Customized dataset-based machine learning approach for black hole attack detection in mobile ad hoc networks
International Journal of Electrical and Computer Engineering
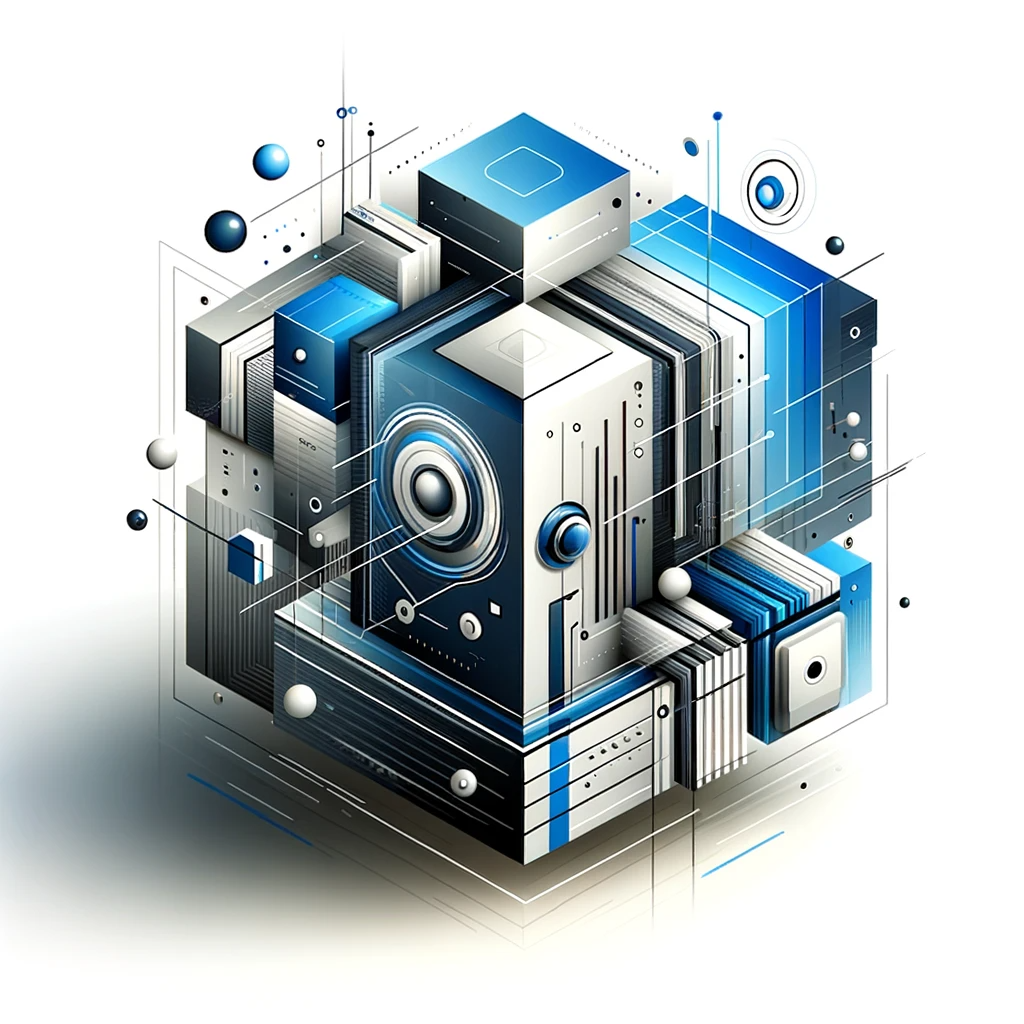
Abstract
This article explores the application of machine learning (ML) algorithms to classify the black hole attack in mobile ad hoc networks (MANETs). Black hole attacks threaten MANETs by disrupting communication and data transmission. The primary goal of this study is to develop an intrusion detection system (IDS) to detect and classify this attack. The research process involves feature selection, the creation of a custom dataset tailored to the characteristics of black hole attacks, and the evaluation of four machine learning models: random forest (RF), logistic regression (LR), k-nearest neighbors (k-NN), and decision tree (DT). The evaluation of these models demonstrates promising results, with significant improvements in accuracy, precision, F1-score, and recall metrics. The findings underscore the potential of machine learning in enhancing the security of MANETs by providing an effective means of attack classification.
