A deep learning approach to detect DDoS flooding attacks on SDN controller
Indonesian Journal of Electrical Engineering and Computer Science
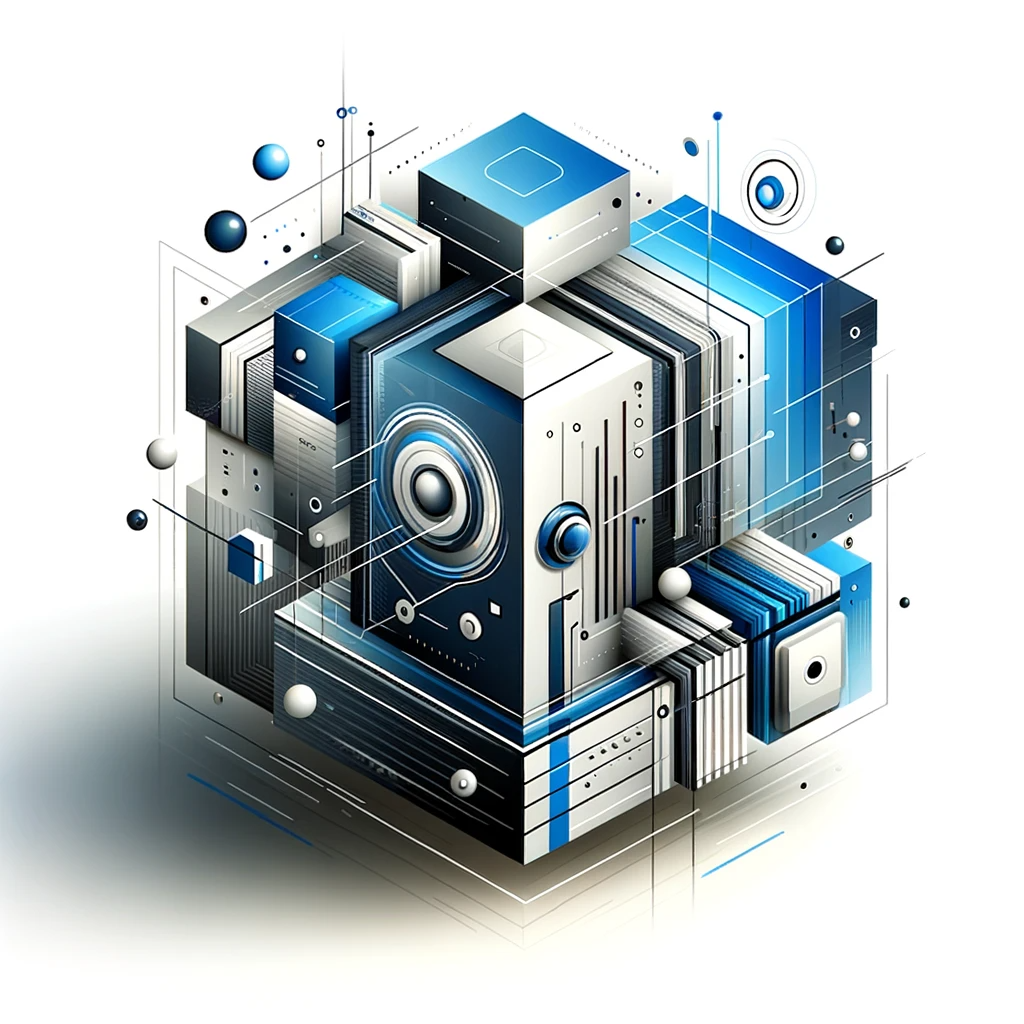
Abstract
Software-defined networking (SDN), integrated into technologies like internet of things (IoT), cloud computing, and big data, is a key component of the fourth industrial revolution. However, its deployment introduces security challenges that can undermine its effectiveness. This highlights the urgent need for security-focused SDN solutions, driving advancements in SDN technology. The absence of inherent security countermeasures in the SDN controller makes it vulnerable to distributed denial of service (DDoS) attacks, which pose a significant and pervasive threat. These attacks specifically target the controller, disrupting services for legitimate users and depleting its resources, including bandwidth, memory, and processing power. This research aims to develop an effective deep learning (DL) approach to detect such attacks, ensuring the availability, integrity, and consistency of SDN network functions. The proposed DL detection approach achieves 98.068% accuracy, 98.085% precision, 98.067% recall, 98.057% F1-score, 1.34% false positive rate (FPR), and 1.713% detection time.
