SMOTE tree-based autoencoder multi-stage detection for man-in-the-middle in SCADA
Indonesian Journal of Electrical Engineering and Computer Science
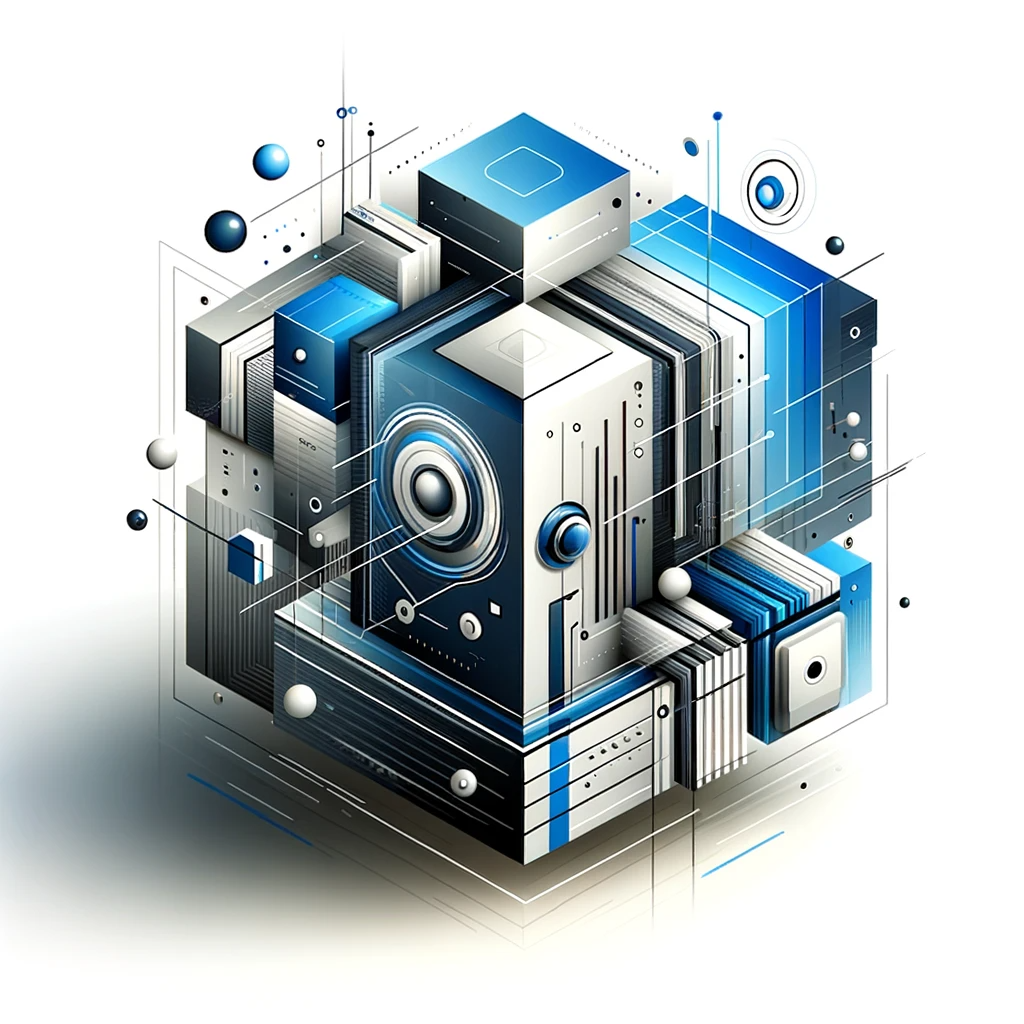
Abstract
Security incidents targeting supervisory control and data acquisition (SCADA) infrastructure are increasing, which can lead to disasters such as pipeline fires or even lost of lives. Man-in-the-middle (MITM) attacks represent a significant threat to the security and reliability of SCADA. Detecting MITM attacks on the Modbus SCADA networks is the objective of this work. In addition, this work introduces SMOTE tree-based autoencoder multi-stage detection (STAM) using the Electra dataset. This work proposes a four-stage approach involving data preprocessing, data balancing, an autoencoder, and tree classification for anomaly detection and multi-class classification. In terms of attack identification, the proposed model performs with highest precision, detection rate/recall, and F1 score. In particular, the model achieves an F1 score of 100% for anomaly detection and an F1 score of 99.37% for multi-class classification, which is preeminence to other models. Moreover, the enhanced performance of multi-class classification with STAM on minority attack classes (replay and read) has shown similar characteristics in features and a reduced number of misclassifications in these classes.
