Self-attention encoder-decoder with model adaptation for transliteration and translation tasks in regional language
International Journal of Reconfigurable and Embedded Systems
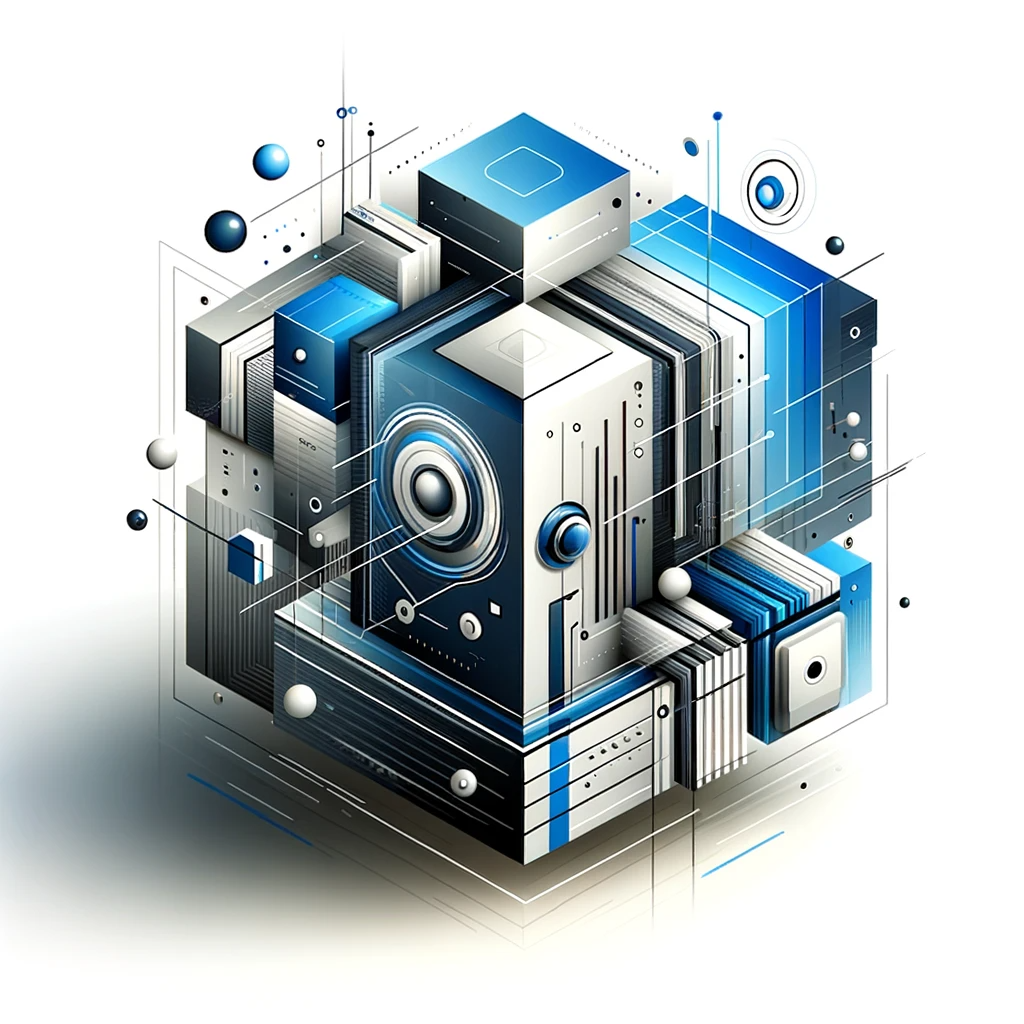
Abstract
The recent advancements in natural language processing (NLP) have highlighted the significance of integrating machine transliteration with translation for enhanced language services, particularly in the context of regional languages. This paper introduces a novel neural network architecture that leverages a self-attention mechanism to create an autoencoder without the need for iterative or convolutional processes. The selfattention mechanism operates on projection matrices, feature matrices, and target queries, utilizing the Softmax function for optimization. The introduction of the self-attention encoder-decoder with model adaptation (SAEDM) represents a breakthrough, marking a substantial enhancement in transliteration and translation accuracy over previous methodologies. This innovative approach employs both student and teacher models, with the student model's loss calculated through the probabilities and prediction labels via the negative log entropy function. The proposed architecture is distinctively designed at the character level, incorporating a word-to-word embedding framework, a beam search algorithm for sentence generation, and a binary classifier within the encoder-decoder structure to ensure the uniqueness of the content. The effectiveness of the proposed model is validated through comprehensive evaluations using transliteration and translation datasets in Kannada and Hindi languages, demonstrating its superior performance compared to existing models.
