Stress detection through wearable sensors: a convolutional neural network-based approach using heart rate and step data
International Journal of Electrical and Computer Engineering
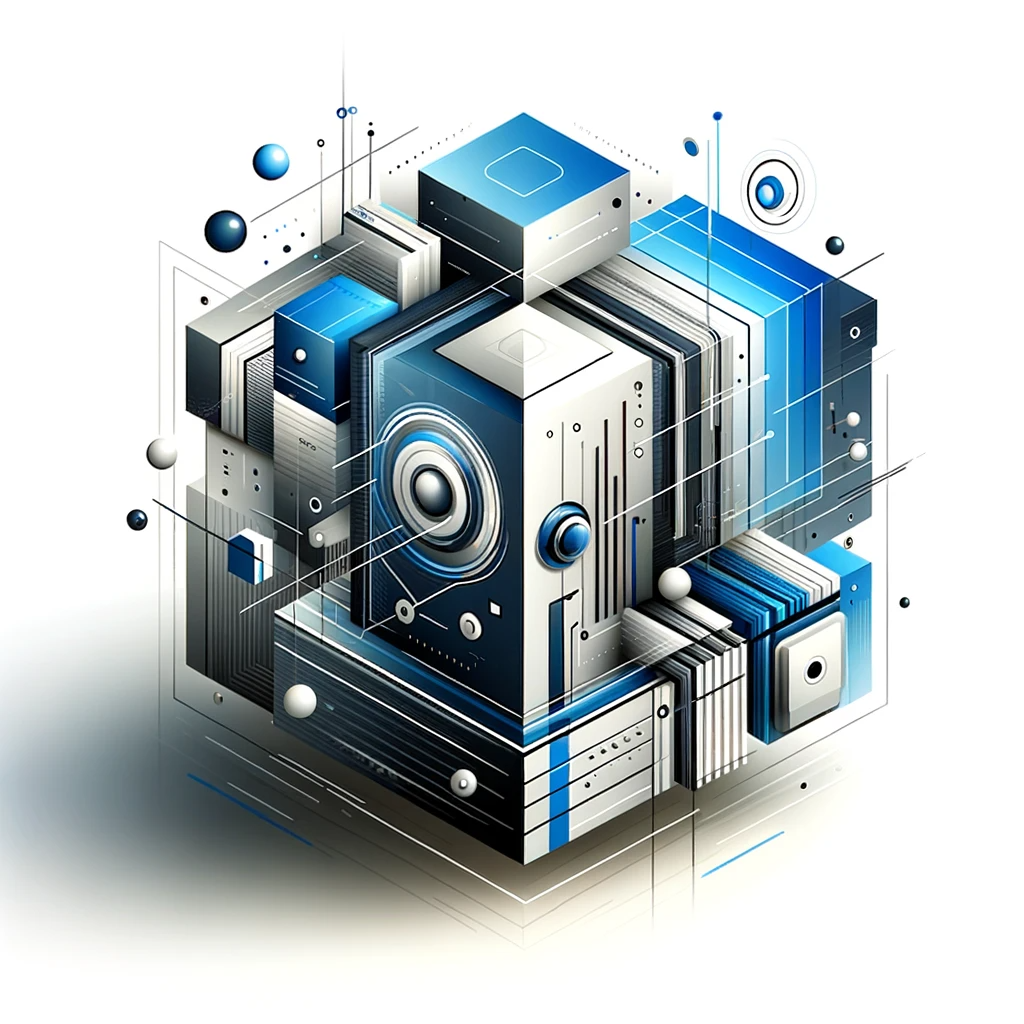
Abstract
With the current technological advancements, particularly in sensing technologies, monitoring various health aspects, including heart rate, has become feasible. The problem addressed in this study is the need for effective stress detection methods to mitigate the significant consequences of high-intensity or long-term stress, which impacts safety and disrupts normal routines. We propose a stress detection system developed based on the convolutional neural network (CNN) method to address this. The study involves university students aged 20–22, focusing on mental stress. The dataset encompasses parameters such as heart rate, footsteps, and resting heart rate recorded through a smartwatch with 149,797-row data. Our results indicate that the CNN model achieves an 84.5% accuracy, 80.9% precision, 79.8% recall, and an 80.4% F1-score, confirming its efficacy in stress classification. The confusion matrix further validates the model’s accuracy, particularly for classes 1 and 2. This research contributes significantly to the development of an effective and practical stress detection method, holding promise for enhancing well-being and preventing stress-related health issues.
