A hybrid learning model to detect cardiovascular disease from electrocardiogram
Indonesian Journal of Electrical Engineering and Computer Science
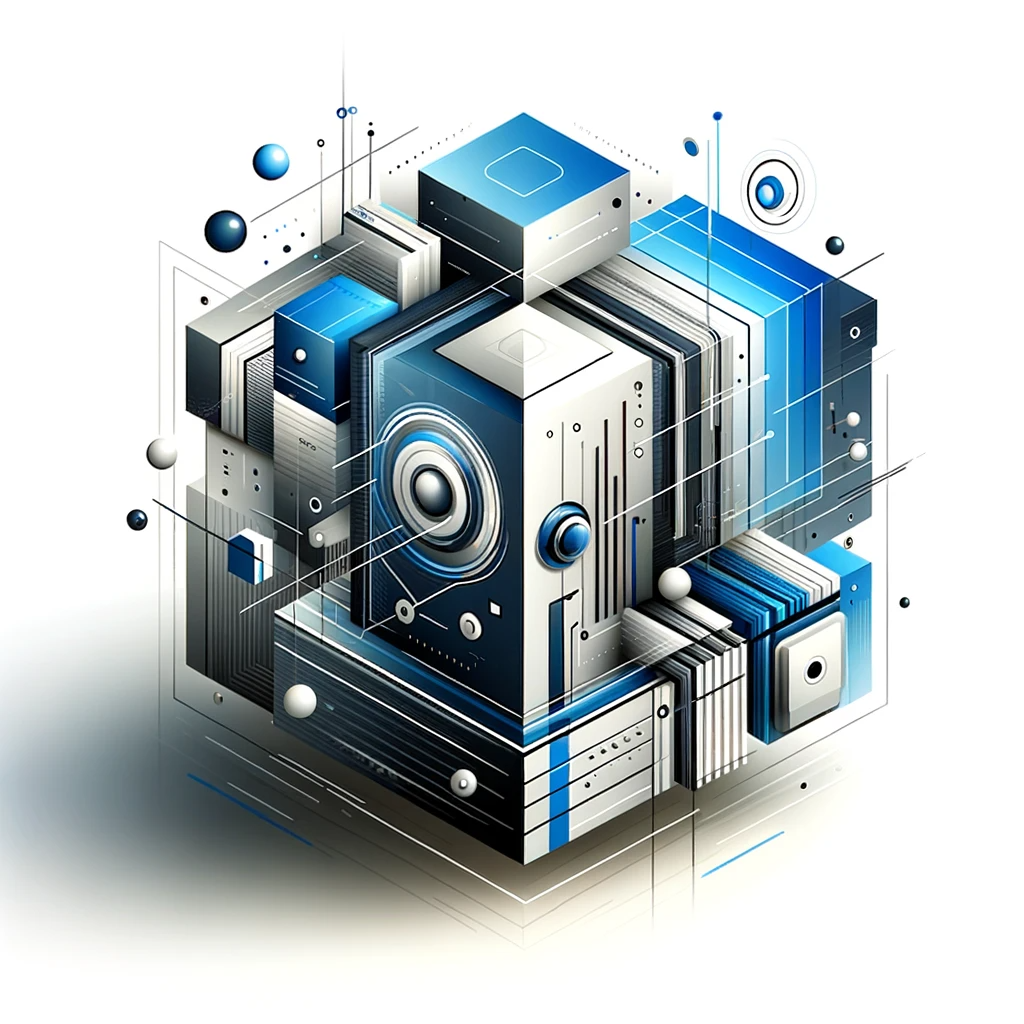
Abstract
Cardiovascular diseases (CVDs) continue to be the world’s most significant cause of morbidity and mortality. This paper introduces a unique hybrid learning model for CVD detection using advanced deep learning (DL) methods. The proposed method combines the potent feature extraction powers of the EfficientNet pre-trained model with attention mechanisms and graph convolutional networks (GCNs) for improved performance. First, rich representations from cardiovascular electrocardiogram (ECG) data extract using the EfficientNet architecture as a feature extractor. Using a large dataset of cardiovascular ECG images, you can fine-tune the pre-trained EfficientNet model with Pipeline to make it more suitable for disease identification. Including attention techniques that allow the network to focus on informative regions within the input, ECG images enhanced the model’s discriminative capacity. The model can attend to the salient areas selectively linked with CVD path physiology through dynamic attention processes. More accurate predictions result from this attention-based refining, strengthening the model’s ability to identify significant patterns suggestive of cardiovascular problems. GCN aims to link the natural structure in cardiovascular data. It can efficiently capture complex interactions and dependencies among various data pieces by expressing medical data as graphs, where nodes correspond to image regions, and edges imply spatial connections. Combining GCN into the proposed hybrid learning architecture facilitates extracting contextual information from local and global sources, augmenting the model’s accuracy.
