Berkeley wavelet transform and improved YOLOv7-based classification technique for brain tumor severity prediction
International Journal of Electrical and Computer Engineering
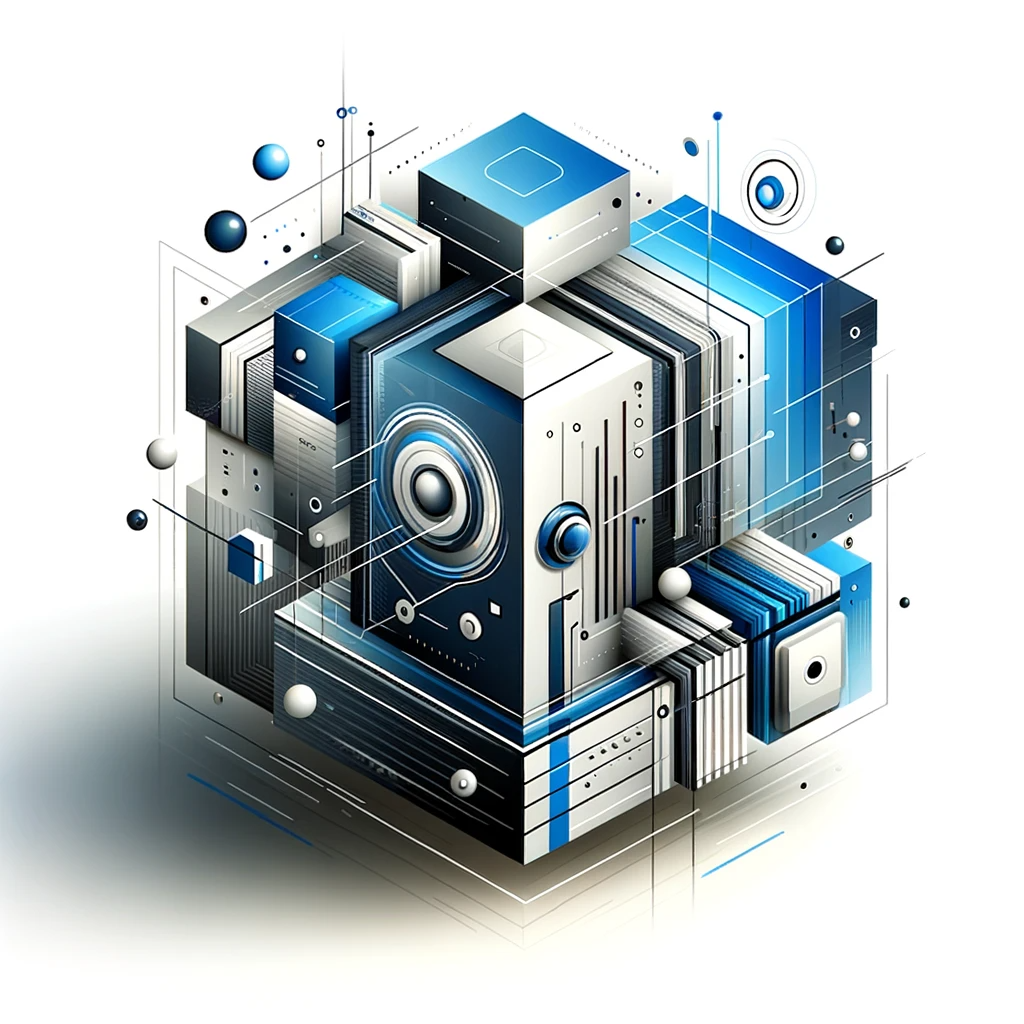
Abstract
Abnormality in brain tissues is a life-threatening illness in humans Un-bias to gender and age if it is unrecognized and untreated within time, will lead to severe complications and extreme conditions. The brain tumor is mainly influenced by a variety of unpredicted and unavoidable reasons. Its evaluation, spread pattern, and identification involves complex assignment. Its early grading and the proper classification ensure effective treatment. The proposed work attempts to extract and classify the tumor region using an automatic classification system for magnetic resonance imaging (MRI) brain tumors. A deep learning convolutional neural network-based architecture YOLO is employed to classify and detect the tumor from brain MR images. The proposed method resulted in superior segmentation, and classification performance in terms of subjective visualization and objective metrics as compared to state of art approaches. The proposed YOLO-based method collectively achieved 98.89% classification accuracy on the BRAINIX and Kaggle datasets.
