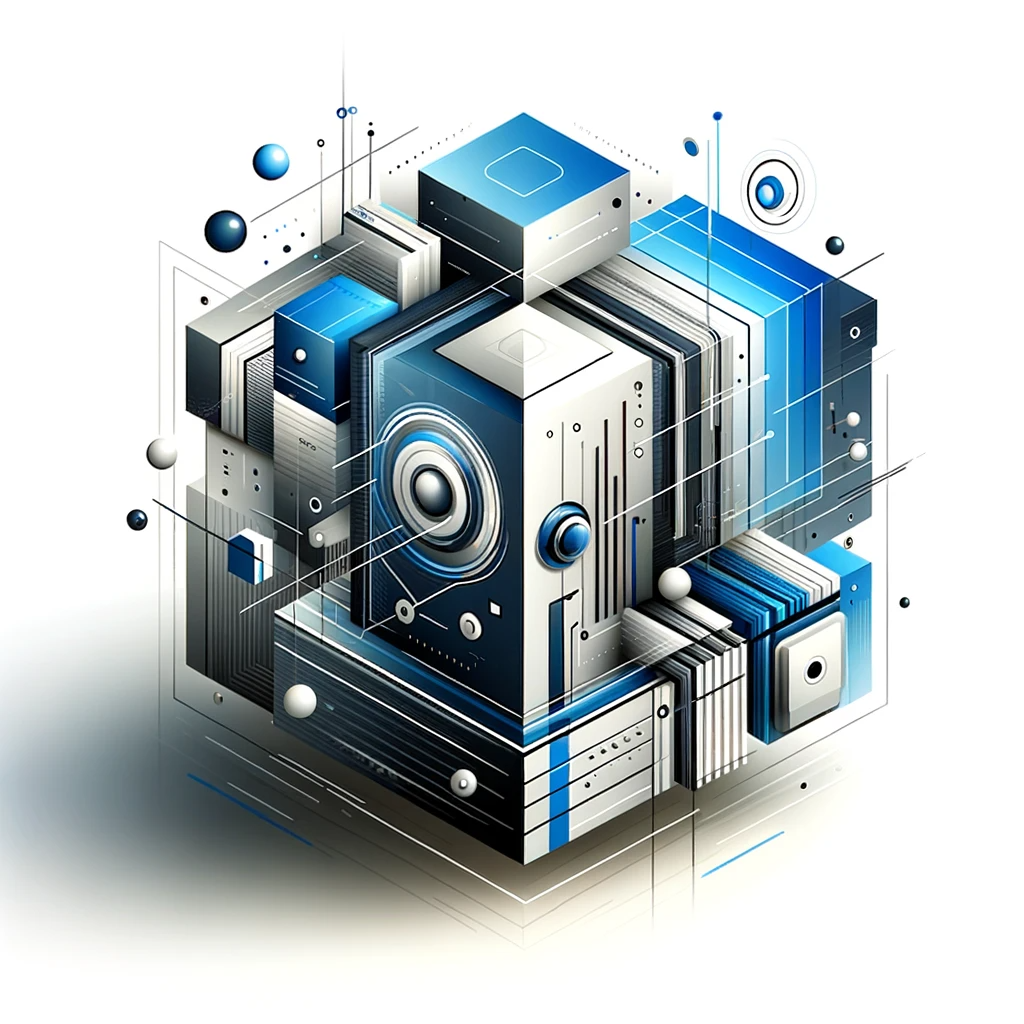
Abstract
In the current field of education, universities must be highly competitive to thrive and grow. Education data mining has helped universities in bringing in new students and retaining old ones. However, there is a major issue in this task, which is the class imbalance between the successful students and at-risk students that causes inaccurate predictions. To address this issue, 12 methods from data-level sampling techniques and 2 methods from deep learning synthesizers were compared against each other and an ideal class balancing method for the dataset was identified. The evaluation was done using the light gradient boosting machine ensemble model, and the metrics included receiver operating characteristic curve, precision, recall and F1 score. The two best methods were Tomek links and neighbourhood cleaning rule from undersampling technique with a F1 score of 0.72 and 0.71 respectively. The results of this paper identified the best class balancing method between the two approaches and identified the limitations of the deep learning approach.