Discover Our Library
Embark on a journey through our expansive collection of articles and let curiosity lead your path to innovation.
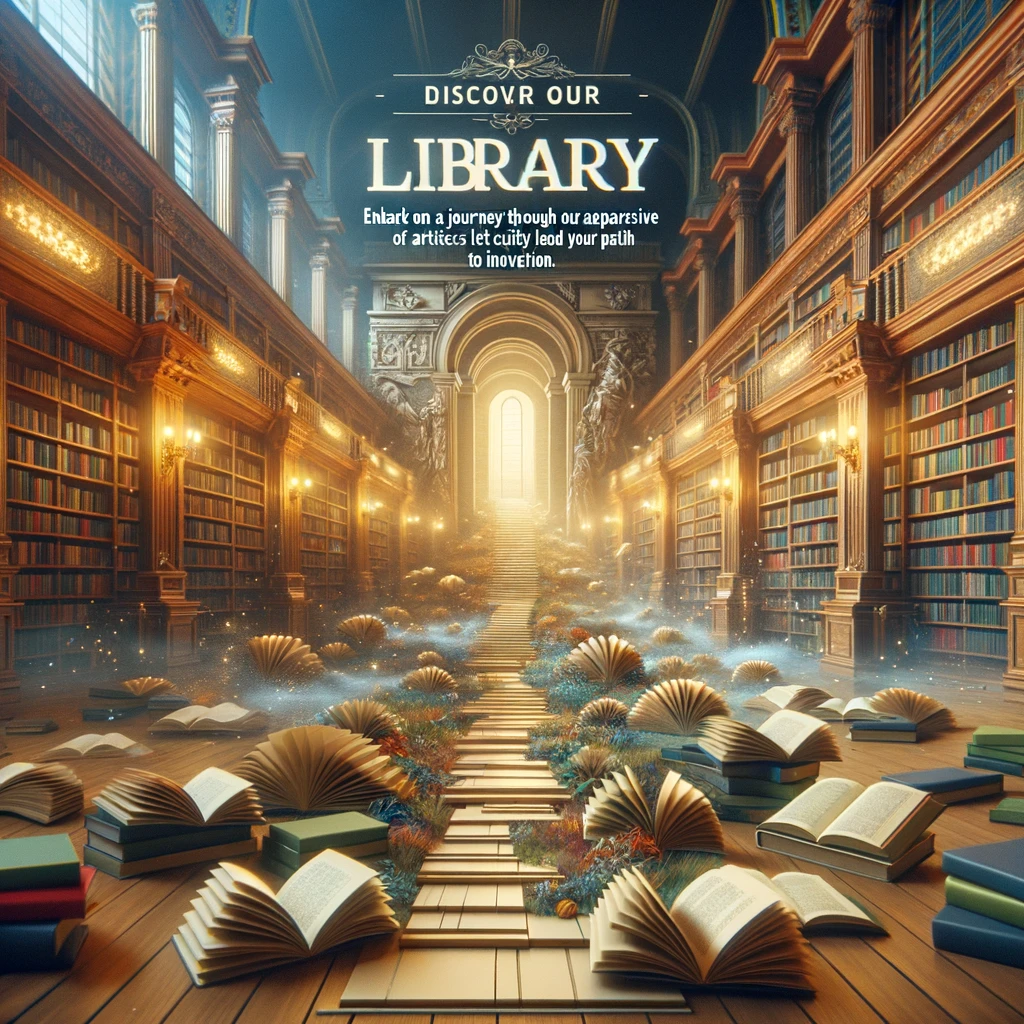