Advanced stress detection with optimized feature selection and hybrid neural networks
International Journal of Electrical and Computer Engineering
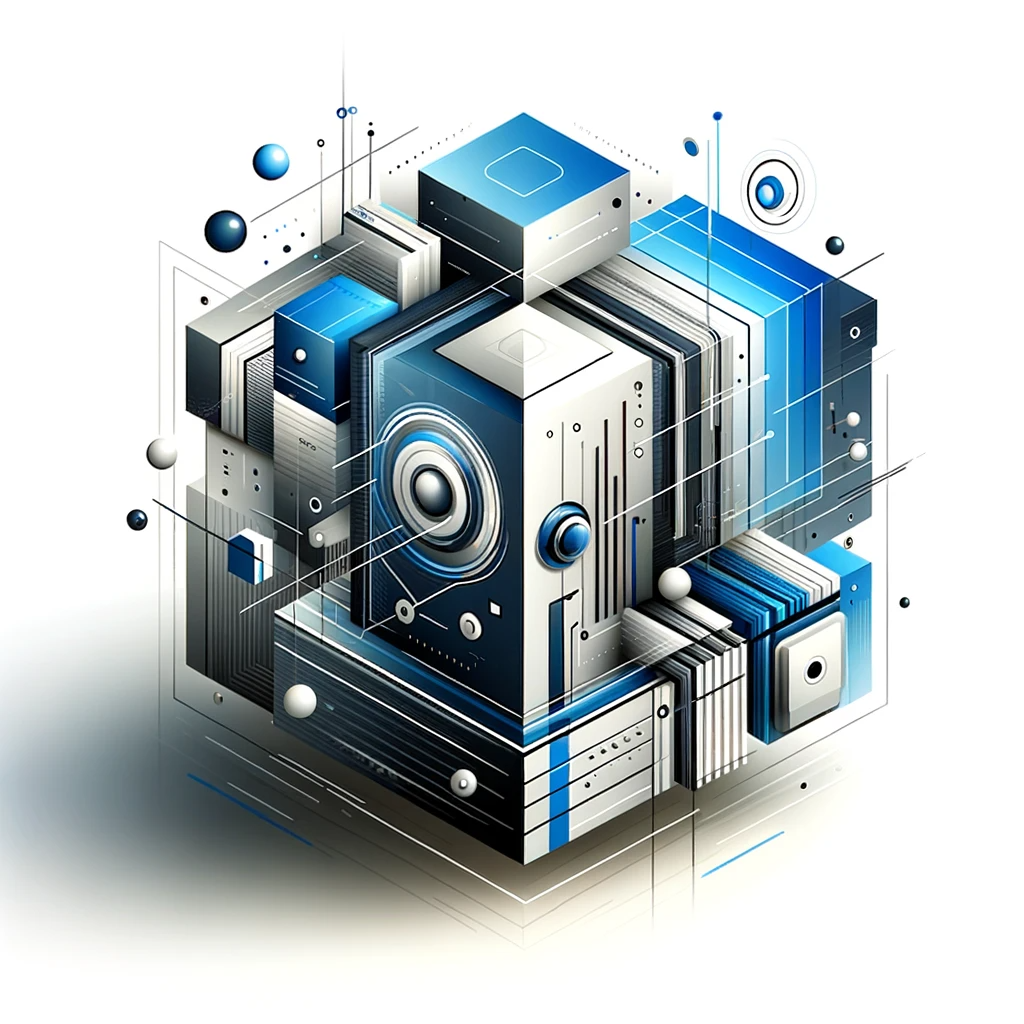
Abstract
Stress impacts both mental and physical health, potentially leading to serious conditions like cardiovascular diseases and mental disorders. Early detection of stress is crucial for reducing these risks. This study aims to improve stress detection by analyzing physiological signals, specifically electroencephalography (EEG) and electrocardiogram (ECG). EEG is affordable, while ECG provides detailed insights into cardiovascular health. Feature selection is a major challenge in analyzing these signals. To address this, the research introduces a novel method that combines the Archimedes optimization algorithm (AoA) with the analytical hierarchical process (AHP) to enhance accuracy in both single and multimodal systems. The proposed multimodal system employs a parallel-structured convolutional neural network (CNN) with a deep architecture to extract spatial features and uses a long short-term memory (LSTM) network to capture temporal dynamics. Experimental results show significant improvements: ECG stress detection accuracy rises from 88.6% to 91.79%, EEG accuracy increases from 95% to 96.6%, and multimodal stress detection accuracy reaches 98.6%. These results highlight the effectiveness of the AoA-AHP-based feature selection technique in boosting stress detection accuracy, contributing to improved mental health management and overall well-being.
