Enhanced automated Alzheimer’s disease detection from MRI images by exploring handcrafted and transfer learning feature extraction methods
International Journal of Electrical and Computer Engineering
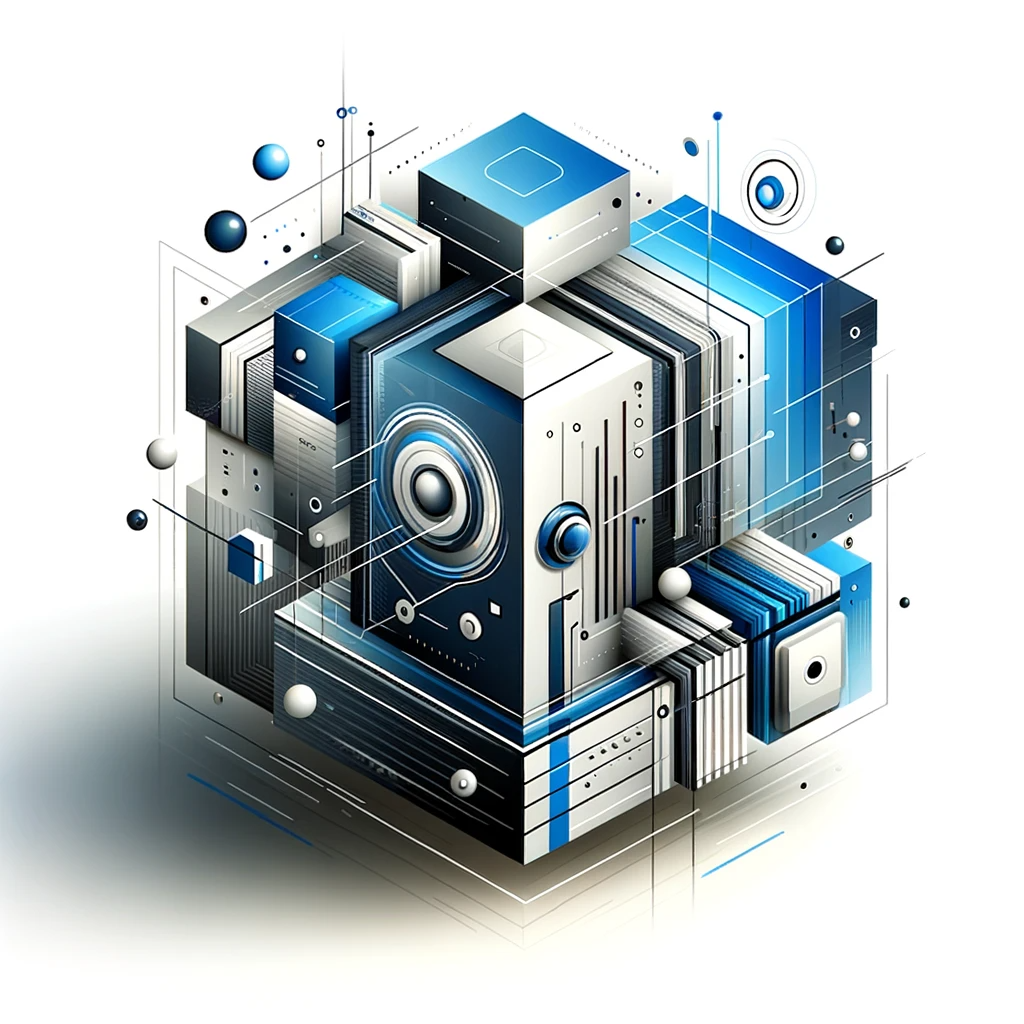
Abstract
The rising prevalence of Alzheimer’s disease (AD) poses a significant global health challenge. Early detection of AD enables appropriate and timely treatment to slow disease progression. In this paper, we propose an enhanced procedure for automated AD detection from magnetic resonance imaging (MRI) images, focusing on two primary tasks: feature extraction and classification. For feature extraction, we have investigated two categories of methods: handcrafted techniques and those based on pre-trained convolutional neural network (CNN) models. Handcrafted methods are preceded by a preprocessing step to improve the MRI image contrast, while the pre-trained CNN models were adapted by utilizing only a part of the models as feature extractors, incorporating a global average pooling (GAP) layer to flatten the feature vector and reduce its dimensionality. For classification, we employed three different algorithms as binary classifiers to detect AD from MRI images. Our results demonstrate that the support vector machine (SVM) classifier achieves a classification accuracy of 99.92% with Gabor features and 100% with ResNet101 CNN features, competing with existing methods. This study underscores the effectiveness of feature extraction using Gabor filters, as well as those based on the adapted pre-trained CNN models, for accurate AD detection from MRI images, offering significant advancements in early diagnosis.
