Enhancing SDN security using ensemble-based machine learning approach for DDoS attack detection
Indonesian Journal of Electrical Engineering and Computer Science
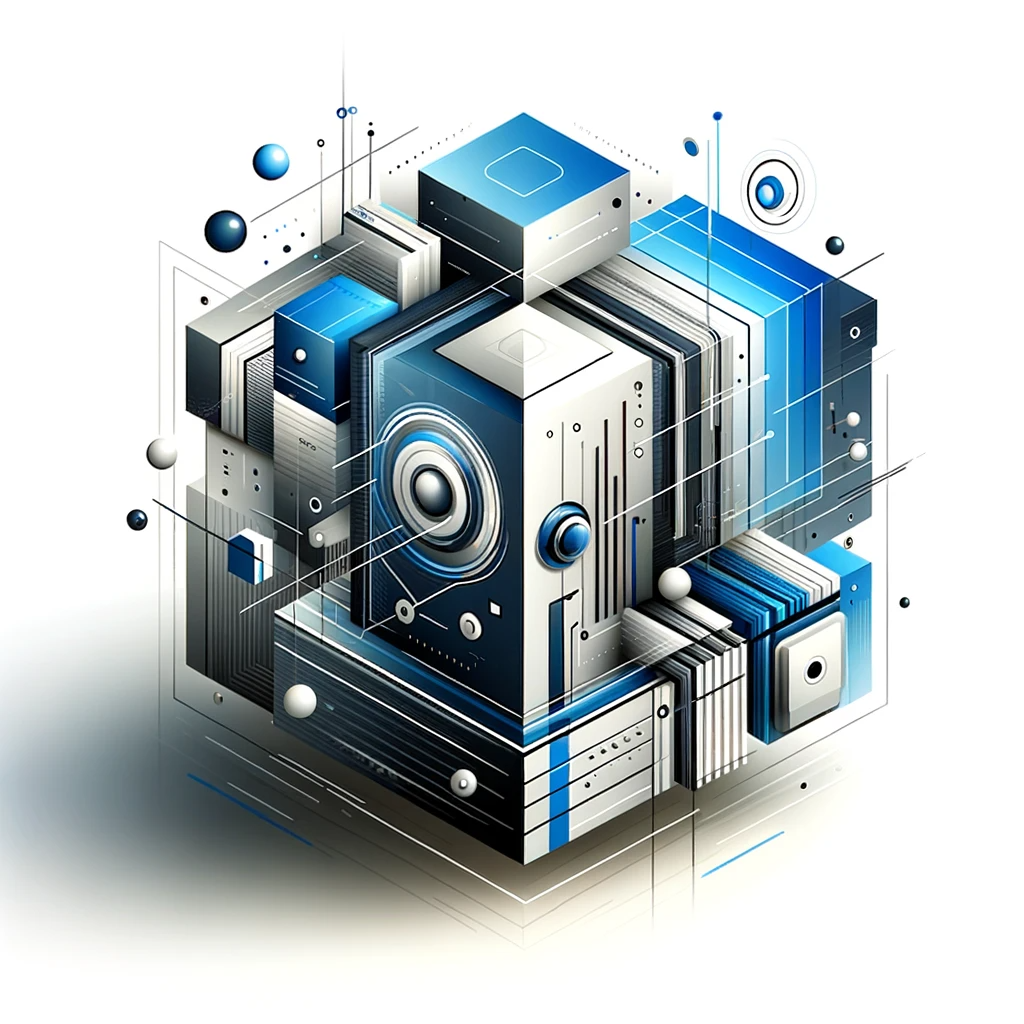
Abstract
Software-defined networking (SDN) is a groundbreaking technology that transforms traditional network frameworks by separating the control plane from the data plane, thereby enabling flexible and efficient network management. Despite its advantages, SDN introduces vulnerabilities, particularly distributed denial of service (DDoS) attacks. Existing studies have used single, hybrid, and ensemble machine learning (ML) techniques to address attacks, often relying on generated datasets that cannot be tested because of accessibility issues. A major contribution of this study is the creation of a novel, publicly accessible dataset, and benchmarking the proposed approach against existing public datasets to demonstrate its effectiveness. This paper proposes a novel approach that combines ensemble learning models with principal component analysis (PCA) for feature selection. The integration of ensemble learning models enhances predictive performance by leveraging multiple algorithms to improve accuracy and robustness. The results showed that the ensemble of random forests (ENRF) model achieved the highest performance across all metrics with 100% accuracy, precision, recall, and F1-score. This study provides a comprehensive solution to the limitations of existing models by offering superior performance, as evidenced by the comparative analysis, establishing this approach as the best among the evaluated models.
