Enhancing accuracy in greenhouse microclimate forecasting through a hybrid long short-term memory light gradient boosting machine ensemble approach
International Journal of Electrical and Computer Engineering
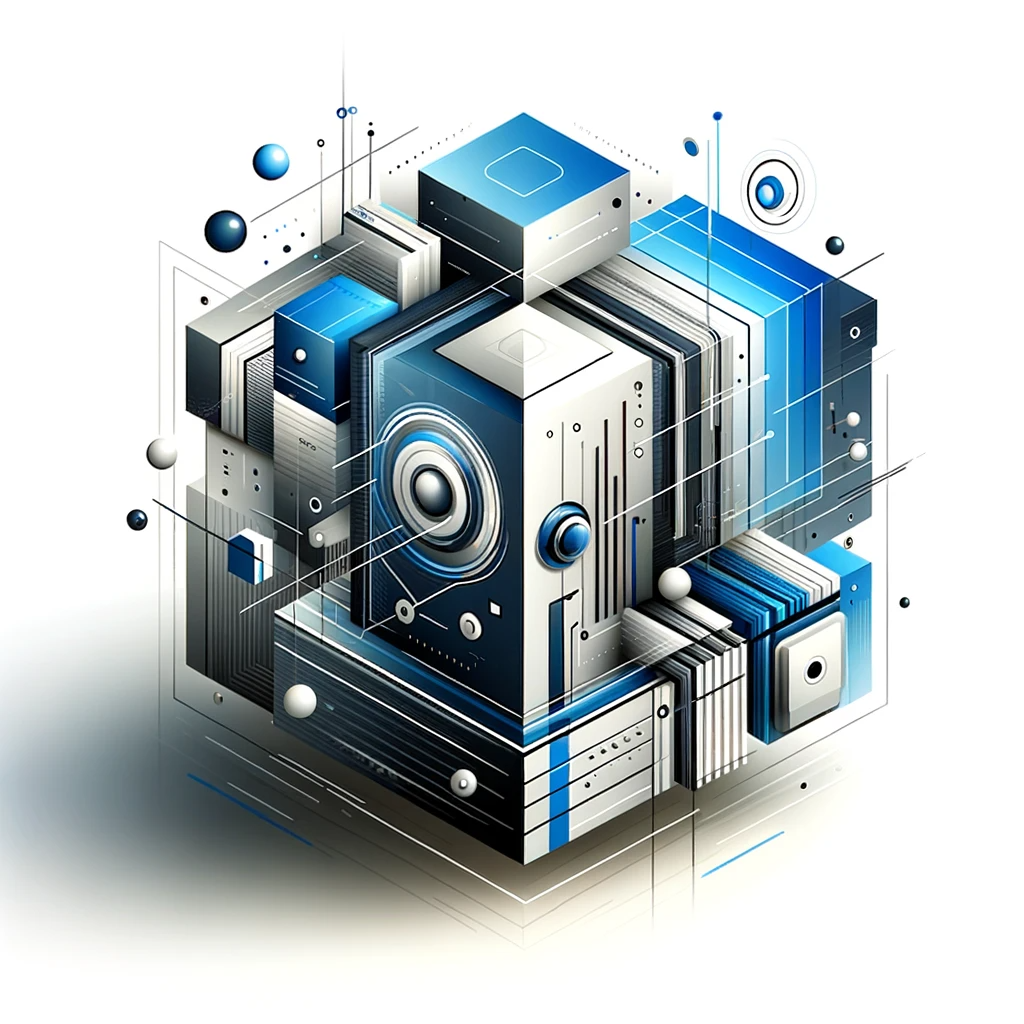
Abstract
Greenhouse cultivation is one of the main methods for improving agricultural yield and quality. With the world needing more and more production, improving greenhouses using innovative technology becomes a must. These high-tech, aka, smart greenhouses depend much on the accuracy and availability of sensor data to perform at their best. In challenging situations such as sensor malfunctions or data gaps, utilizing historical data to predict microclimate parameters within the greenhouse is essential for maintaining optimal growing conditions and effective sustainable resource management control. In this work, and by employing a synthesis technique across various time series models, we forecast internal temperature and humidity, the two main parameters for a greenhouse, by incorporating diverse characteristics as input into a customized forecasting model. The selected architecture integrates deep learning and nonlinear learning models, specifically long short-term memory (LSTM) and light gradient boosting machine (LightGBM) as an ensemble approach, providing a comprehensive framework for time-series prediction, evaluated through mean absolute error (MAE), root mean square error (RMSE), and coefficient of determination (R²) metrics. With a focus on improving accuracy in anticipating environmental changes, we have achieved high precision in predicting temperature (98.45%) and humidity (99.61%).
