Optimization techniques applied on image segmentation process by prediction of data using data mining techniques
International Journal of Electrical and Computer Engineering
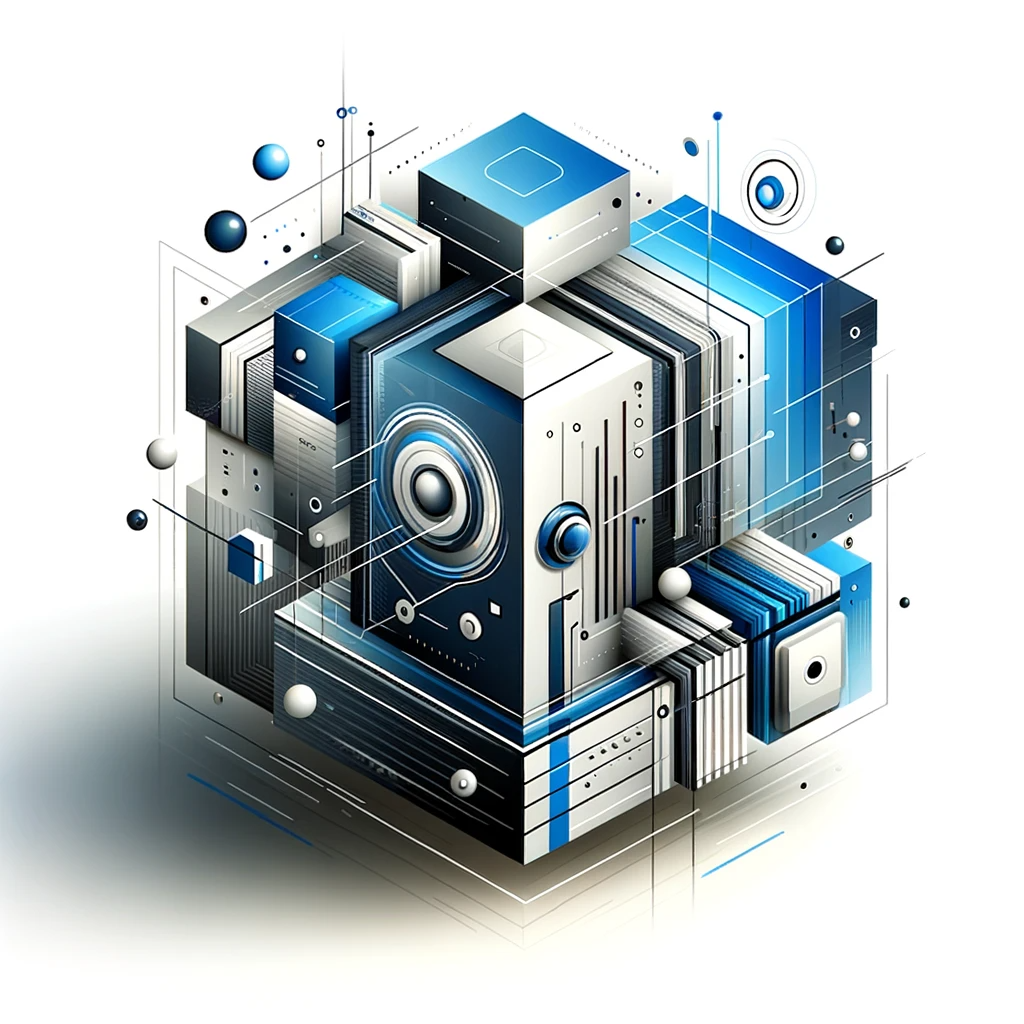
Abstract
The research work presents an enhanced method that combines rule-based color image segmentation with fuzzy density-based spatial clustering of applications with noise (FDBSCAN). This technique enhances super-pixel robustness and improves overall image quality, offering a more effective solution for image segmentation. The study is specifically applied to the challenging and novel task of predicting the age of tigers from camera trap images, a critical issue in the emerging field of wildlife research. The task is fraught with challenges, particularly due to variations in image scale and thickness. Proposed methods demonstrate that significant improvements over existing techniques through the broader set of parameters of min and max to achieve superior segmentation results. The proposed approach optimizes segmentation by integrating fuzzy clustering with rule-based techniques, leading to improved accuracy and efficiency in processing color images. This innovation could greatly benefit further research and applications in real-world scenarios. Additionally, the scale and thickness variations of the present barracuda panorama knowledge base offer many advantages over other enhancement strategies that have been proposed for the use of these techniques. The experiments show that the proposed algorithm can utilize a wider range of parameters to achieve better segmentation results.