Discover Our Library
Embark on a journey through our expansive collection of articles and let curiosity lead your path to innovation.
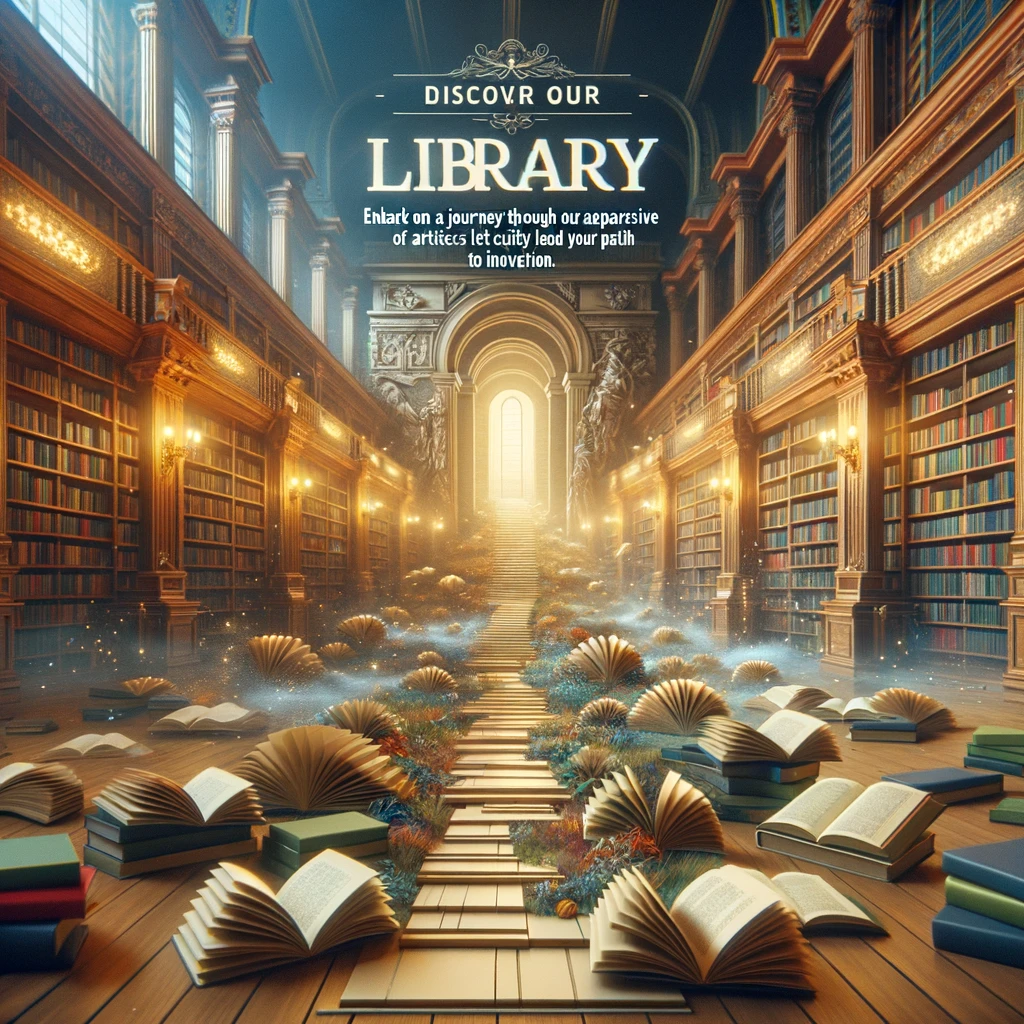