User behavior analysis for insider attack detection using a combination of memory prediction model and recursive feature elimination algorithm
International Journal of Electrical and Computer Engineering
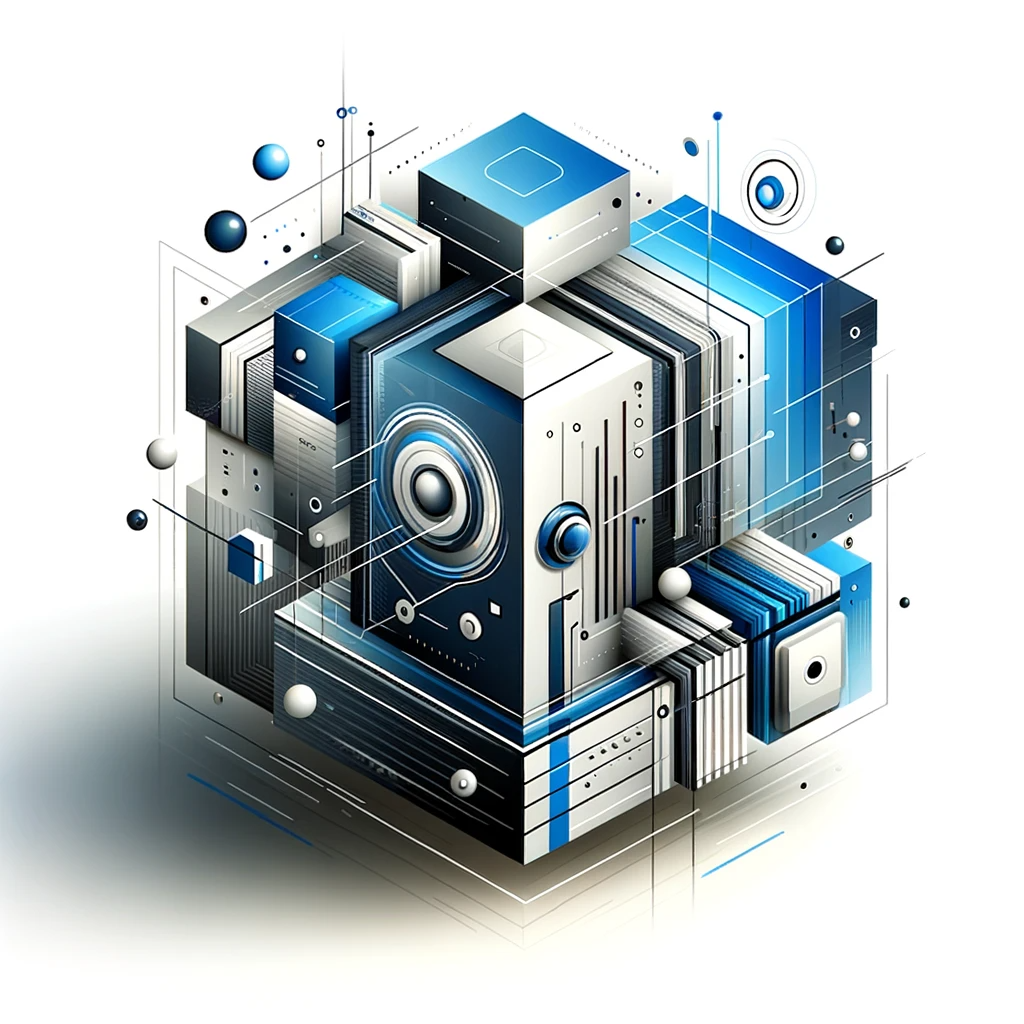
Abstract
Existing defense tools against the insider attacks are rare, not in real time fashion and suffer from low detection accuracy as the attacks become more sophisticated. Thus, a detection tool with online learning ability and better accuracy is required urgently. This study proposes an insider attack detection model by leveraging entity behavior analysis technique based on a memory prediction model combined with the recursive feature elimination (RFE) feature selection algorithm. The memory-prediction model provides ability to perform online learning, while the RFE algorithm is deployed to reduce data dimensionality. Dataset for the experiment was created from a real network with 150 active users, and mixed with attacks data from publicly available dataset. The dataset is simulated on a testbed network environment consisting of a server configured to run 4 virtual servers and other two computers as traffic generator and detection tool. The experimental results show 94.01% of detection accuracy, 95.64% of precision, 99.28% of sensitivity, and 96.08% of F1-score. The proposed model is able to perform on-the-fly learning to address evolving nature of the attacks. Combining memory prediction models with the RFE for user behavior analysis is a promising approach, and achieving high accuracy is definitely a positive outcome.
