Airport infrastructure and runway precision aids for forecasting flight arrival delays
International Journal of Electrical and Computer Engineering
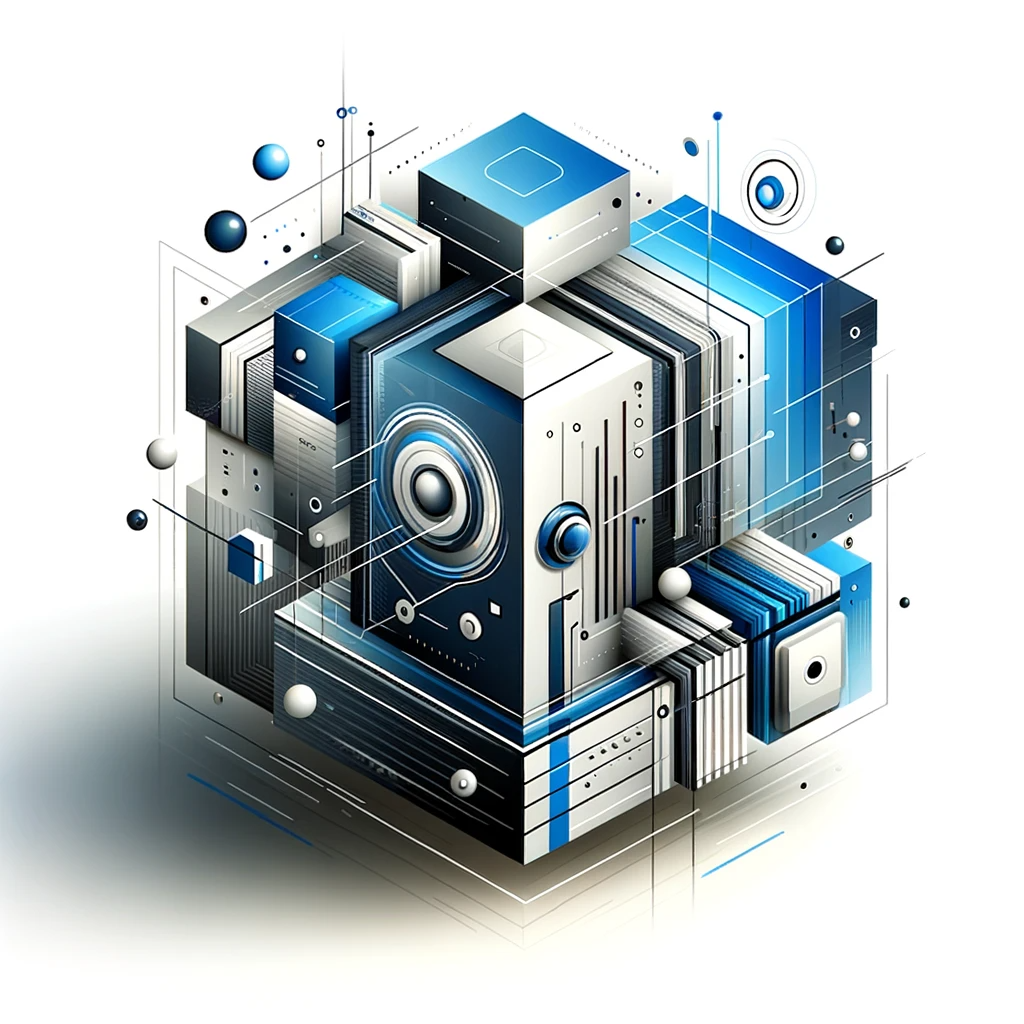
Abstract
Recent research has concentrated on using machine learning approaches to forecast flight delays. The majority of prior prediction algorithms were based on simple and standard attributes collected from the database from which the data were pulled. This article is the first attempt to propose novel features linked to airport capacity and infrastructure. The total runways, the total runway intersections, the longest runway length, the shortest runway length, the runway precision rate, the total terminals, and the total gates were all examined. In this paper, we suggest an optimized multilayer perceptron to predict flight arrival retards implementing data for domestic flights operated in United States airports. We employed data normalization, sampling techniques, and hyper-parameter tuning to strengthen the reliability of the suggested model. The experimental findings demonstrated that data normalization, sampling approaches, and Bayesian optimization produced the most accurate model with 92.49% accuracy. The achievements of the study were compared to other benchmark research from literature. The time complexity for the proposed model was computed and presented at the end of the investigation.
