A multi-scale convolutional neural network and discrete wavelet transform based retinal image compression
Indonesian Journal of Electrical Engineering and Computer Science
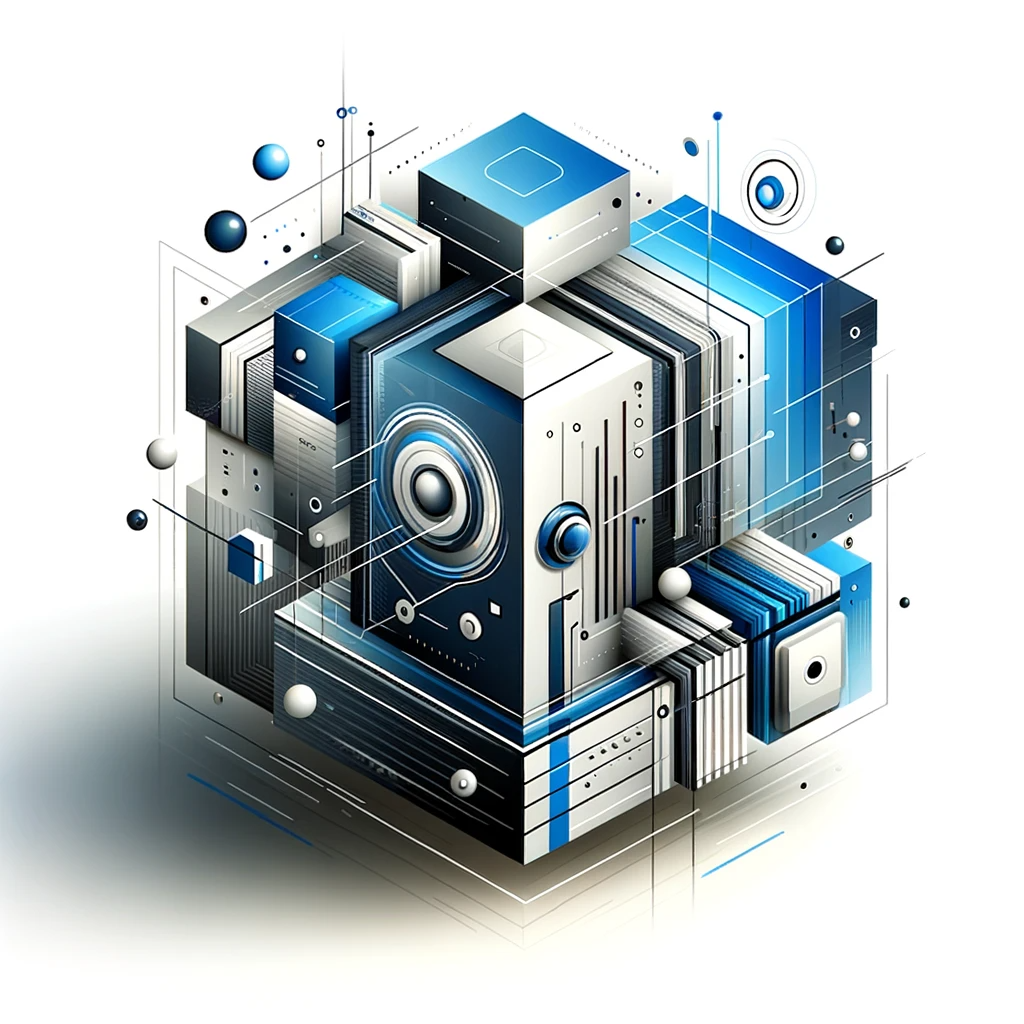
Abstract
The different applications of medical images have contributed significantly to the growing amount of image data. As a result, compression techniques become essential to allow real-time transmission and storage within limited network bandwidth and storage space. Deep learning, particularly convolutional neural networks (CNN) have marked rapid advances in many computer vision tasks and have progressively drawn attention for being used in image compression. Therefore, we present a method for compressing retinal images based on deep CNN and discrete wavelet transform (DWT). To further enhance CNN capabilities, multi-scale convolutions are introduced into the network architecture. In this proposed method, multiscale CNNs are used to extract useful features to provide a compact representation at the encoding stage and guarantee a better reconstruction quality of the image at the decoding stage. Based on compression efficiency and reconstructed image quality, a wide range of experiments have been conducted to validate the proposed technique performance compared with popular image compression standards and existing deep learning-based methods. At a compression ratio (CR) of 80, the proposed method achieved an average peak signal-to-noise ratio (PSNR) value of 38.98 dB and 96.8% similarity in terms of multi-scale structural similarity (MS-SSIM), demonstrating its effectiveness.
