Efficient deep learning approach for enhancing plant leaf disease classification
Indonesian Journal of Electrical Engineering and Computer Science
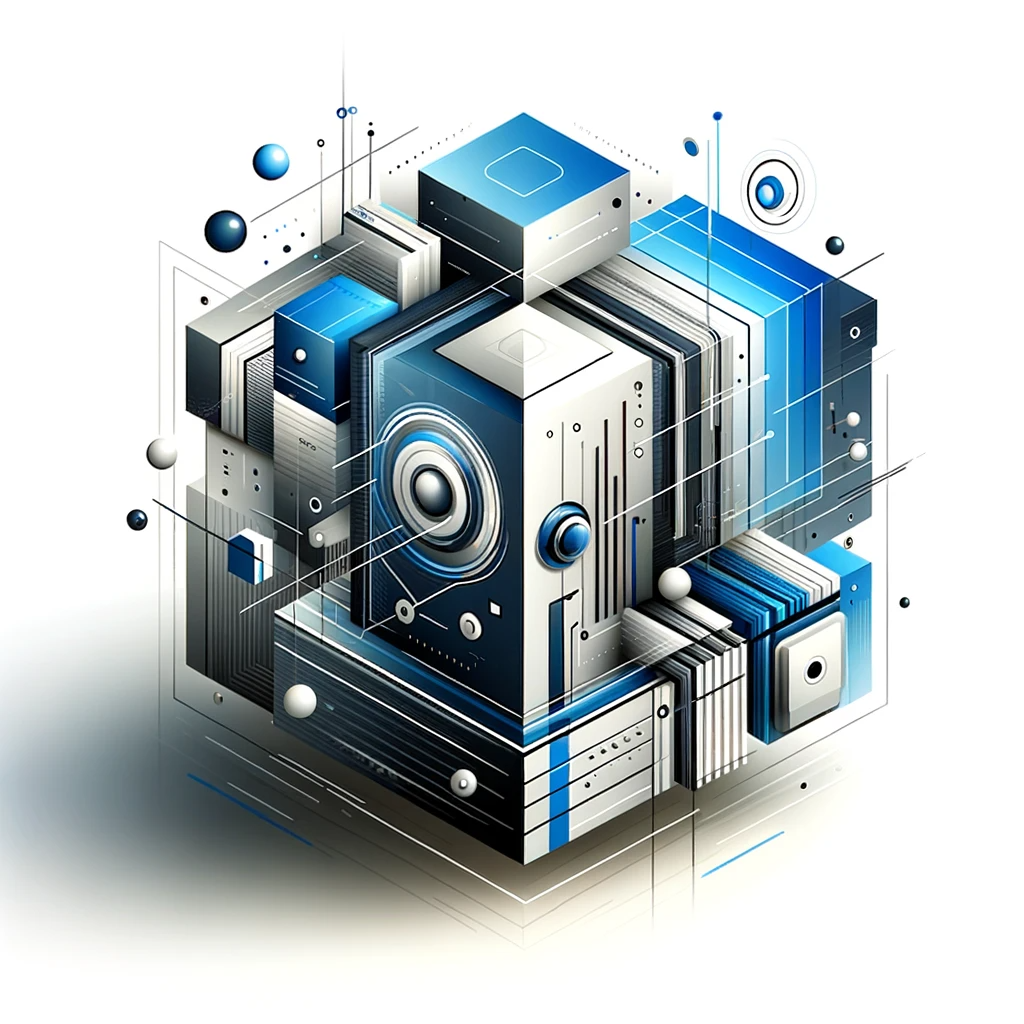
Abstract
The widespread occurrence of plant diseases is a major factor in the reduction of agricultural output, affecting both crop quality and quantity. These diseases typically begin on the leaves, influenced by alterations in plant structure and growing techniques, and can eventually spread over the entire plant. This results in a notable decrease in crop variety and yield. Successfully managing these diseases depends on accurately classifying and detecting leaf infections early, which is essential for controlling their spread and ensuring healthy plant growth. To address these challenges, this paper introduces an efficient approach for detecting plant leaf diseases. A concatenation of pre-trained convolutional neural networks (CNN) for enhanced plant leaf disease using transfer learning technique is implemented, with a specific focus on accurate early detection, utilizing the comprehensive new plant diseases dataset. The combined residual network-50 (ResNet-50) with densely connected convolutional network-121 (DenseNet-121) architecture aims to provide an efficient and reliable solution to these critical agricultural concerns. Various evaluation metrics were utilized to evaluate the robustness of the proposed hybrid model. The proposed ResNet-50 with the DenseNet-121 hybrid model achieved a rate of accuracy of 99.66%.
