Machine learning models in renewable energy forecasting: a systematic literature review
Indonesian Journal of Electrical Engineering and Computer Science
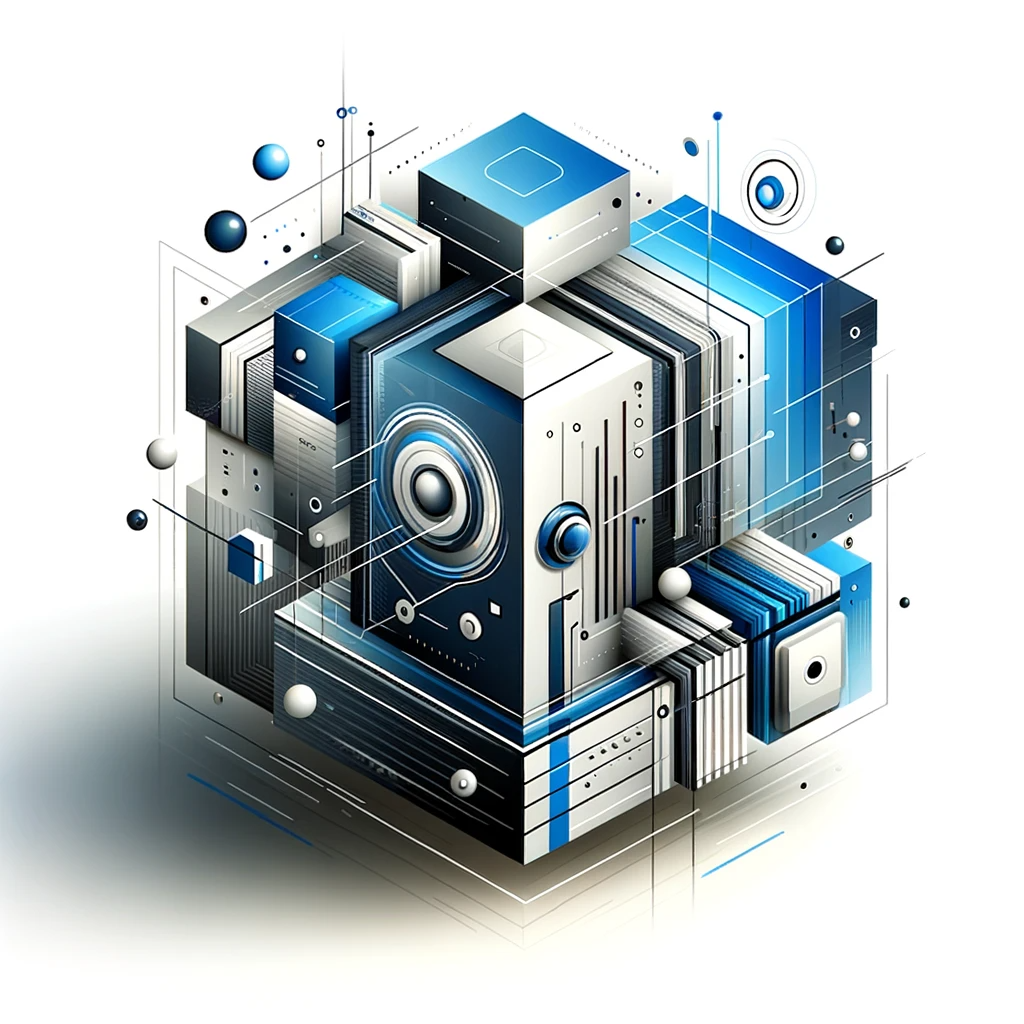
Abstract
During the past years, the convergence of machine learning (ML) technologies with renewable energy sectors has become a significant key area of innovation as a key area of innovation, enhancing the efficiency and predictability of sustainable energy sources. ML algorithms, adept at handling complex data, have become essential in forecasting energy outputs from variable sources like solar and wind. This integration has led to the development of smarter, more adaptive grid systems, capable of efficiently managing the variability of renewable energy sources. This review paper focuses on several key areas: firstly, it provides a summary of related work, specifically focusing on ML in the renewable energy field. Secondly, it delves into ML models and evaluation metrics used for solar and wind energy forecasting. Thirdly, it analyzes 21 studies published from 2019 to 2023, primarily centered on solar energy (60%) and wind energy (40%), with an emphasis on various forecasting horizons, highlighting the results of the ML algorithms used and the performance metrics to evaluate their effectiveness. Finally, it identifies gaps and opportunities in this field. The state-of-the-art review and its findings can offer a solid foundation for future research initiatives.
