Tree-based models and hyperparameter optimization for assessing employee performance
Indonesian Journal of Electrical Engineering and Computer Science
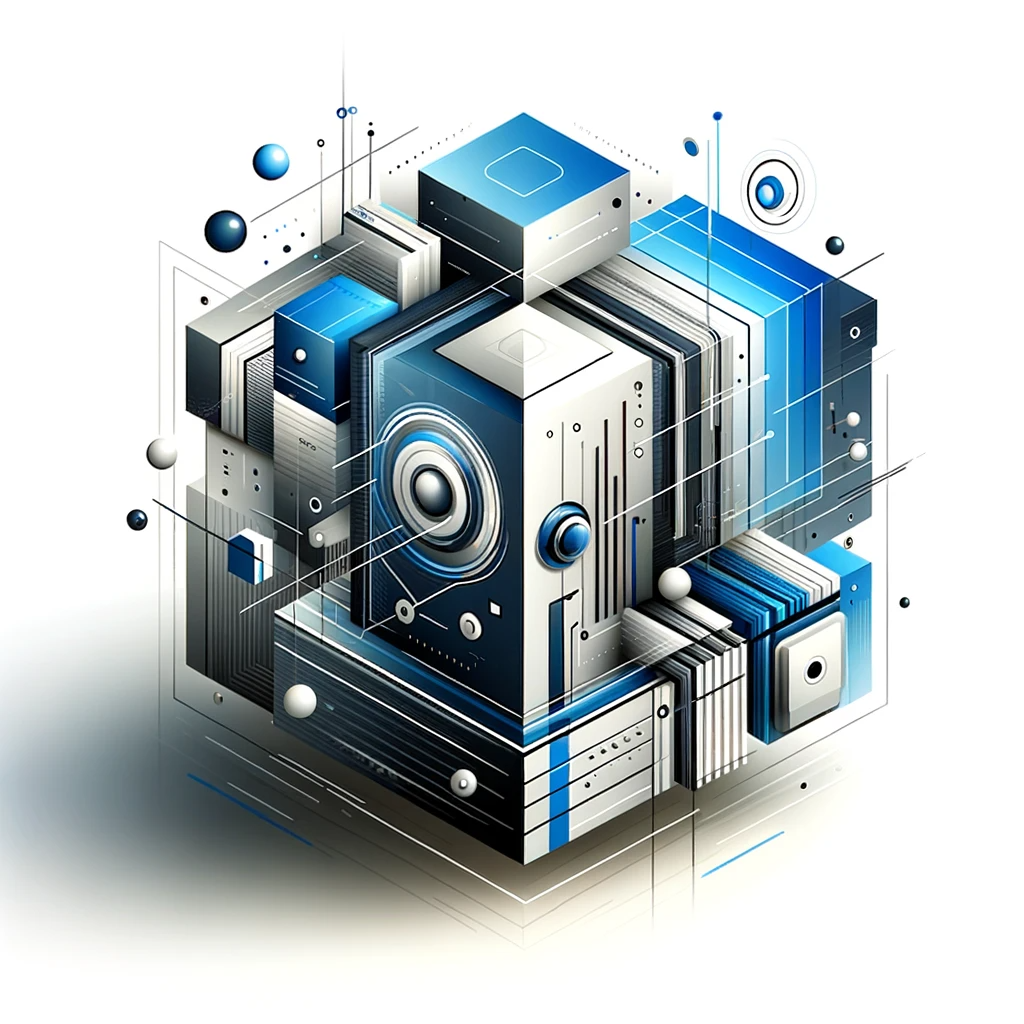
Abstract
The Palembang city fire and rescue service (FRS) is encountering challenges in adhering to national standards for fire response time. Hence, the Palembang city FRS is committed to enhancing employee performance through quarterly performance assessments based on various criteria such as attendance, work targets, behavior, education, and performance reports. This study proposes tree-based models in machine learning (ML) and hyperparameter optimization to assess the performance of Palembang city FRS employees. Tree-based models encompass decision trees (DT), random forests (RF), and extreme gradient boosting (XGB). The predictive performance of each model was evaluated using the confusion matrix (CM), the area under the receiver operating characteristic (AUROC), and the kappa coefficient (KC). The results indicate that RF performs better than DT and XGB in the sensitivity, AUROC, and KC metrics by 1.0000, 0.9874, and 0.8584, respectively.