Discover Our Library
Embark on a journey through our expansive collection of articles and let curiosity lead your path to innovation.
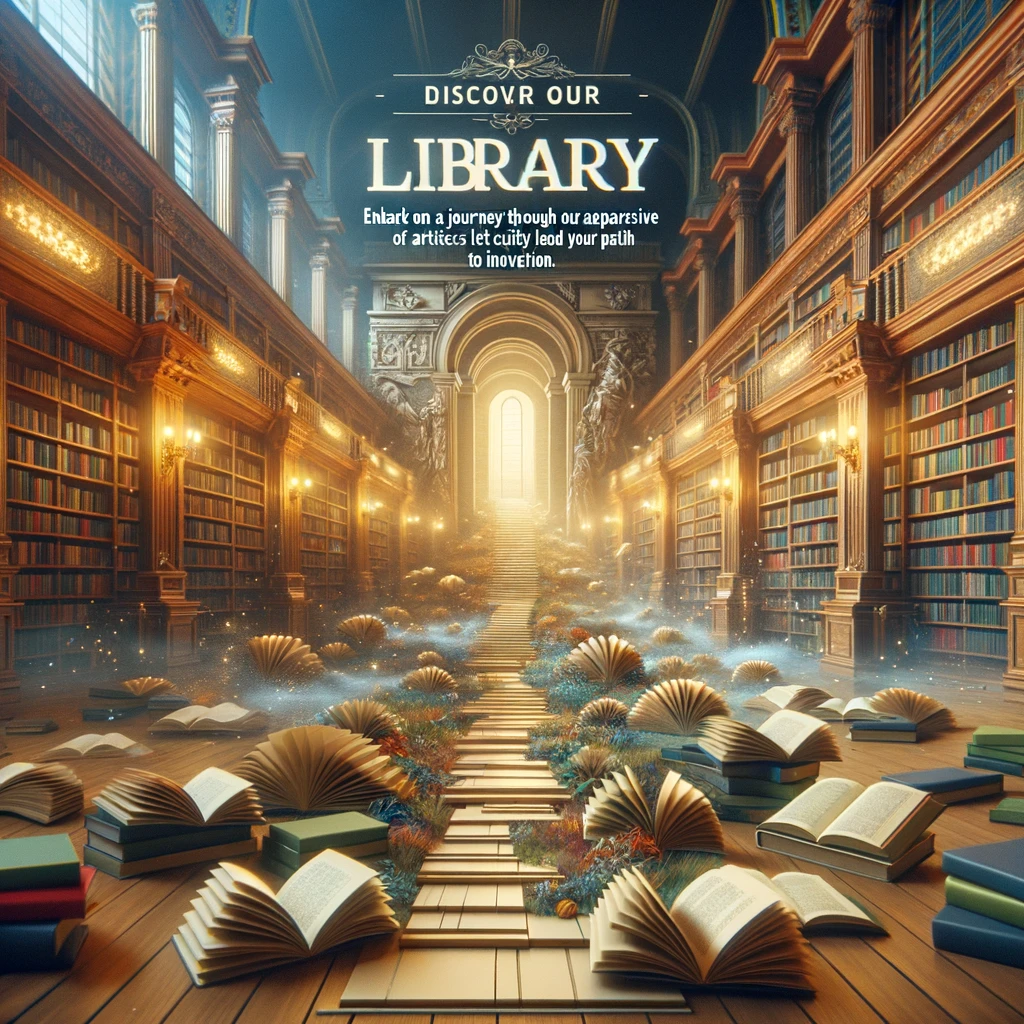