IDCCD: evaluation of deep learning for early detection caries based on ICDAS
Indonesian Journal of Electrical Engineering and Computer Science
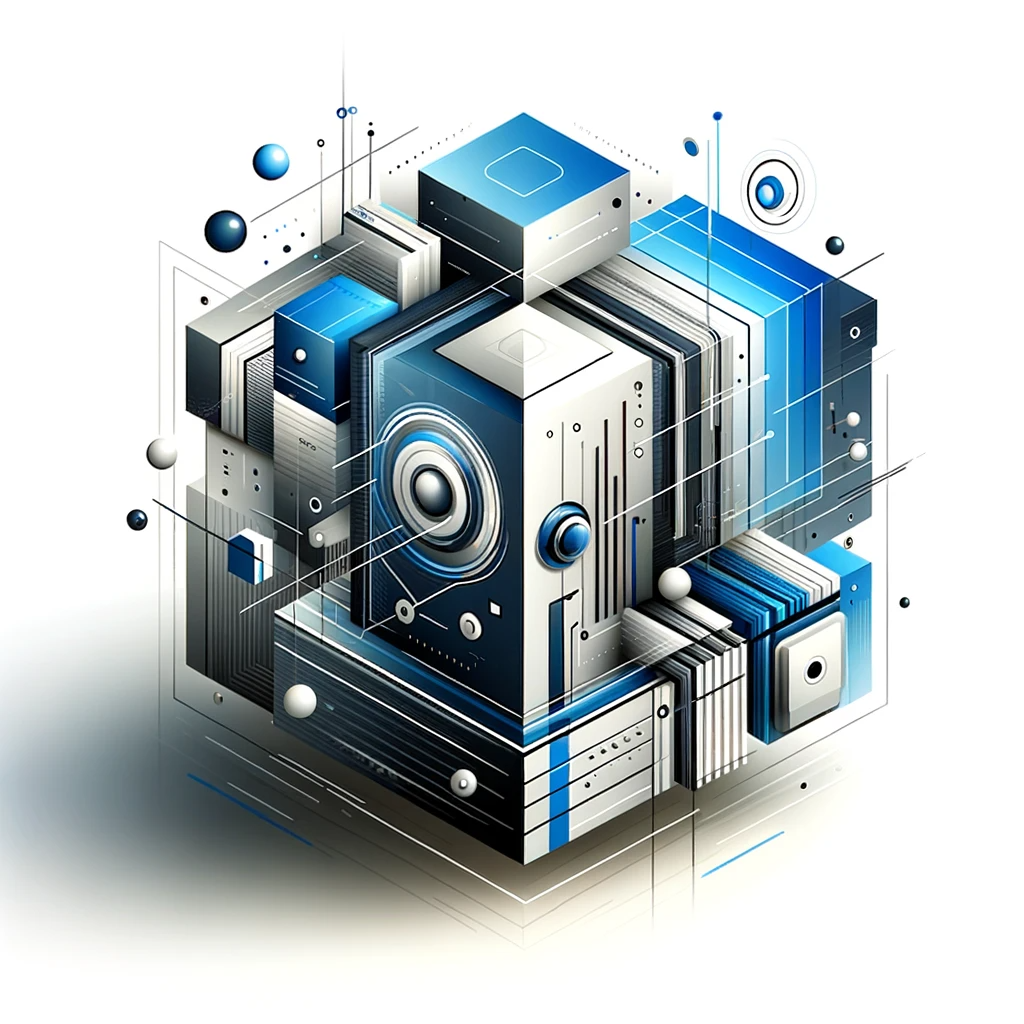
Abstract
Dental caries is a common oral disease in children, influenced by environmental, psychological, behavioral, and biological factors. The American academy of pediatric dentistry recommends screening from the time the first tooth erupts or at one year of age to prevent caries, which mostly affects children from racial and ethnic minorities. In Indonesia, the 2023 health survey reported a caries prevalence of 84.8% in children aged 5-9 years. This research introduces early caries detection using three deep learning models: faster-RCNN, you only look once (YOLO) V8, and detection transformer (DETR), using Indonesian dental caries characteristic datasets (IDCCD) focused on Indonesian data with international caries detection and assessment system (ICDAS) classification D0 to D6. The results showed that YOLO V8-s and DETR gave good results, with mean average precision (mAP) of 41.8% and 41.3% for intersection over union (IoU) 50, and 24.3% and 26.2% for IoU 50:90. Precision-recall (PR) curves show that both models have high precision at low recall (0 to 0.2), but precision decreases sharply as recall increases. YOLO V8-s showed a slower and more regular decrease in precision, indicating a more stable performance compared to DETR.