Discover Our Library
Embark on a journey through our expansive collection of articles and let curiosity lead your path to innovation.
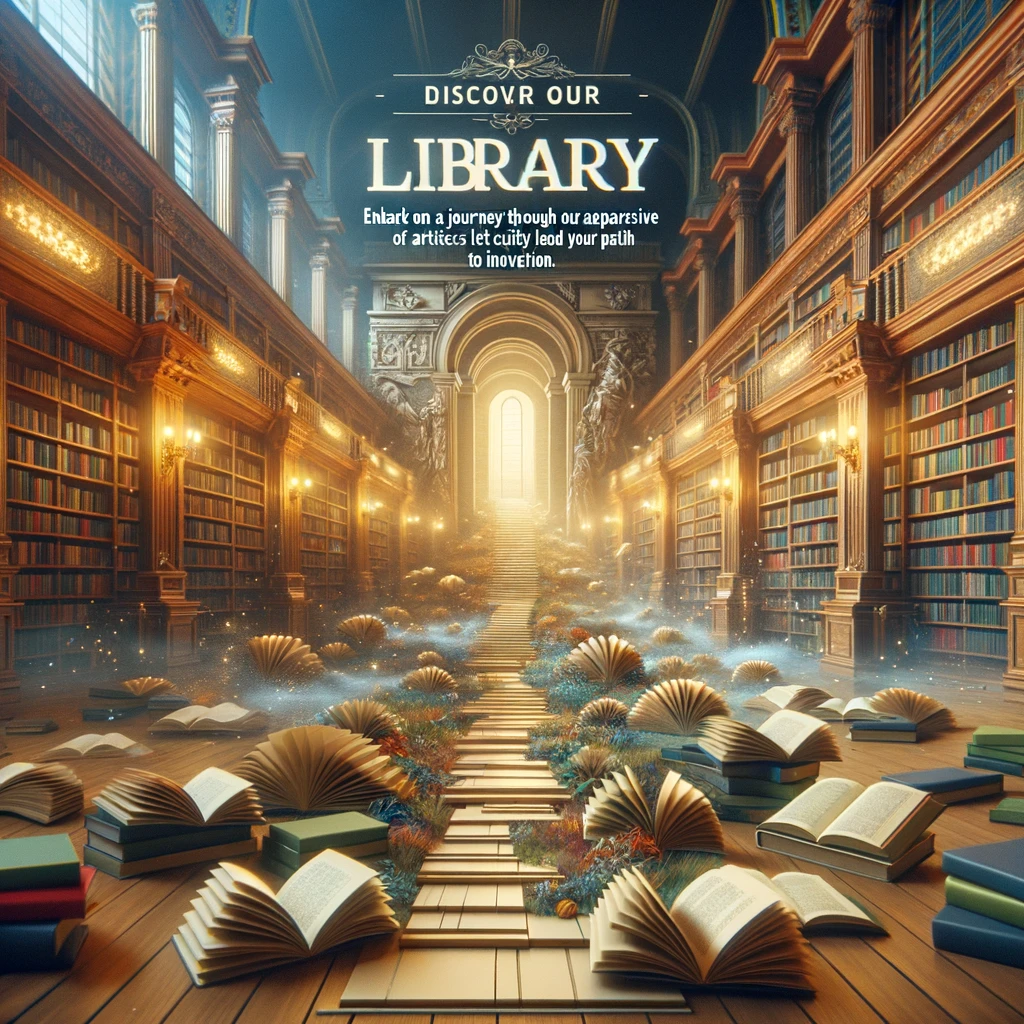