Advancing network security: a comparative research of machine learning techniques for intrusion detection
International Journal of Electrical and Computer Engineering
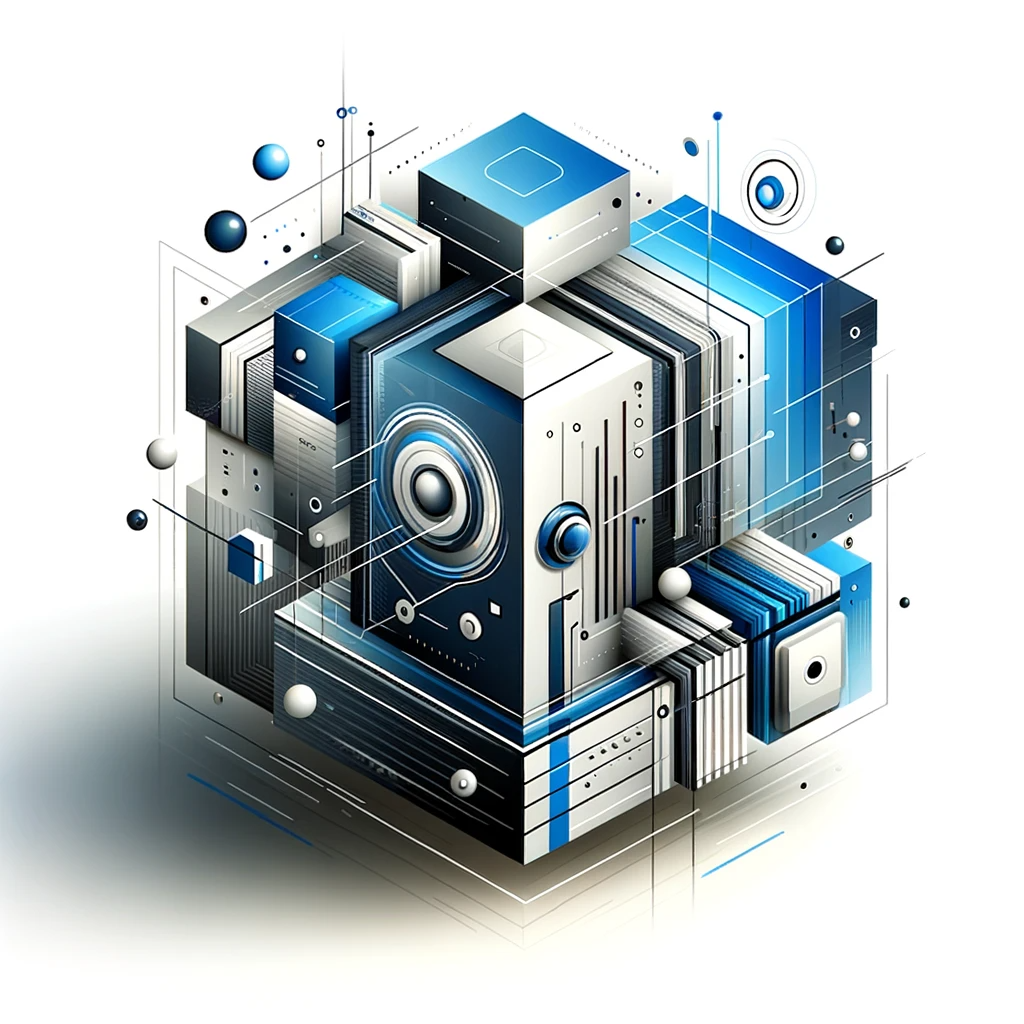
Abstract
In the current digital era, the advancement of network-based technologies has brought a surge in security vulnerabilities, necessitating complex and dynamic defense mechanisms. This paper explores the integration of machine learning techniques within intrusion detection systems (IDS) to tackle the intricacies of modern network threats. A detailed comparative analysis of various algorithms, including k-nearest neighbors (KNN), logistic regression, and perceptron neural networks, is conducted to evaluate their efficiency in detecting and classifying different types of network intrusions such as denial of service (DoS), probe, user to root (U2R), and remote to local (R2L). Utilizing the national software laboratory knowledge discovery and data mining (NSL-KDD) dataset, a standard in the field, the study examines the algorithms’ ability to identify complex patterns and anomalies indicative of security breaches. Principal component analysis is utilized to streamline the dataset into 20 principal components for data processing efficiency. Results indicate that the neural network model is particularly effective, demonstrating exceptional performance metrics across accuracy, precision, and recall in both training and testing phases, affirming its reliability and utility in IDS. The potential for hybrid models combining different machine learning (ML) strategies is also discussed, highlighting a path towards more robust and adaptable IDS solutions.
