Denigration analysis of Twitter data using cyclic learning rate based long short-term memory
International Journal of Electrical and Computer Engineering
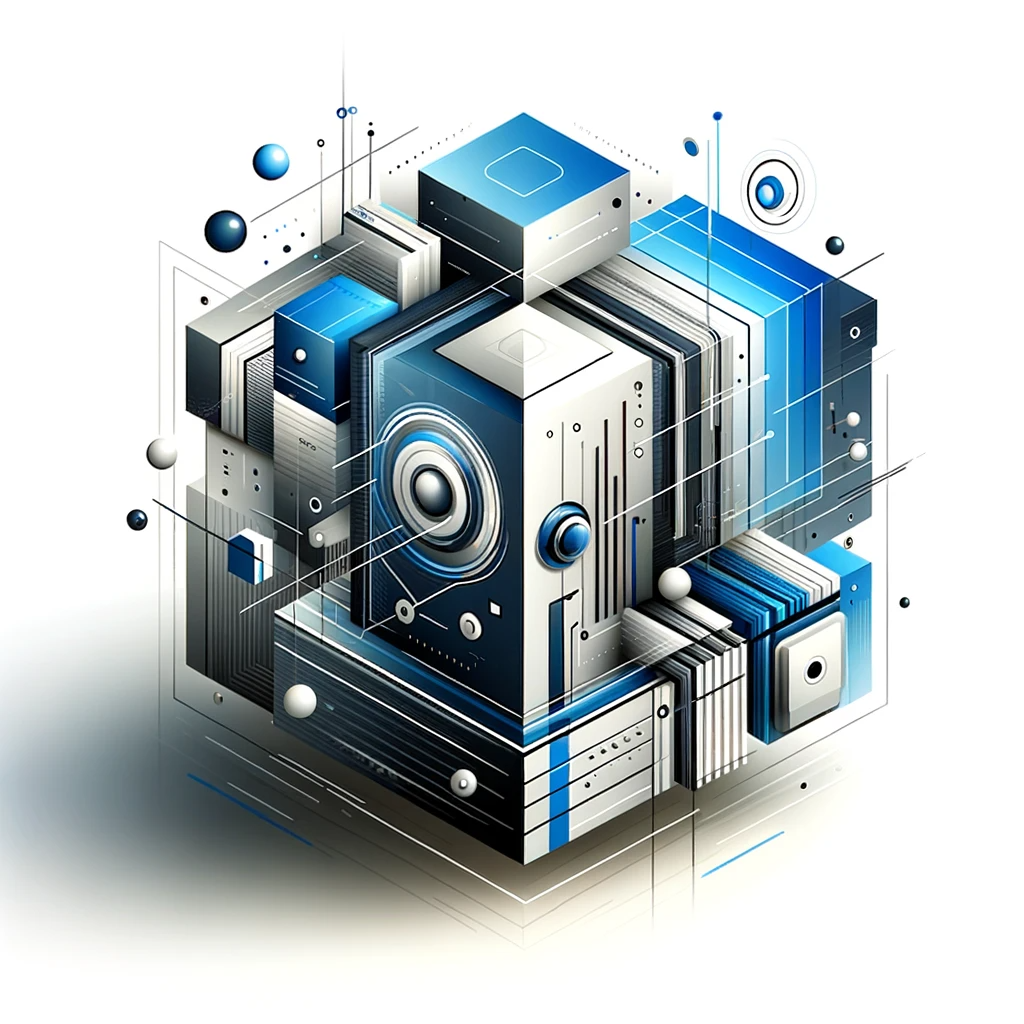
Abstract
Technological innovation has given rise to a new form of bullying, often leading to significant harm to one's reputation within social circles. When a single person becomes target to animosity and harassment in a cyberbullying incident, it is termed as denigration. Many different cyberbullying detection techniques are carried out to counter this, concentrating on word-based data and user account features only. The main objective of this research is to enhance the learning rate of long short-term memory (LSTM) using cyclic learning rate (CLR). Therefore, in this research, cyberbullying in social media is detected by developing a framework based on LSTM-CLR which is more stable for enhancing classification accuracy without the need for multiple trials and modifications. The effectiveness of the suggested LSTM-CLR is assessed for identifying cyberbullying using Twitter data. The attained results show that the proposed LSTM-CLR obtains 82% accuracy, 80% precision, 83% recall and 81% F-measure in the classification of cyberbullying tweets, which is superior when compared with the existing multilayer perceptron (MLP) and bidirectional encoder representations from transformers (BERT) models.
