Boosting stroke prediction with ensemble learning on imbalanced healthcare data
Indonesian Journal of Electrical Engineering and Computer Science
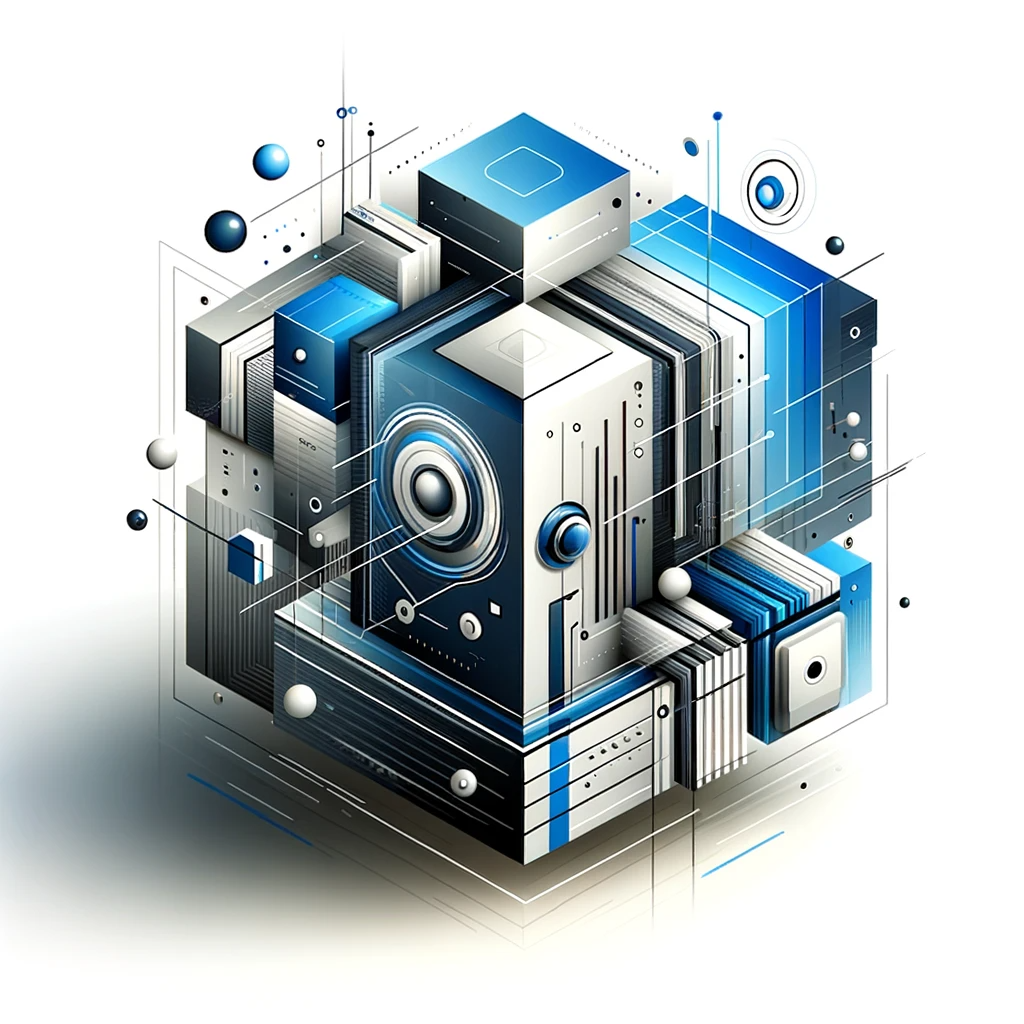
Abstract
Detecting strokes at the early day is crucial for preventing health issues and potentially saving lives. Predicting strokes accurately can be challenging, especially when working with unbalanced healthcare datasets. In this article, we suggest a thorough method combining machine learning (ML) algorithms and ensemble learning techniques to improve the accuracy of predicting strokes. Our approach includes using preprocessing methods for tackling imbalanced data, feature engineering for extracting key information, and utilizing different ML algorithms such as random forests (RF), decision trees (DT), and gradient boosting (GBoost) classifiers. Through the utilization of ensemble learning, we amalgamate the advantages of various models in order to generate stronger and more reliable predictions. By conducting thorough tests and assessments on a variety of datasets, we demonstrate the efficacy of our approach in addressing the imbalanced stroke datasets and greatly enhances prediction accuracy. We conducted comprehensive testing and validation to ensure the reliability and applicability of our method, improving the accuracy of stroke prediction and supporting healthcare planning and resource allocation strategies.
