Trust factor validation for distributed denial of service attack detection using machine learning
International Journal of Electrical and Computer Engineering
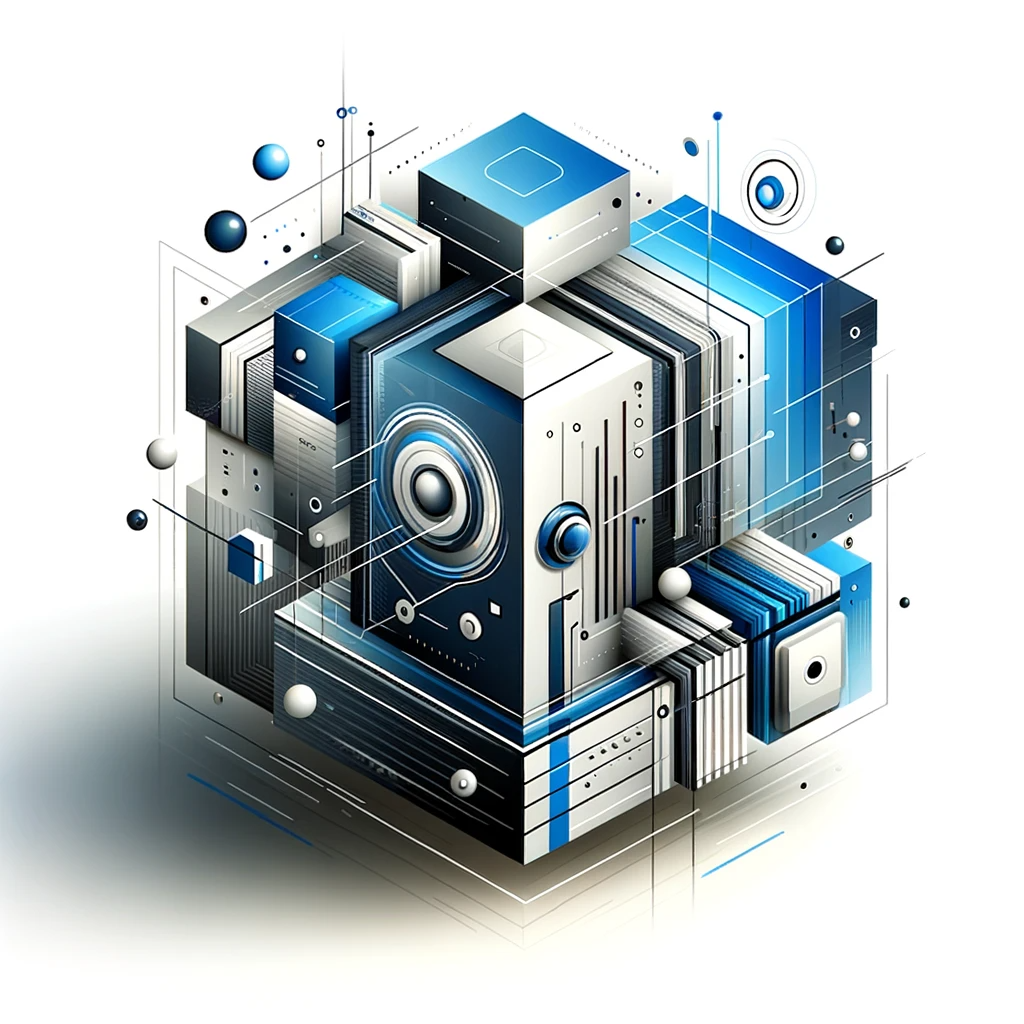
Abstract
Distributed denial of service (DDoS) attacks, predicted to be 100 Gbps and greater, are expected to begin in the first quarter of 2019, with 77% of all attacks concentrated on at least two vectors. According to a Neustar Research Agency assessment, DDoS attacks are becoming more powerful and common. Among many other issues, distributed denial of service is a notable security issue. A large number of research projects have been conducted to address this issue, but their methodologies are either inaccurate or computationally expensive, making developing an effective DDoS assault detection method a critical demand of current research. A DDoS attack employs a huge number of agents or resources to carry out the attack, resulting in a large-scale attack power. The attackers use their intelligence to discover the weak system, which is then coordinated and managed remotely. The suggested detection framework uses a frequent time interval balancing module with node trust factor validation (FTIBM-NTFV) that is used to identify the DDoS attacks in the system for improving the security levels of the network. The proposed model is compared with the traditional methods and the results are analyzed that represents the proposed model is achieving better outcomes.