Discover Our Library
Embark on a journey through our expansive collection of articles and let curiosity lead your path to innovation.
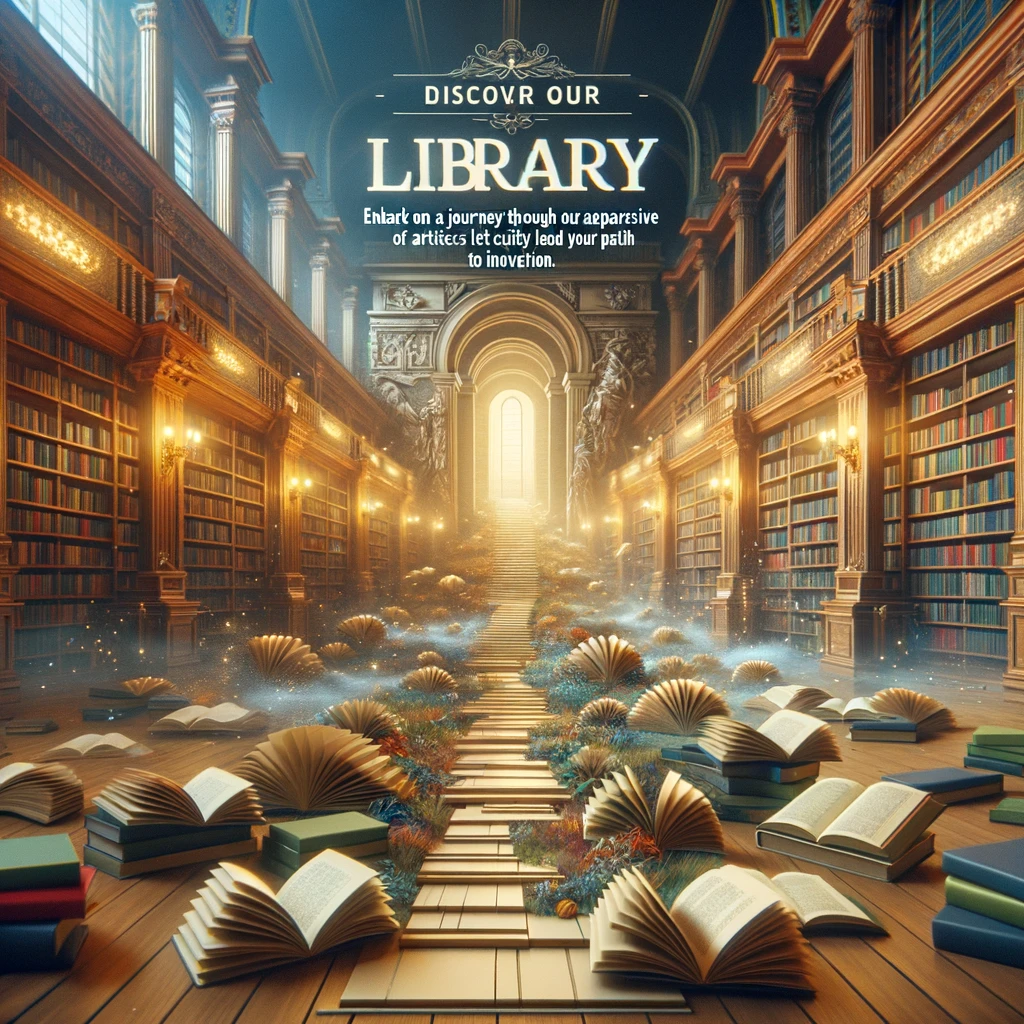