Discover Our Library
Embark on a journey through our expansive collection of articles and let curiosity lead your path to innovation.
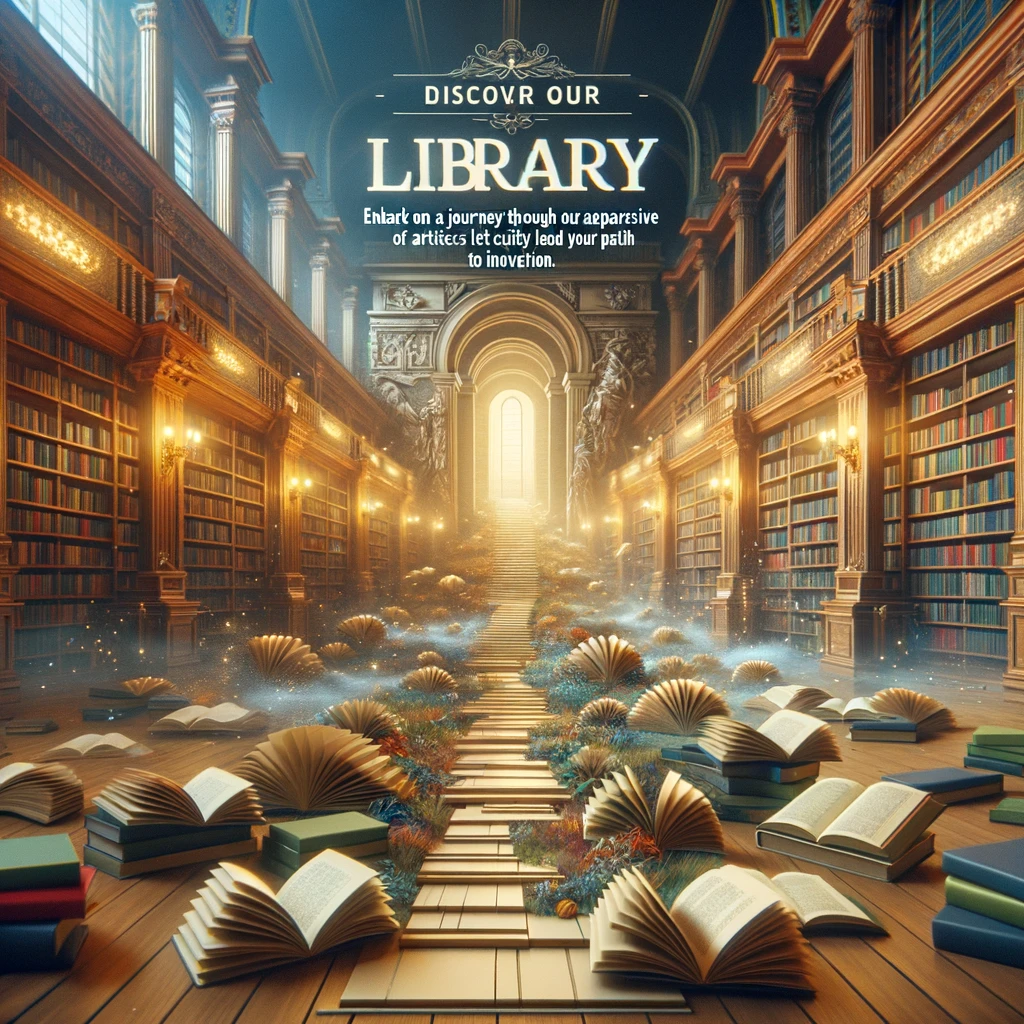