Revisiting self-supervised contrastive learning for imbalanced classification
International Journal of Electrical and Computer Engineering
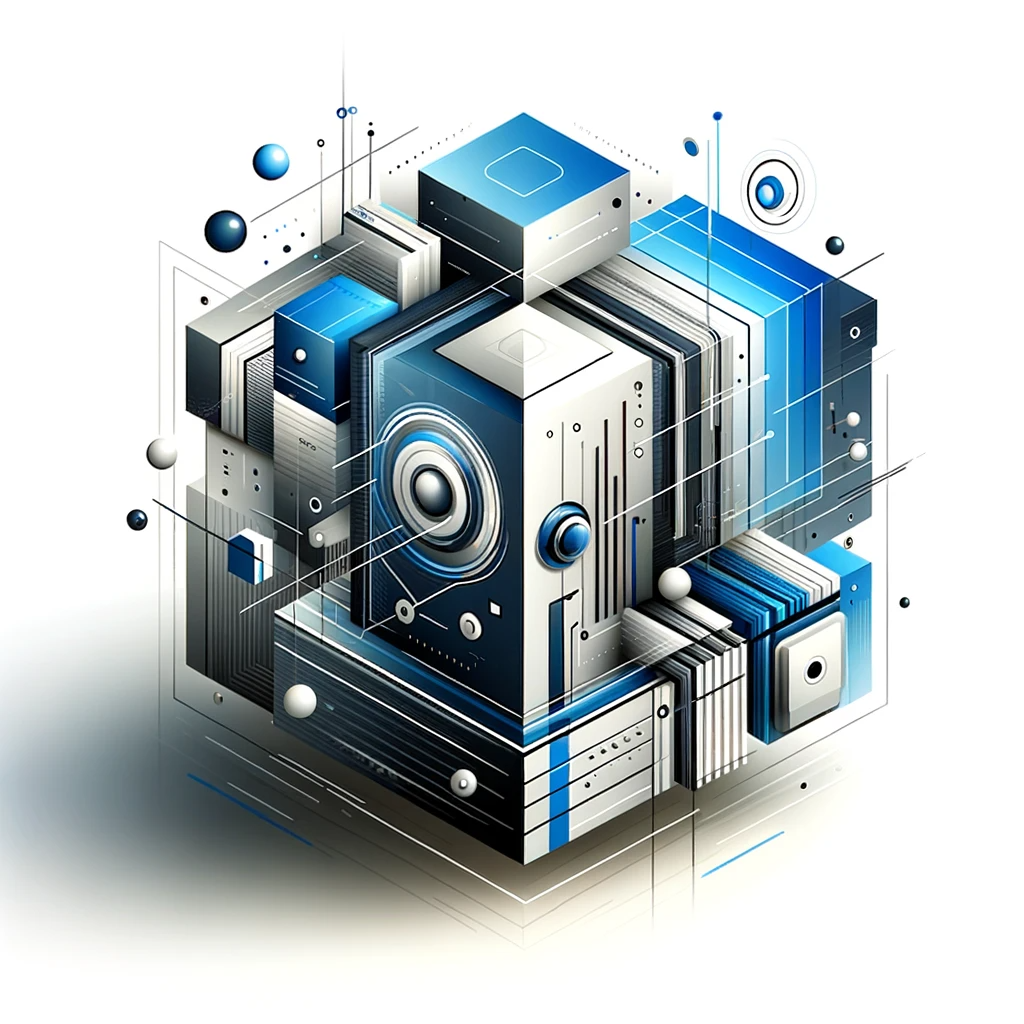
Abstract
Class imbalance remains a formidable challenge in machine learning, particularly affecting fields that depend on accurate classification across skewed datasets, such as medical imaging and software defect prediction. Traditional approaches often fail to adequately address the underrepresentation of minority classes, leading to models that exhibit high performance on majority classes but have poor performance on critical minority classes. Self-supervised contrastive learning has become an extremely encouraging method for this issue, enabling the utilization of unlabeled data to generate robust and generalizable models. This paper reviews the advancements in self-supervised contrastive learning for imbalanced classification, focusing on methodologies that enhance model performance through innovative contrastive loss functions and data augmentation strategies. By pulling similar instances closer and pushing dissimilar ones apart, these techniques help mitigate the biases inherent in imbalanced datasets. We critically analyze the effectiveness of these methods in diverse scenarios and propose future research directions aimed at refining these approaches for broader application in real-world settings. This review serves as a guide for researchers exploring the potential of contrastive learning to address class imbalances, highlighting recent successes and identifying crucial gaps that need addressing.