Discover Our Library
Embark on a journey through our expansive collection of articles and let curiosity lead your path to innovation.
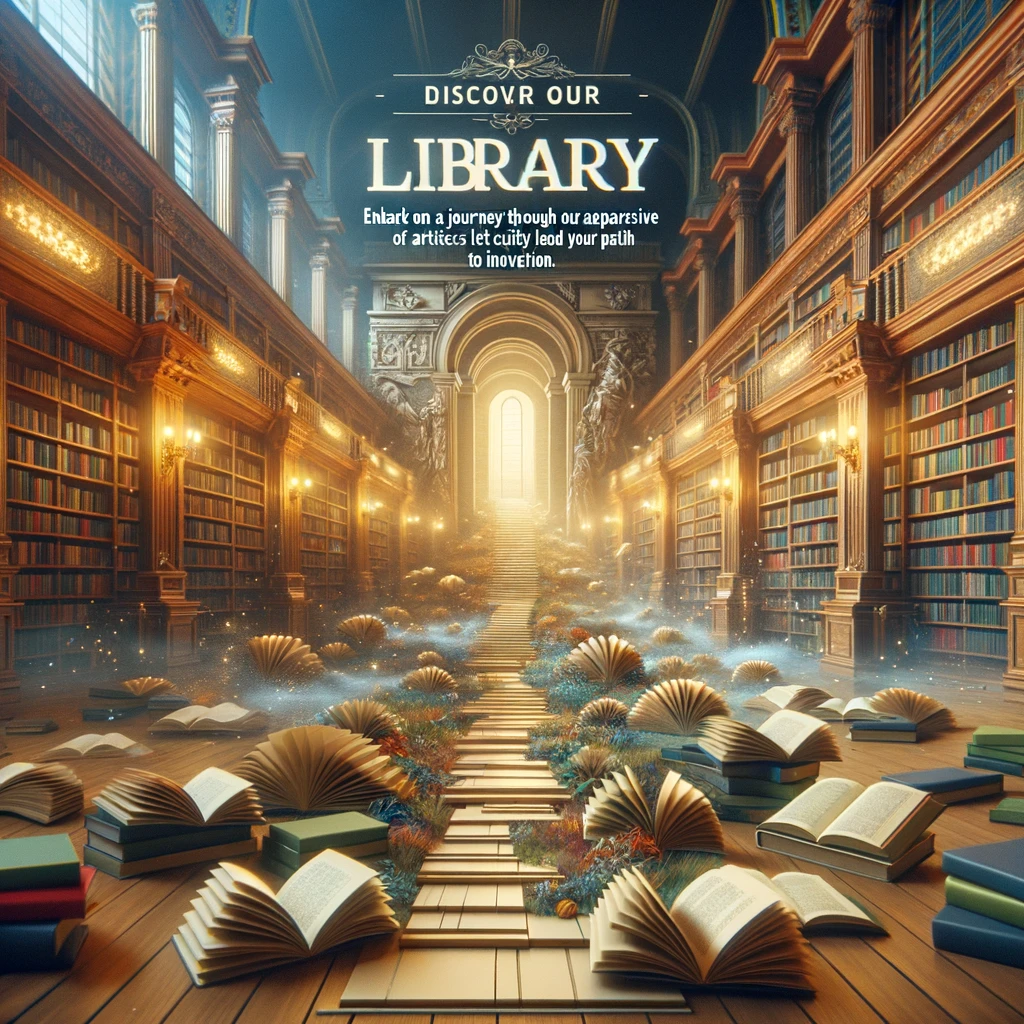