Predicting student status using machine learning by analyzing classroom behaviors with X-API data
Indonesian Journal of Electrical Engineering and Computer Science
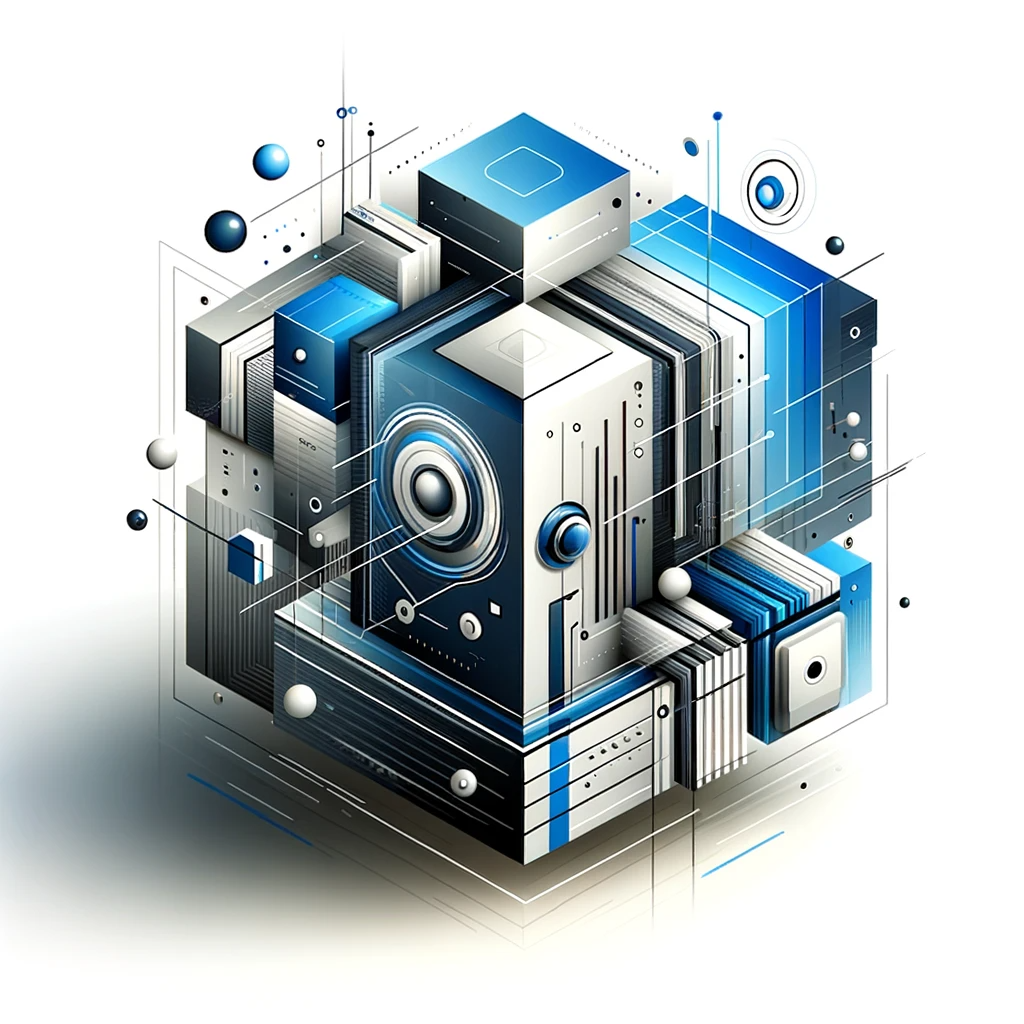
Abstract
We explore the emergence and growing significance of educational data mining, a field dedicated to extracting valuable insights from vast datasets gathered from diverse educational environments. Utilizing the experience API (XAPI) and the Kalboard 360 online learning platform, our research presents a novel behaviorally based student performance model that evaluates the influence of student interactions on academic results. We create reliable models for precisely projecting academic success by utilizing machine learning techniques including logistic regression, k-nearest neighbors (KNNs), support vector machines (SVM), decision trees, random forests (RF), and XGBoost. The outcomes show a notable increase in categorization accuracy. Through the personalization of instruction, formative assessment support, and proactive identification of each student's unique needs to maximize their learning experience, this approach holds the potential to improve educational processes.
