A novel technique for selecting financial parameters and technical indicators to predict stock prices
International Journal of Electrical and Computer Engineering
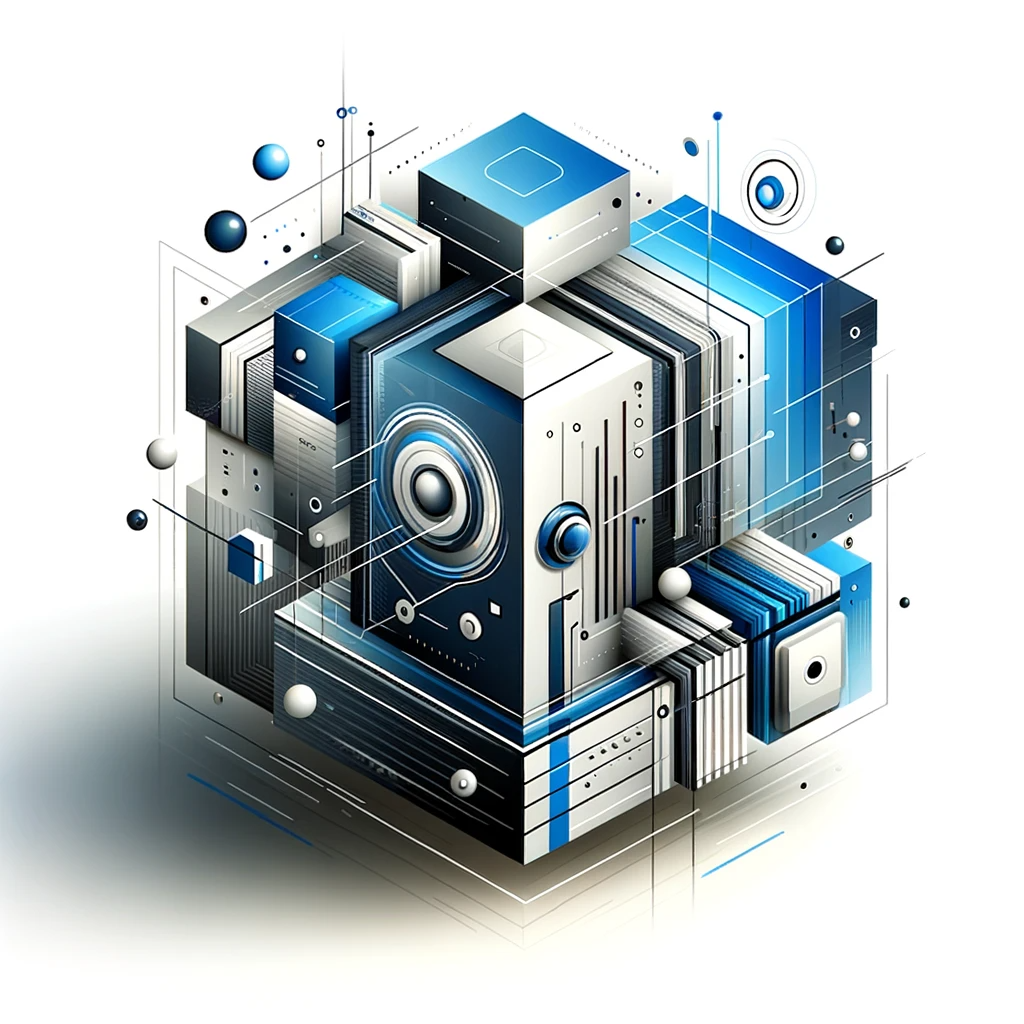
Abstract
Stock price predictions are crucial in financial markets due to their inherent volatility. Investors aim to forecast stock prices to maximize returns, but accurate predictions are challenging due to frequent price fluctuations. Most literature focuses on technical indicators, which rely on historical data. This study integrates both financial parameters and technical indicators to predict stock prices. It involves three main steps: identifying essential financial parameters using recursive feature elimination (RFE), selecting quality stocks with a decision tree (DT), and forecasting stock prices using artificial neural networks (ANN), deep neural networks (DNN), and extreme gradient boosting (XGBoost). The models’ performance is evaluated with root mean square error (RMSE) and mean absolute error (MAE) scores. ANN and DNN models showed superior performance compared to the XGBoost model. The experiments utilized Indian stock data.