Discover Our Library
Embark on a journey through our expansive collection of articles and let curiosity lead your path to innovation.
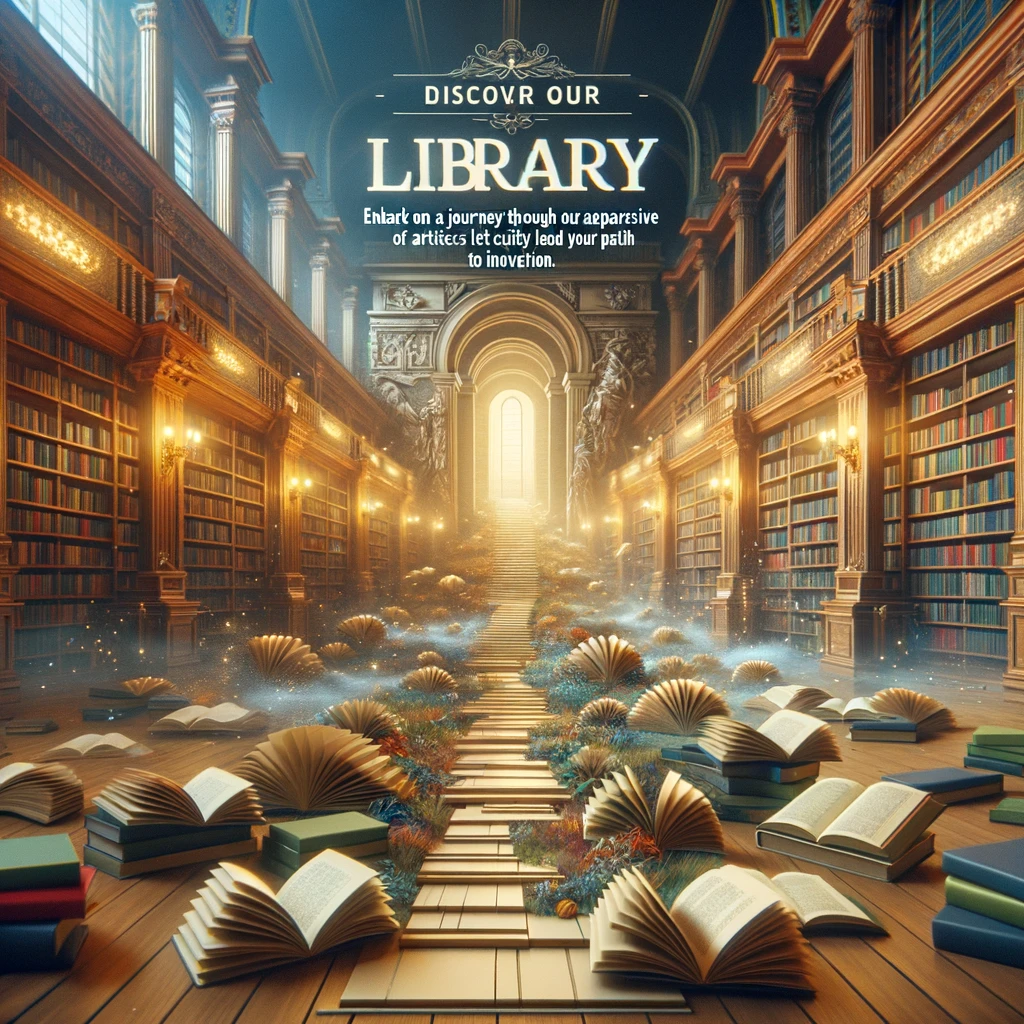