Discover Our Library
Embark on a journey through our expansive collection of articles and let curiosity lead your path to innovation.
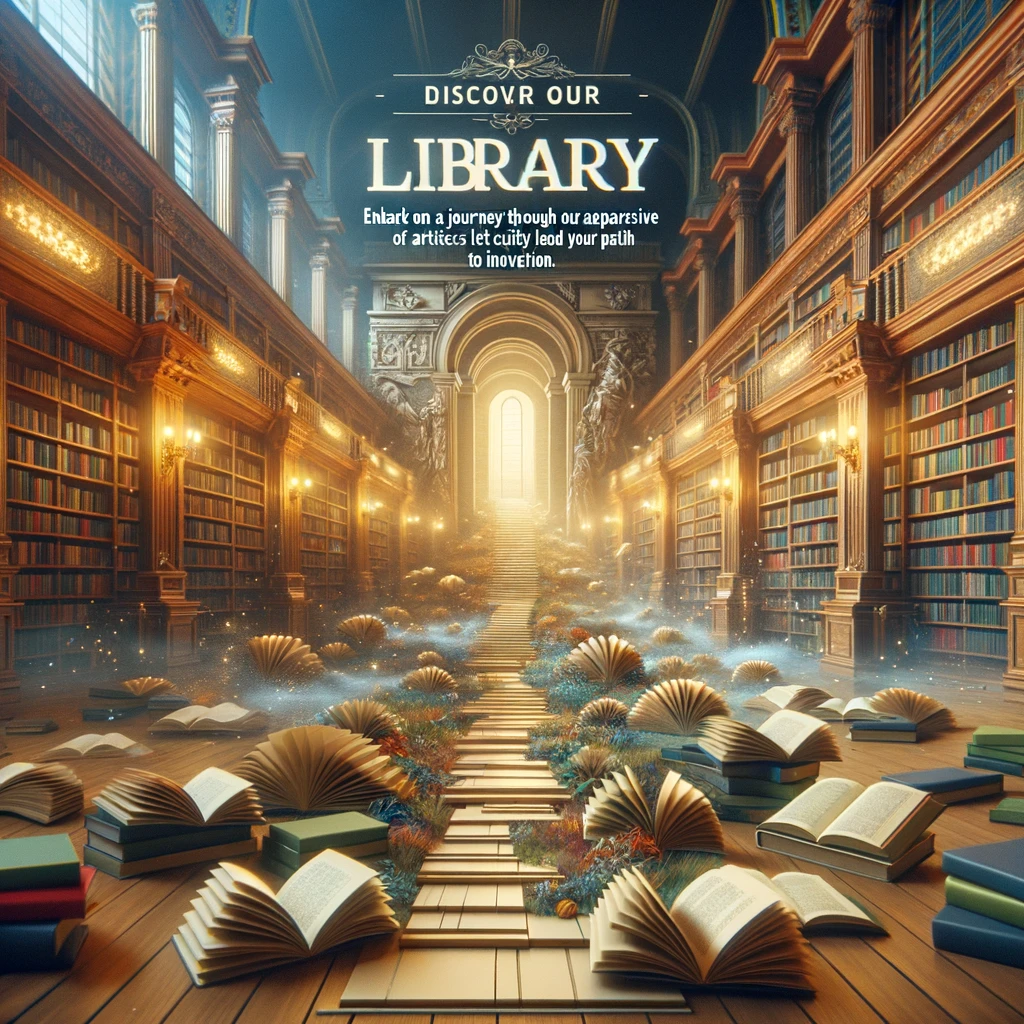