Discover Our Library
Embark on a journey through our expansive collection of articles and let curiosity lead your path to innovation.
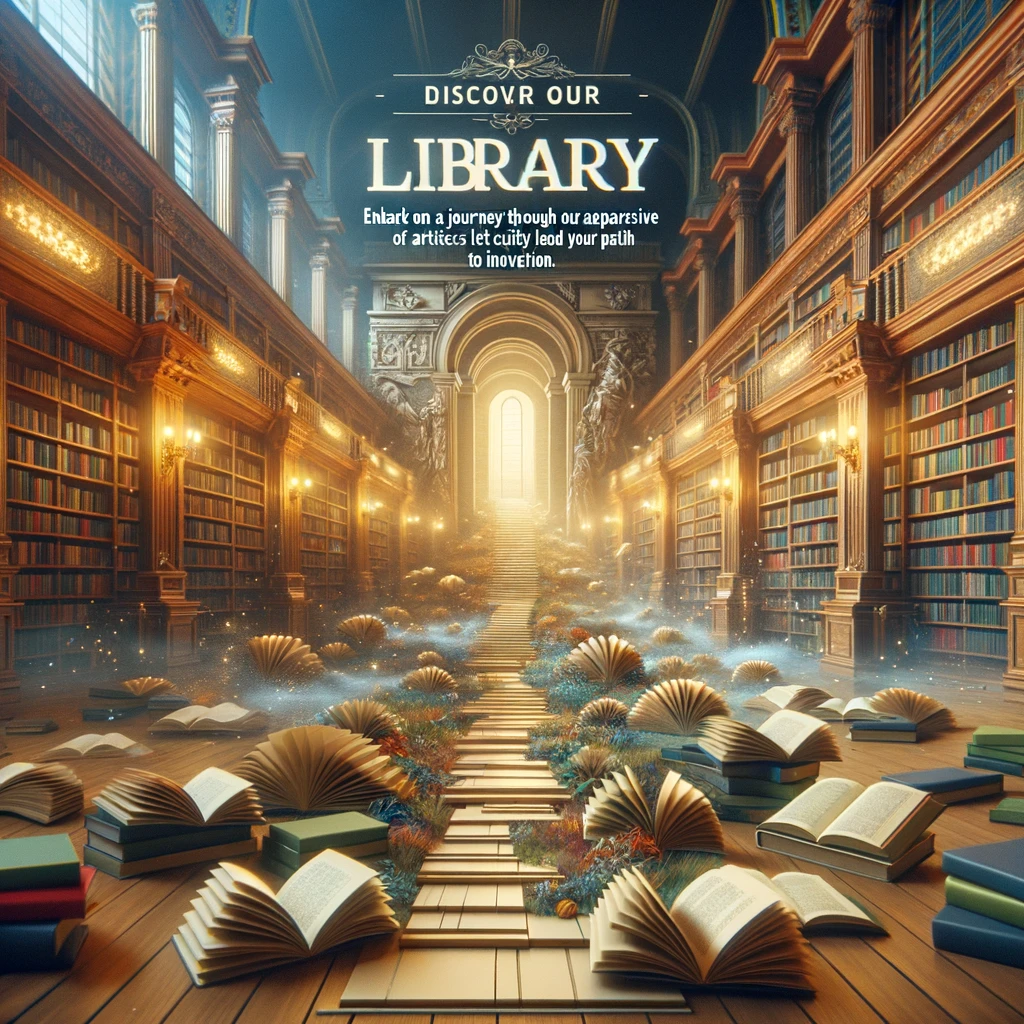