Discover Our Library
Embark on a journey through our expansive collection of articles and let curiosity lead your path to innovation.
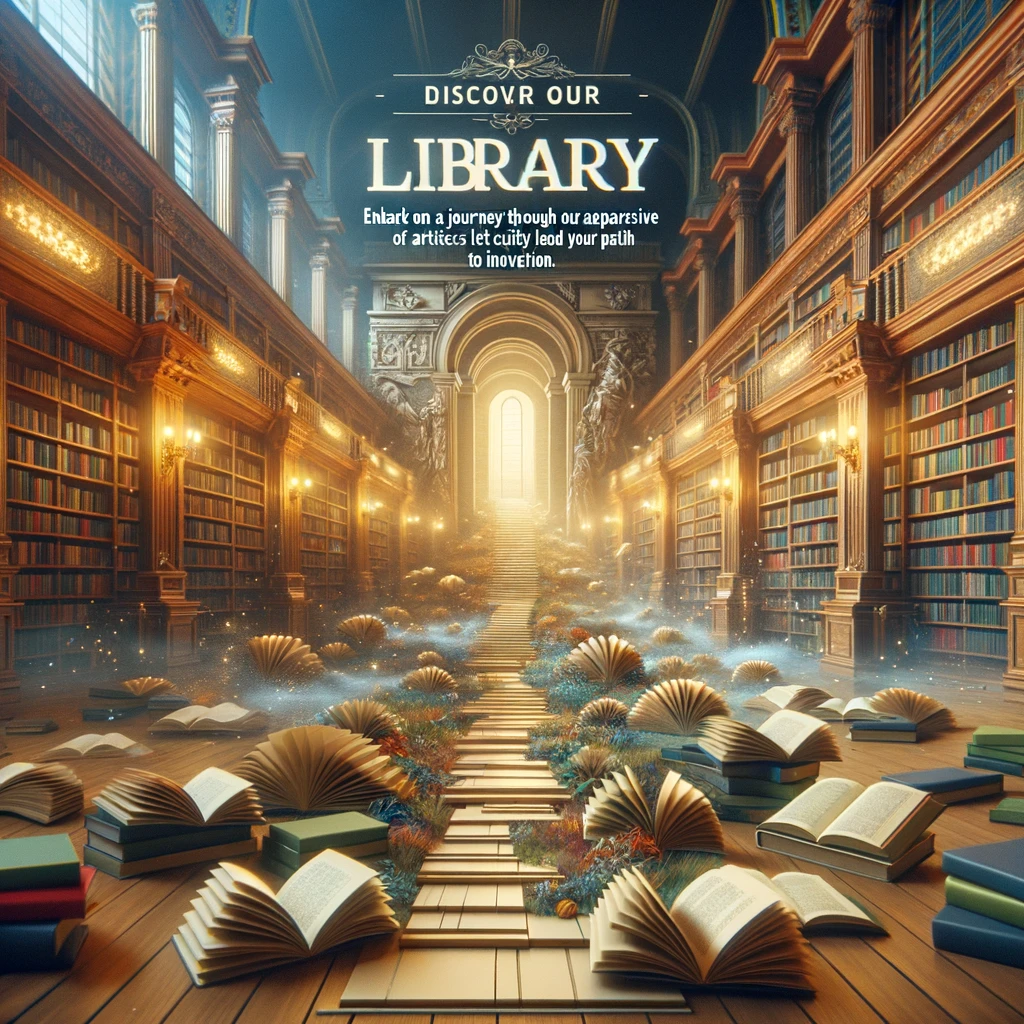