Discover Our Library
Embark on a journey through our expansive collection of articles and let curiosity lead your path to innovation.
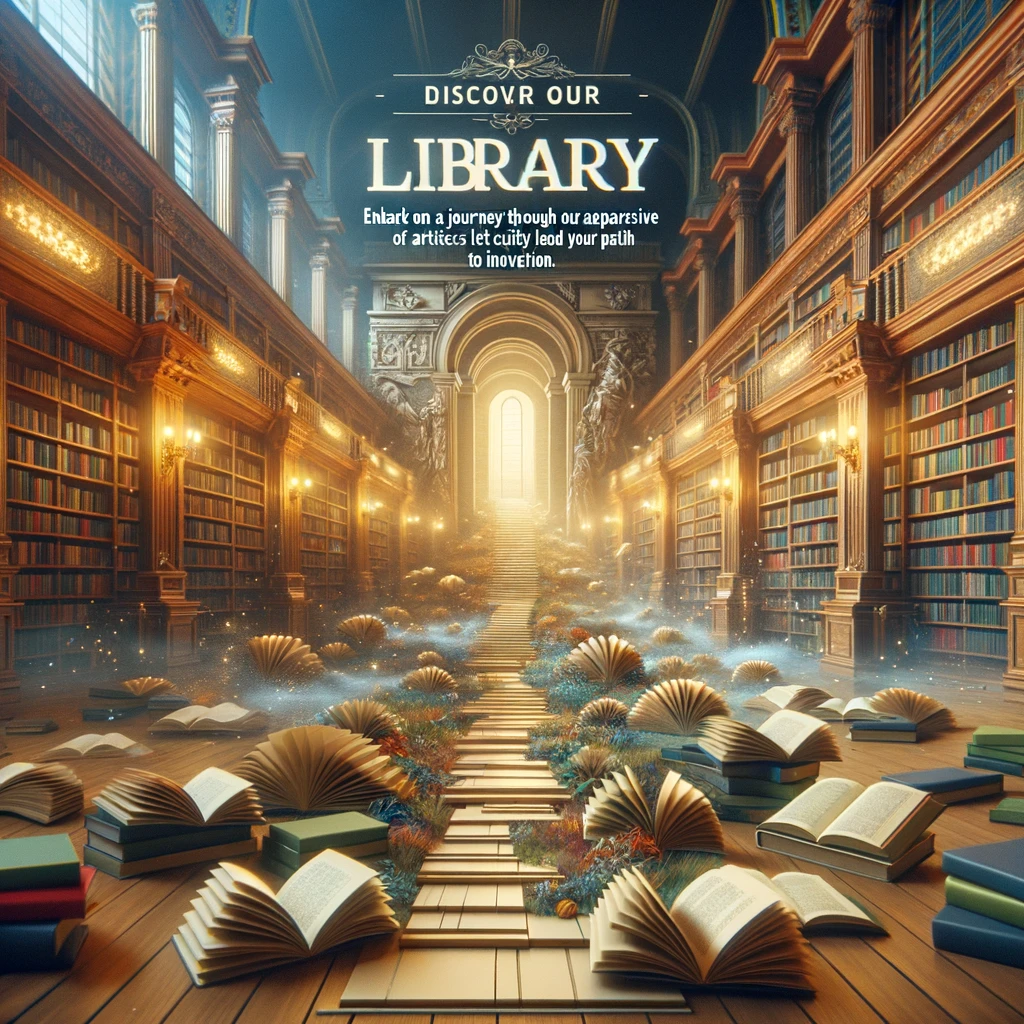