Discover Our Library
Embark on a journey through our expansive collection of articles and let curiosity lead your path to innovation.
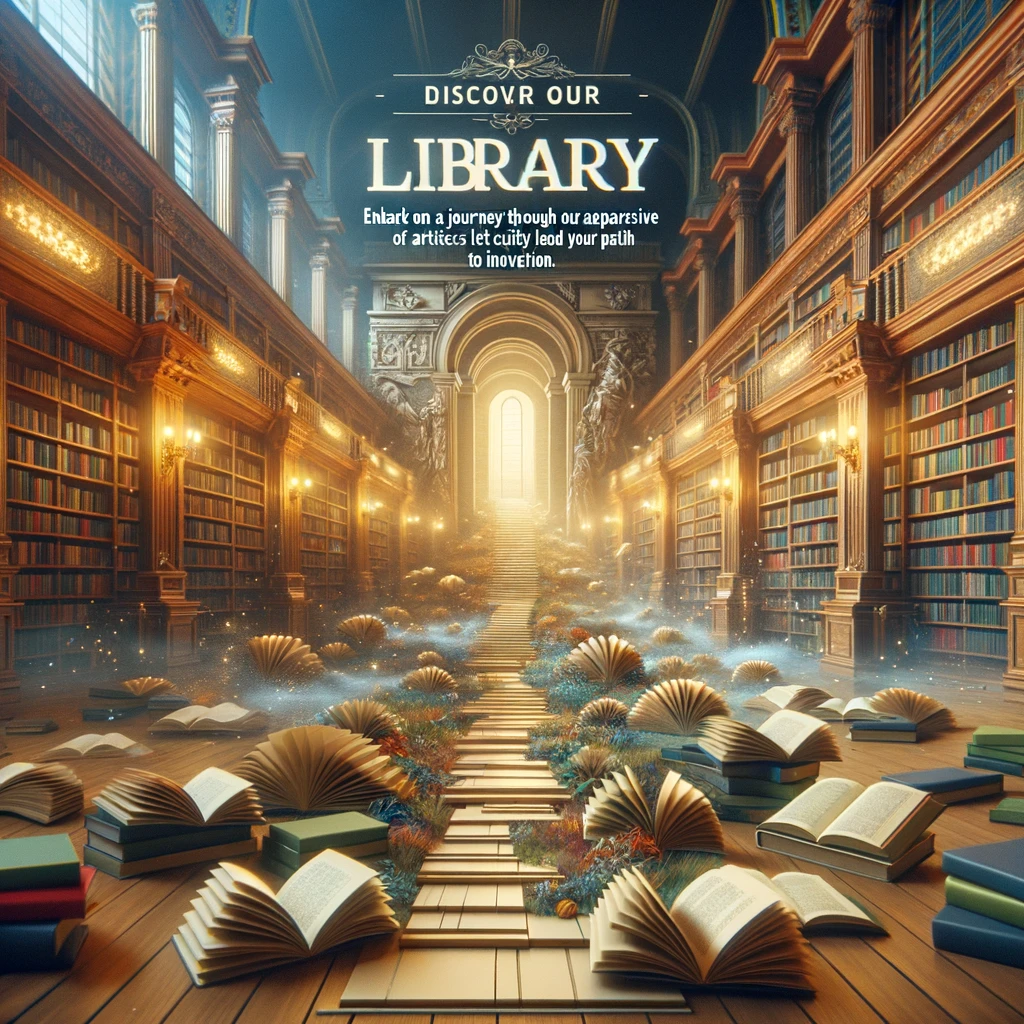