Discover Our Library
Embark on a journey through our expansive collection of articles and let curiosity lead your path to innovation.
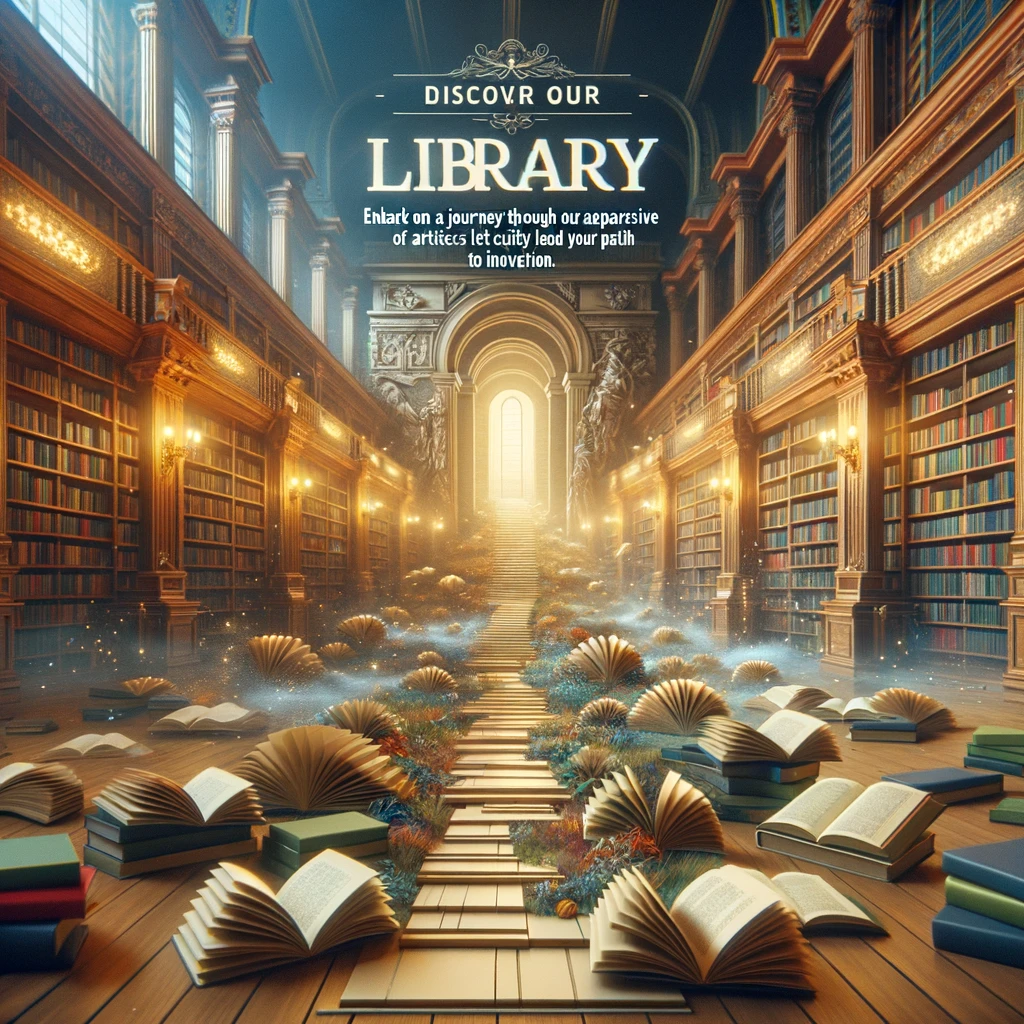