Discover Our Library
Embark on a journey through our expansive collection of articles and let curiosity lead your path to innovation.
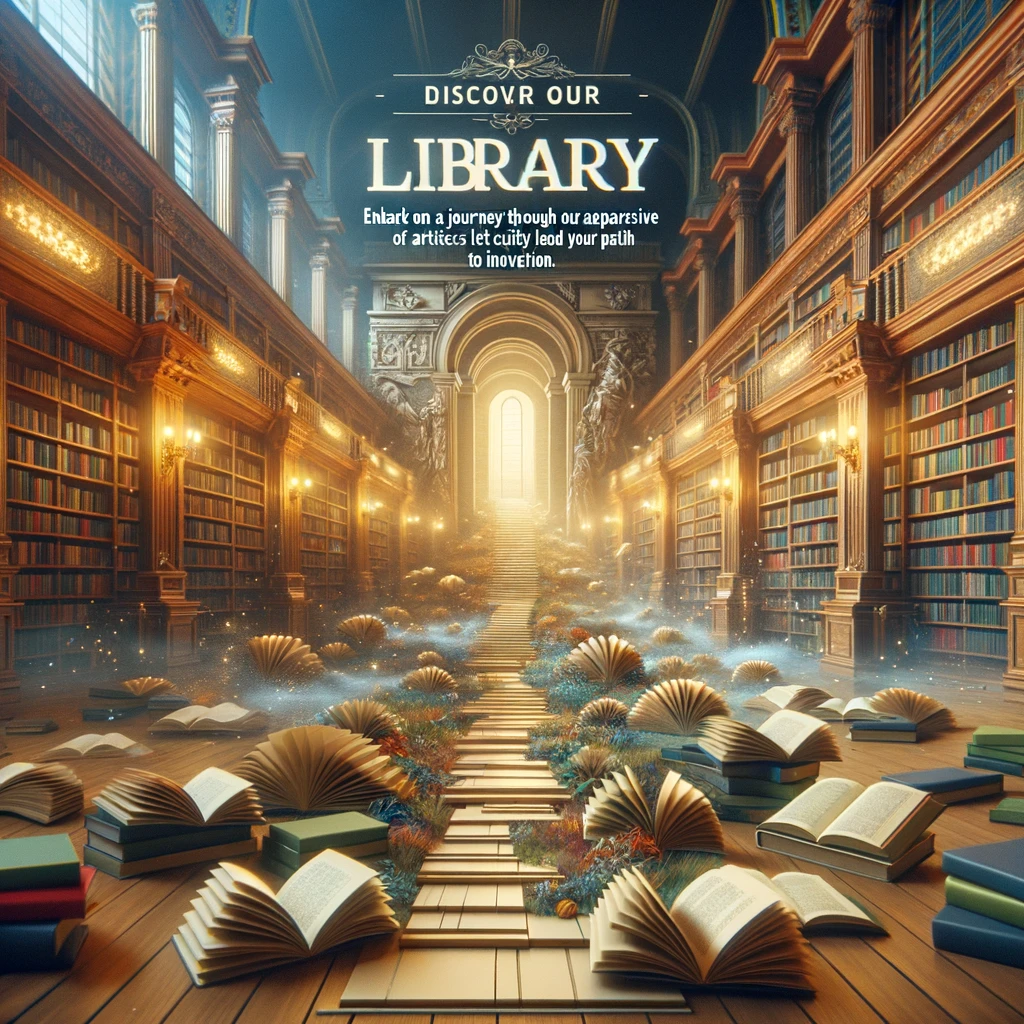