Discover Our Library
Embark on a journey through our expansive collection of articles and let curiosity lead your path to innovation.
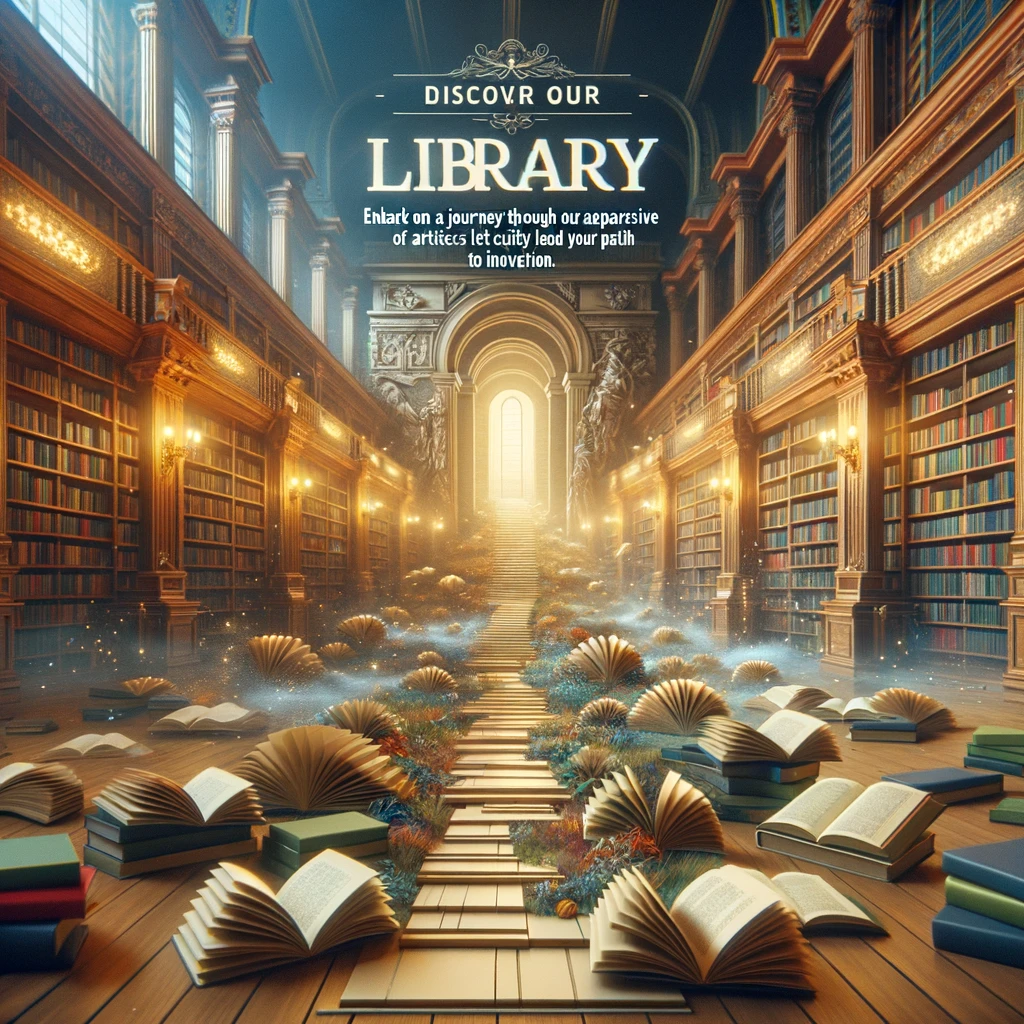