Analysing feature selection: impacts towards forecasting electricity power consumption
International Journal of Reconfigurable and Embedded Systems
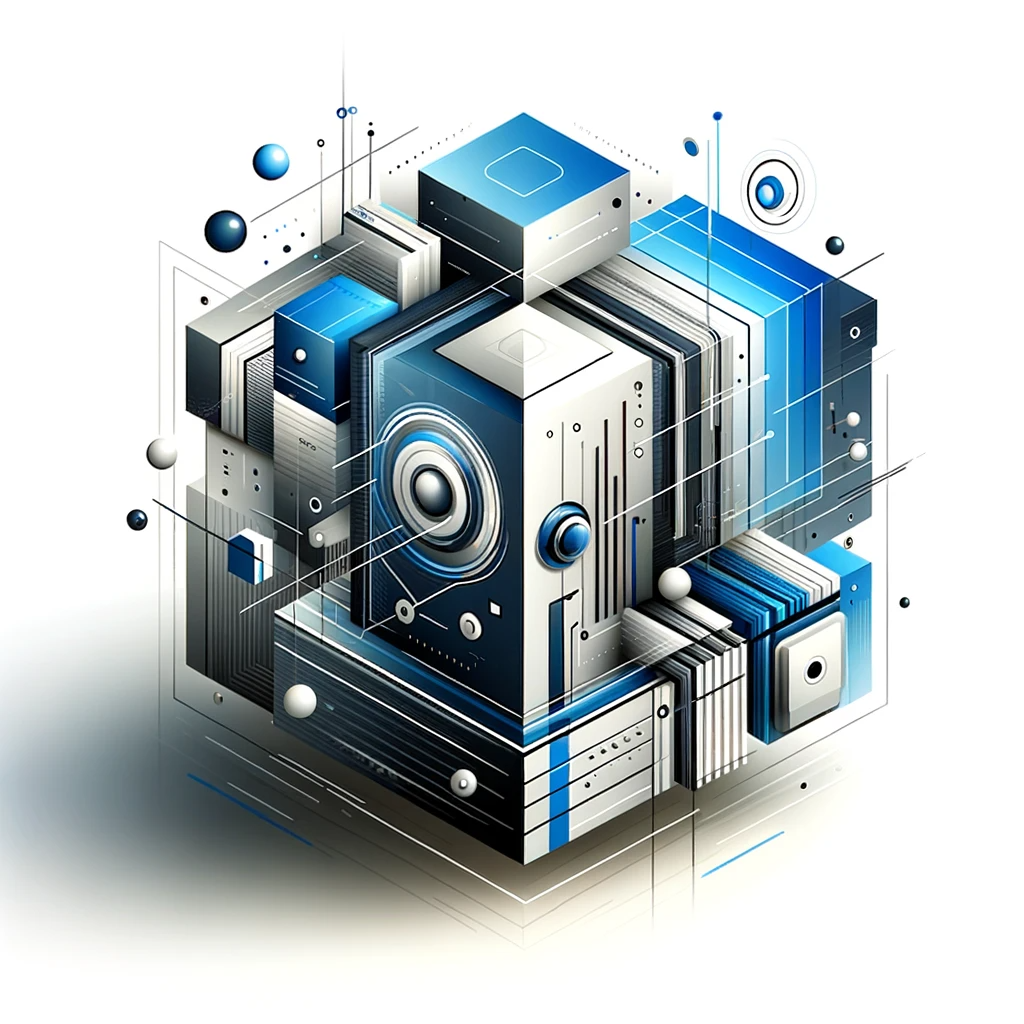
Abstract
This study focuses on the development of electrical power forecasting based on electricity usage in Wuzhou, China. To develop a forecasting model, the important features need to be identified. Therefore, this study investigates the performance of the feature selection method, focusing on the mutual information as a filter and random forest as a wrapper-based feature selection. From the experiment, six features have been chosen, whereby both feature selection methods chose almost identical features. Later, the selected features are trained and tested with common machine learning models, namely random forest regressor, support vector regression (SVR), k-nearest neighbor (KNN) regressor, and extreme gradient boosting (XGBoost) regressor. The performances of the feature selections tested on each of the models are measured in terms of mean absolute error (MAE), root mean square error (RMSE) and coefficient of determination (R²). Findings from the experiment revealed that XGBoost outperform the other machine learning models with RMSE 0.9566 and R² indicated with 0.2561. However, SVR outperformed XGBoost and other model by obtaining MAE 0.6028. It can be concluded that the performance of filter-based outperformed the embedded feature selection.
