A novel dataset and part-of-speech tagging approach for enhancing sentiment analysis in Kannada
Indonesian Journal of Electrical Engineering and Computer Science
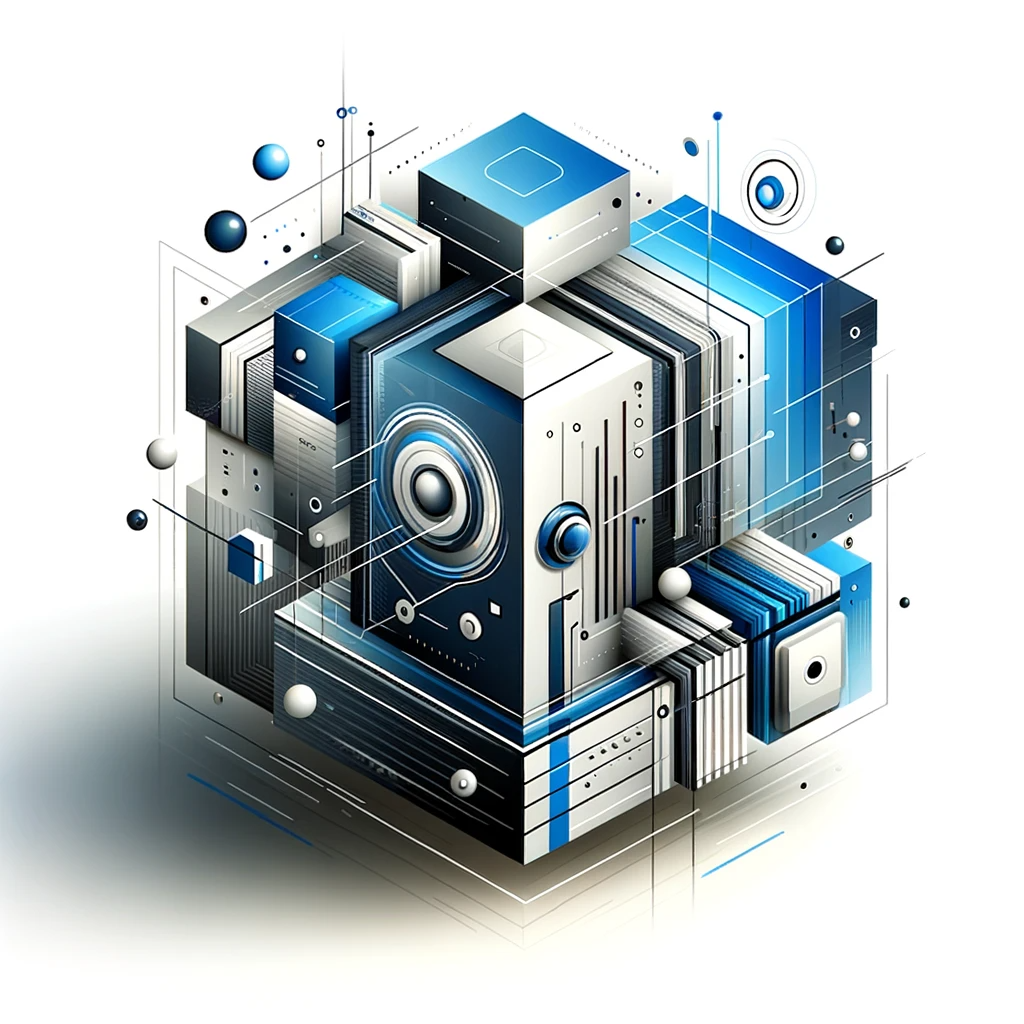
Abstract
The problem addressed in this research is the limited availability of labelled datasets and effective sentiment analysis tools for the Kannada language. Existing challenges include linguistic variations, cultural diversities, and the absence of comprehensive datasets designed specifically for sentiment analysis in Kannada. This research aims to enhance sentiment analysis capabilities for the Kannada language, addressing challenges posed by linguistic variations and limited labelled datasets. A novel Kannada dataset derived from SemEval 2014 task 4 was created using a conversion process. The dataset was processed using part-of-speech tagging, and a specialized model called K-BERT (Kannada bidirectional encoder representations from transformers) was introduced and implemented using Python within the Anaconda environment. Performance evaluation results showcased K-BERT's superiority over traditional machine learning (ML) algorithms and the BERT model, achieving an accuracy of 0.98, precision of 0.97, recall of 0.97, and F-score of 0.98 in sentiment classification for Kannada text data. This work contributes a unique Kannada dataset, introduces the K-BERT model specifically designed for Kannada sentiment analysis, and emphasizes the importance of collaborative efforts in advancing natural language processing (NLP) research for multilingual environments.