Discover Our Library
Embark on a journey through our expansive collection of articles and let curiosity lead your path to innovation.
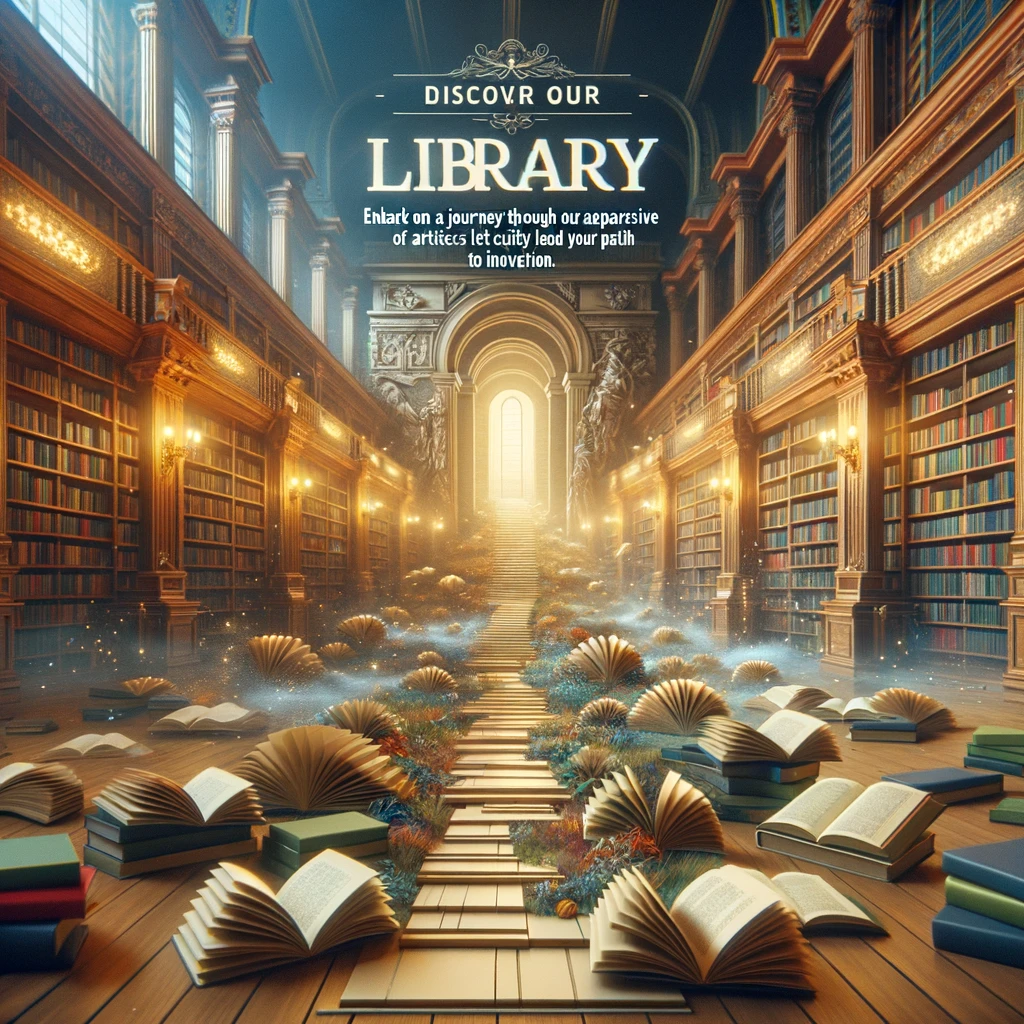