Discover Our Library
Embark on a journey through our expansive collection of articles and let curiosity lead your path to innovation.
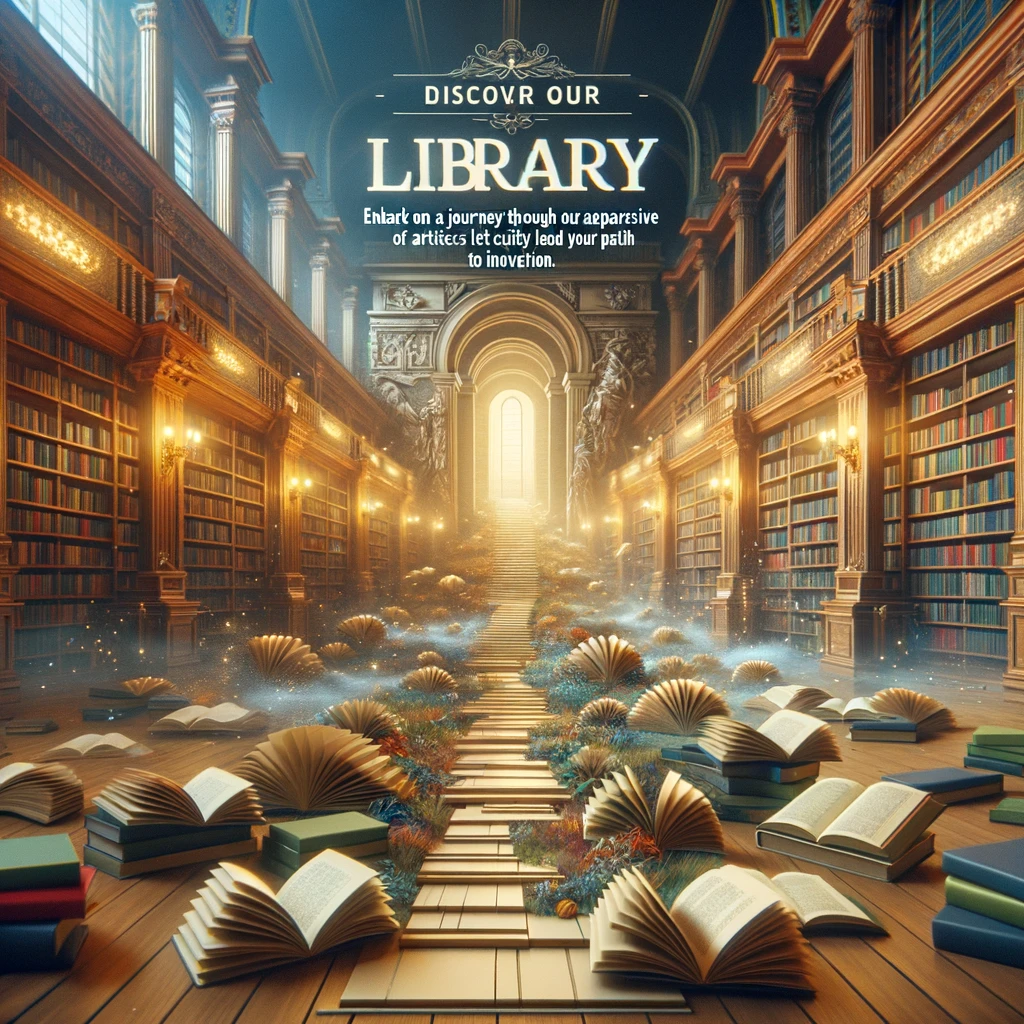