Discover Our Library
Embark on a journey through our expansive collection of articles and let curiosity lead your path to innovation.
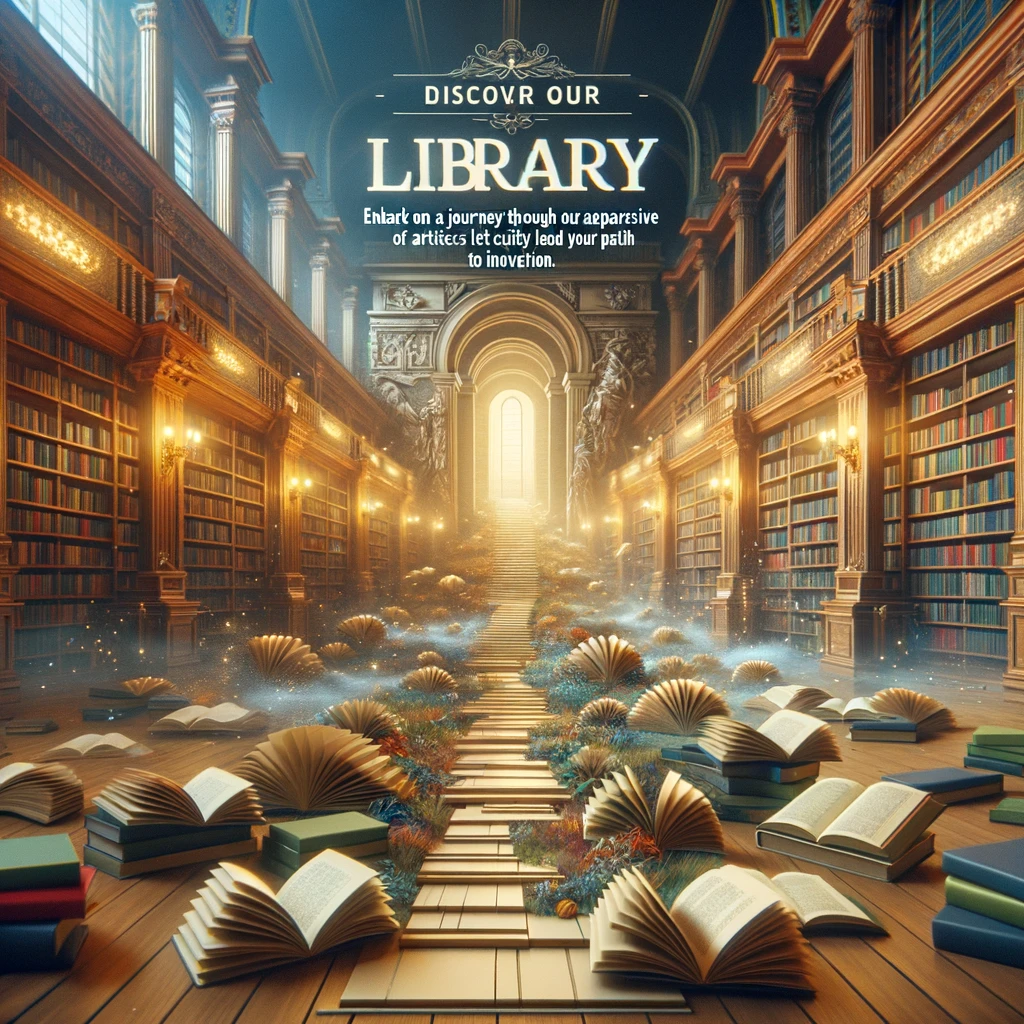