Discover Our Library
Embark on a journey through our expansive collection of articles and let curiosity lead your path to innovation.
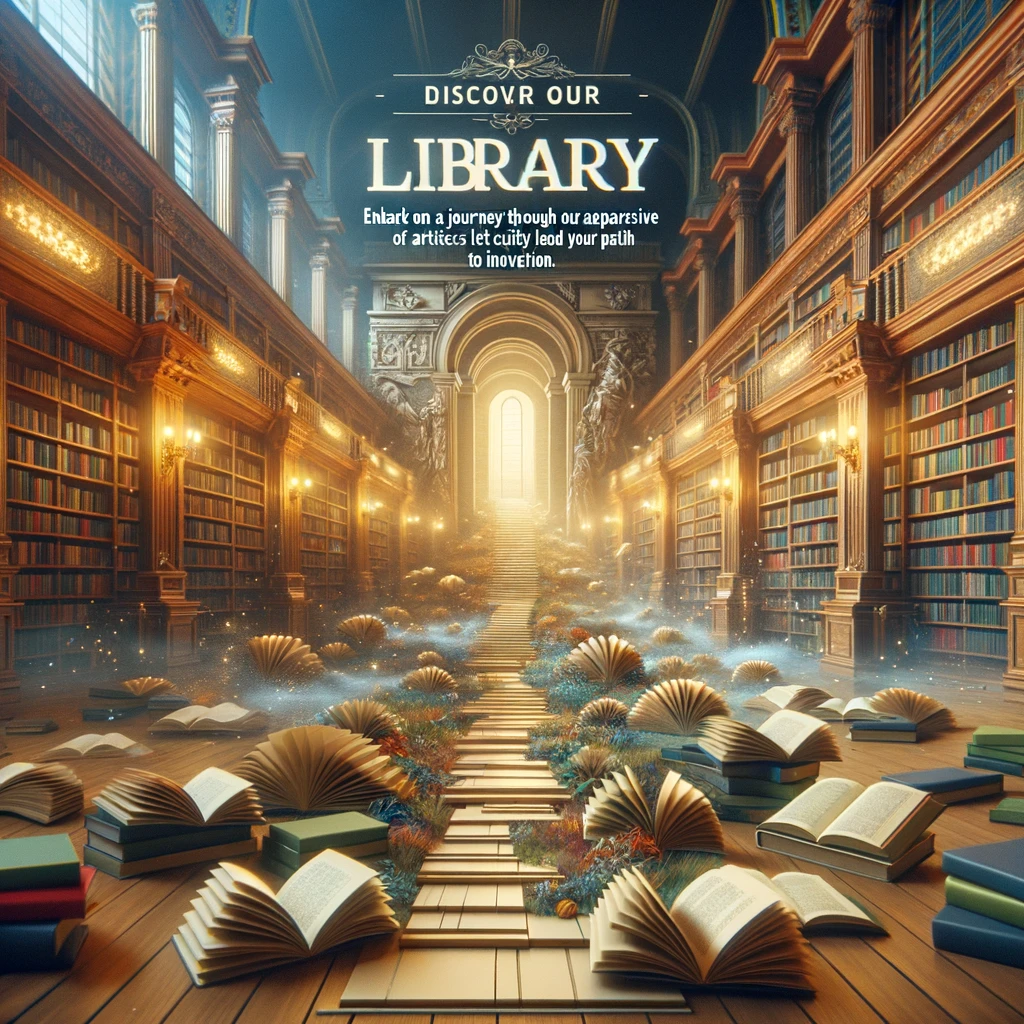