Discover Our Library
Embark on a journey through our expansive collection of articles and let curiosity lead your path to innovation.
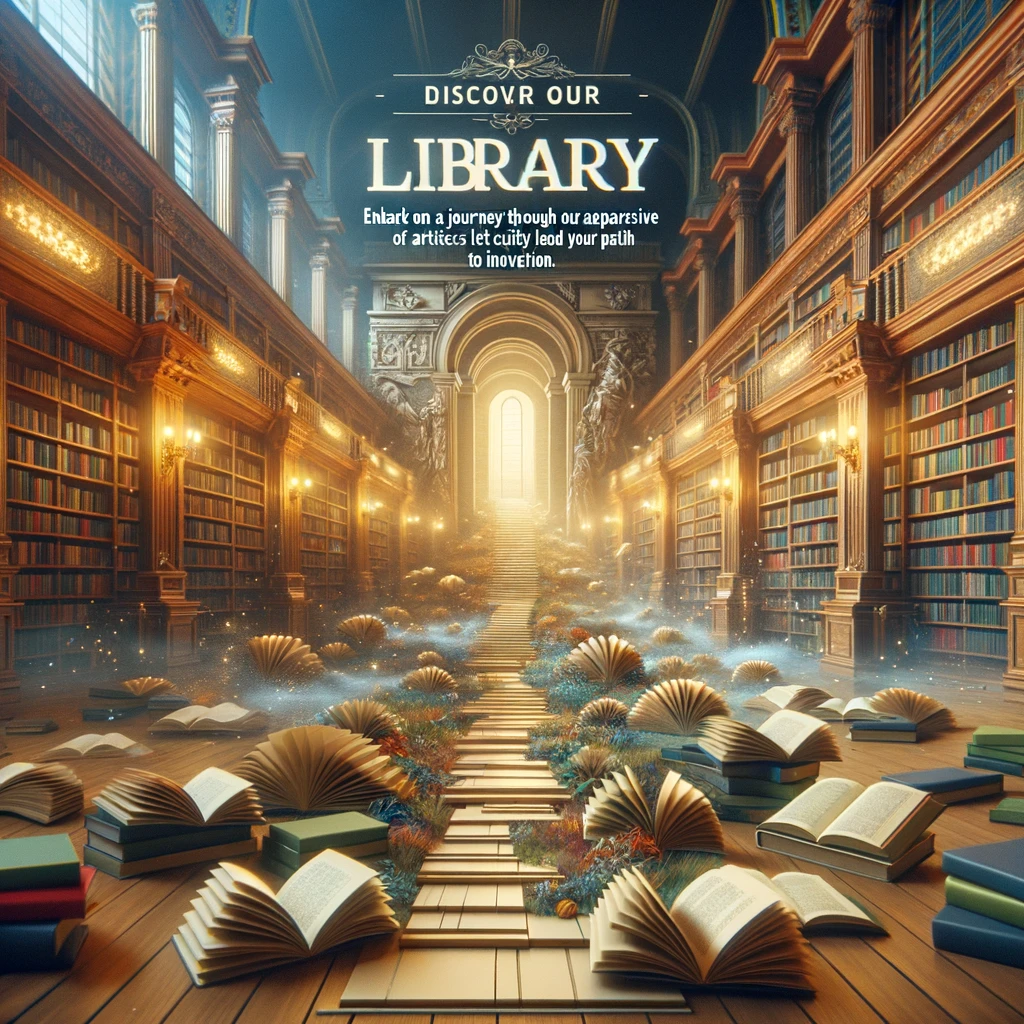