Discover Our Library
Embark on a journey through our expansive collection of articles and let curiosity lead your path to innovation.
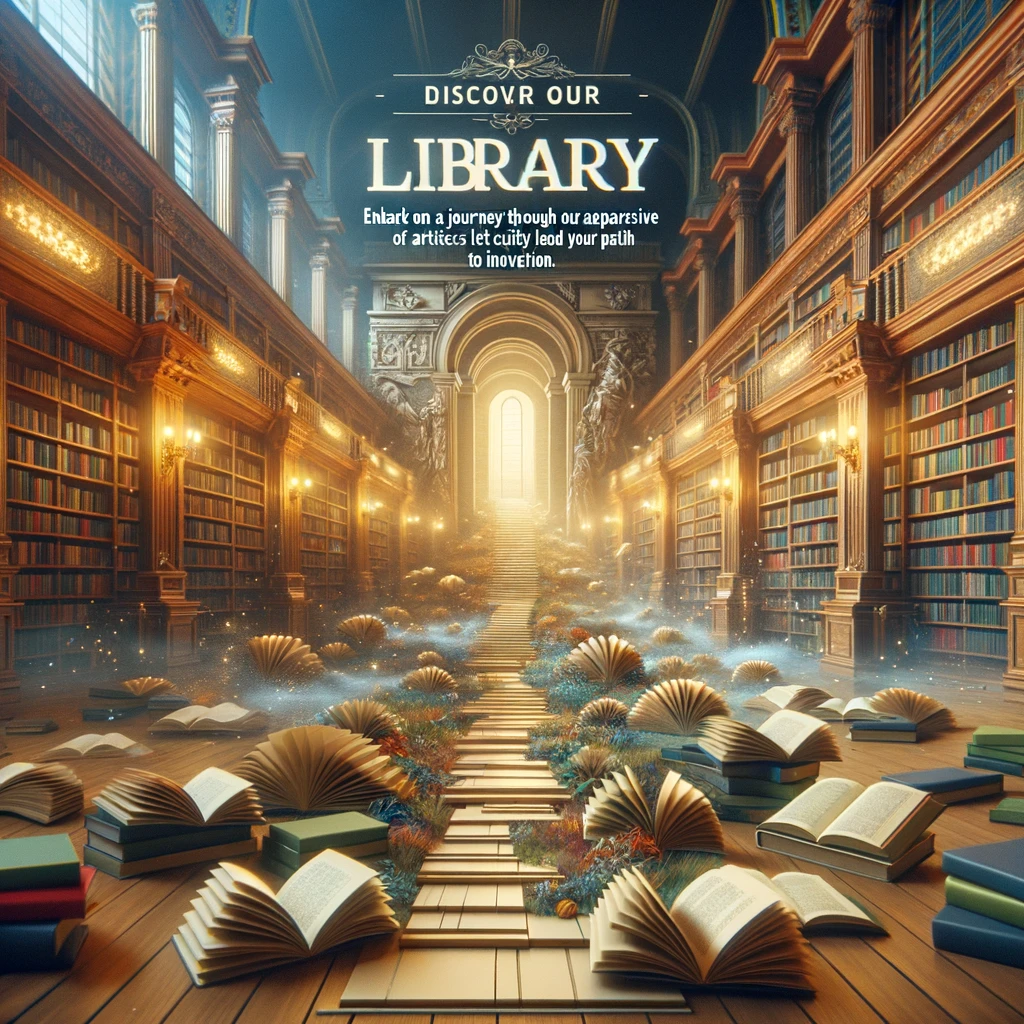