Discover Our Library
Embark on a journey through our expansive collection of articles and let curiosity lead your path to innovation.
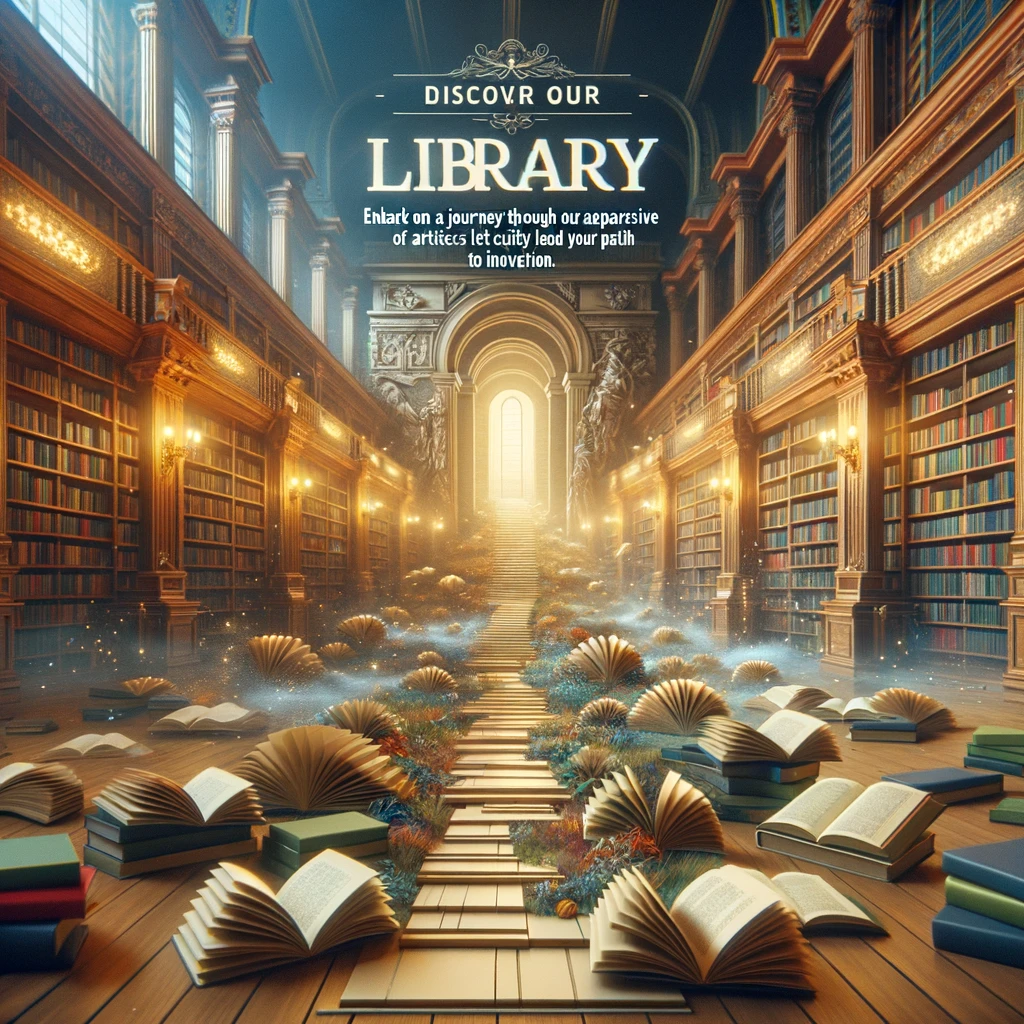