Discover Our Library
Embark on a journey through our expansive collection of articles and let curiosity lead your path to innovation.
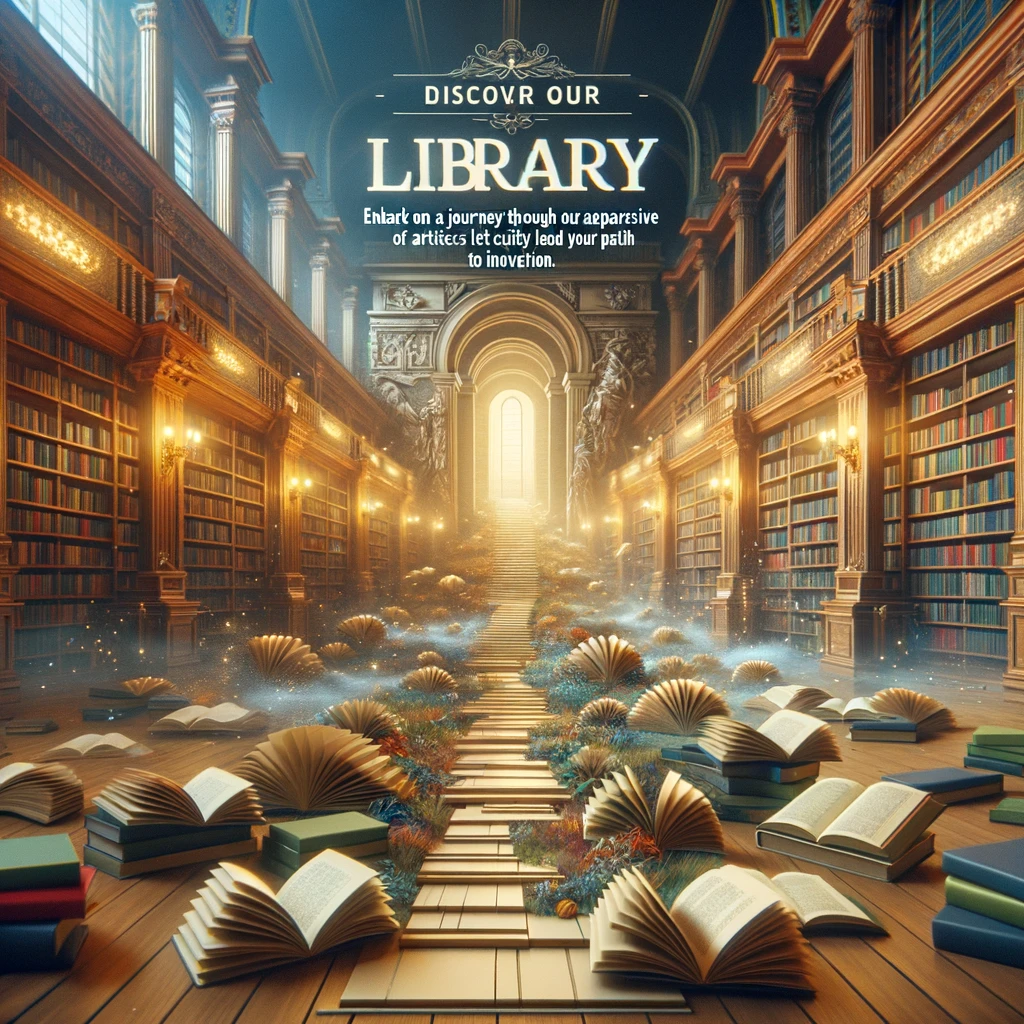