Discover Our Library
Embark on a journey through our expansive collection of articles and let curiosity lead your path to innovation.
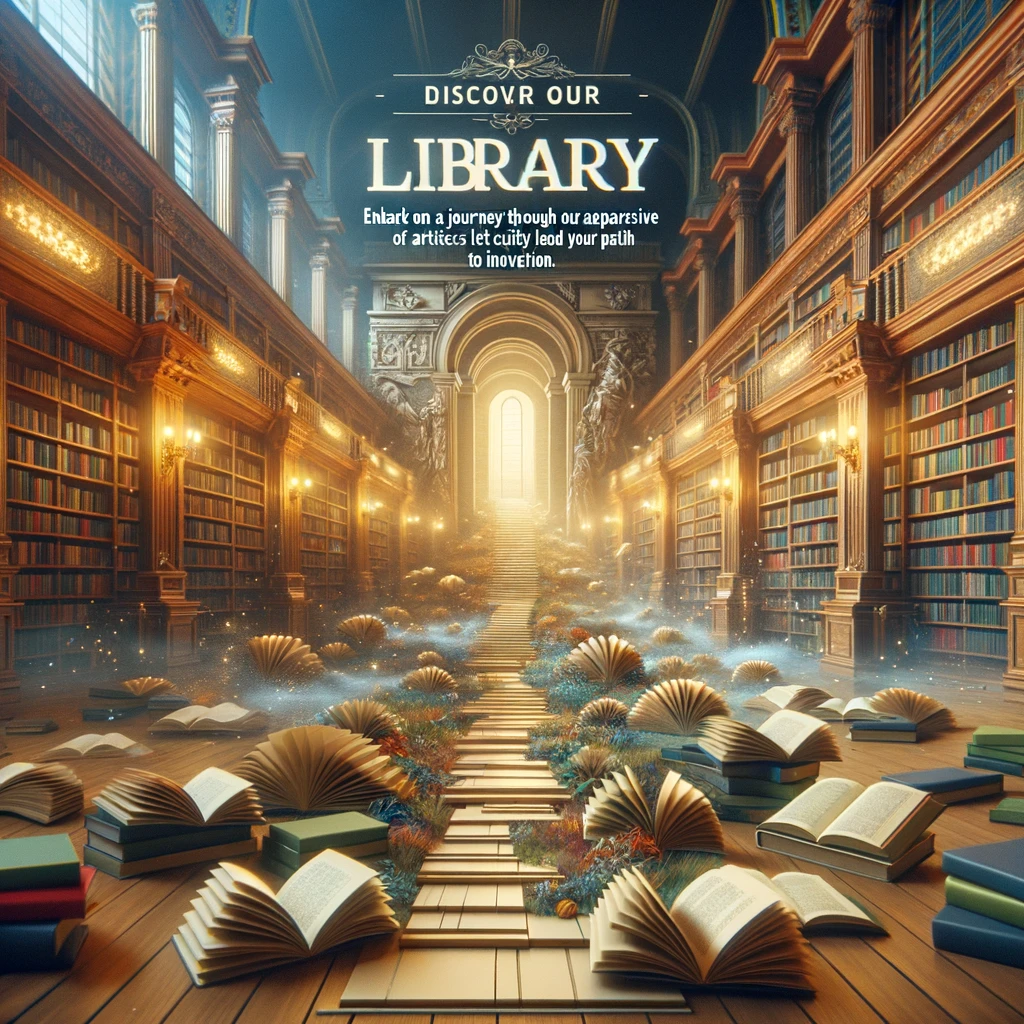