Discover Our Library
Embark on a journey through our expansive collection of articles and let curiosity lead your path to innovation.
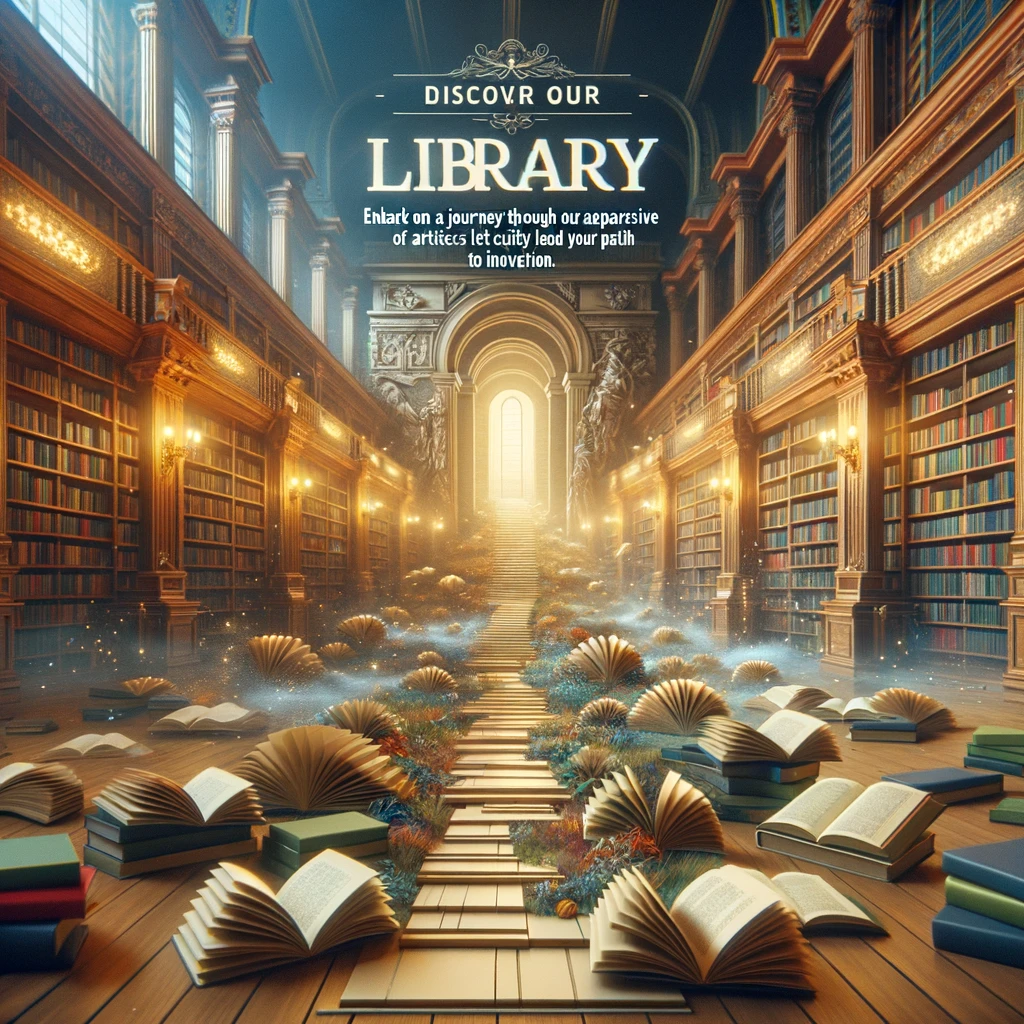