Discover Our Library
Embark on a journey through our expansive collection of articles and let curiosity lead your path to innovation.
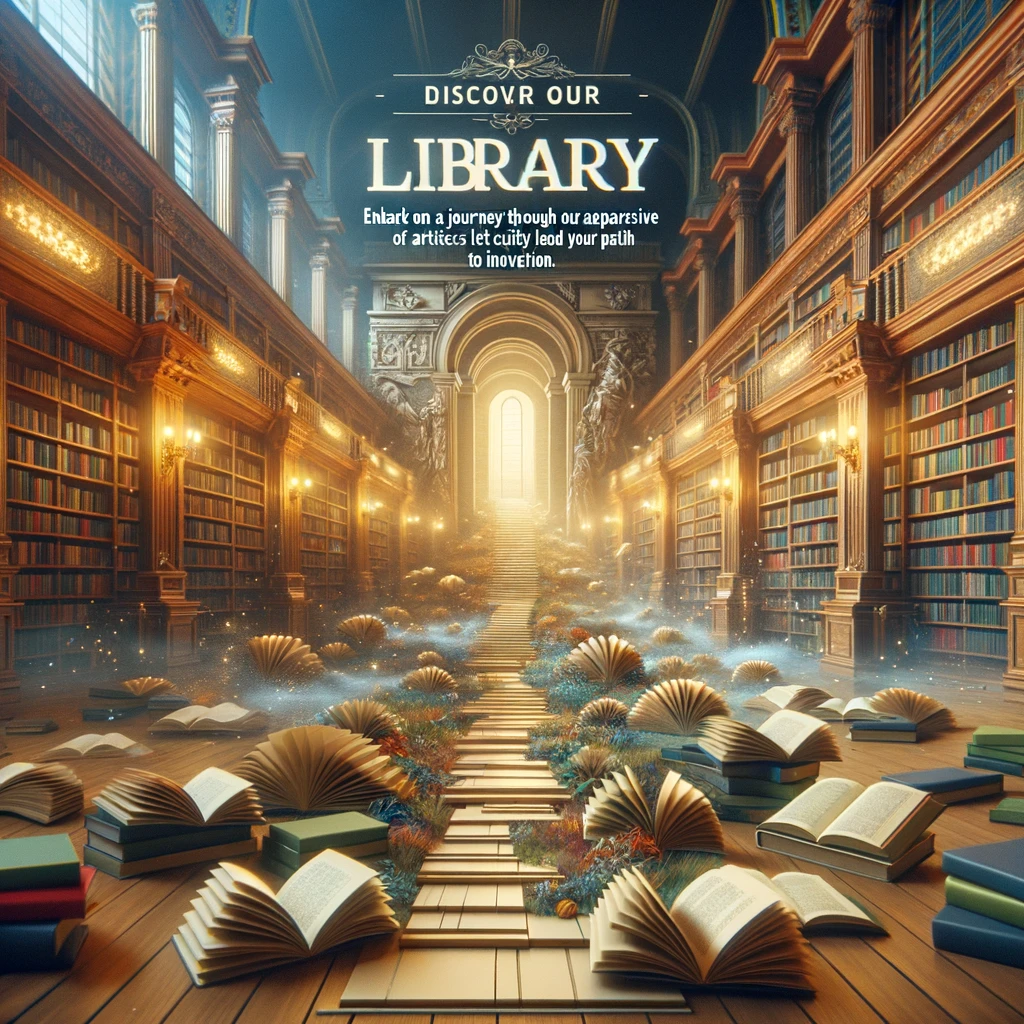