Discover Our Library
Embark on a journey through our expansive collection of articles and let curiosity lead your path to innovation.
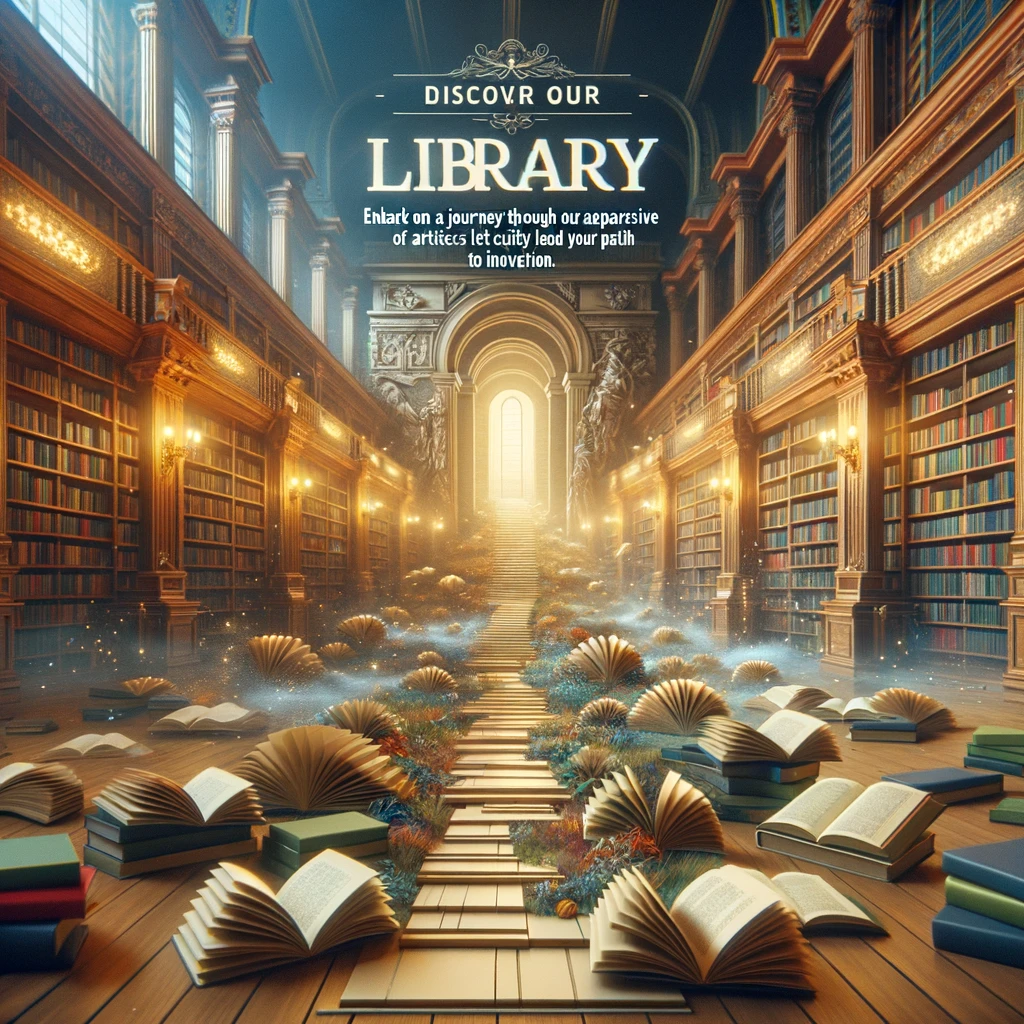