Discover Our Library
Embark on a journey through our expansive collection of articles and let curiosity lead your path to innovation.
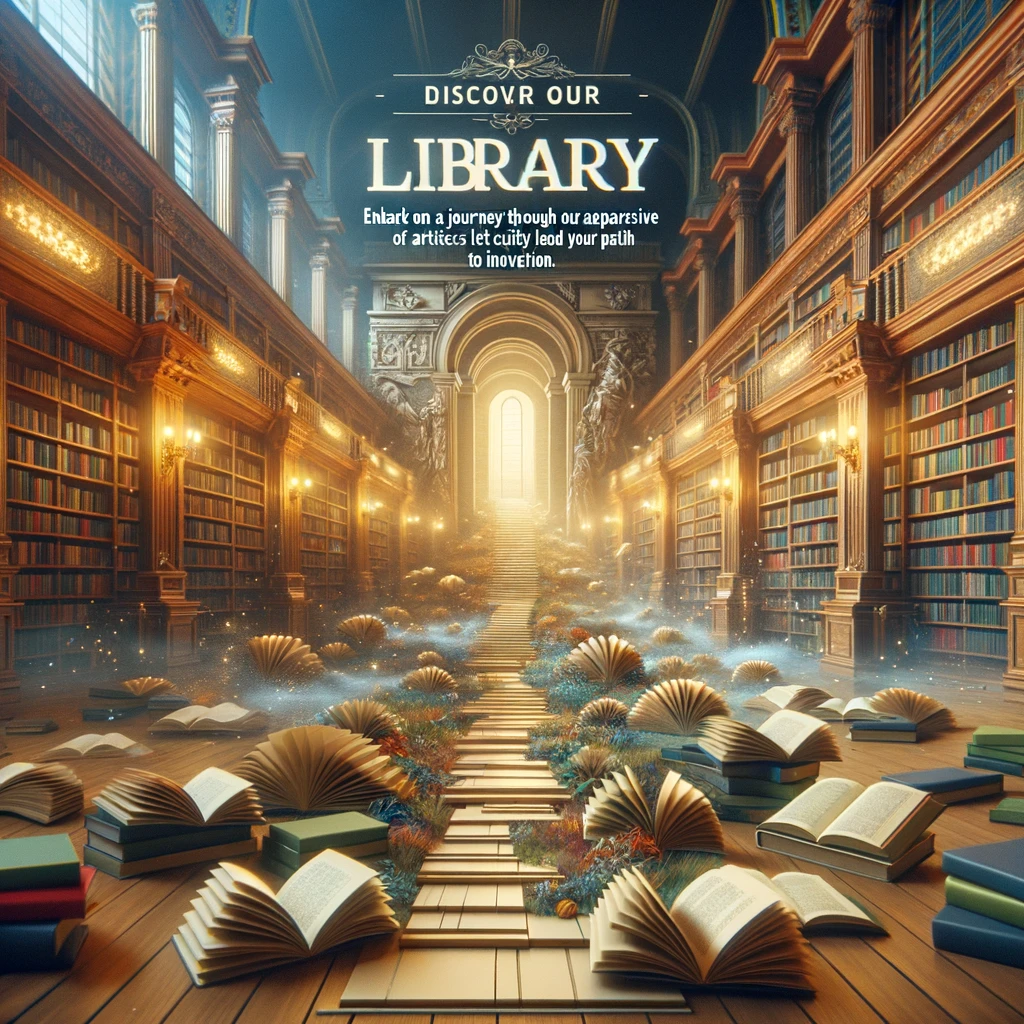