An innovative machine learning optimization-based data fusion strategy for distributed wireless sensor networks
Indonesian Journal of Electrical Engineering and Computer Science
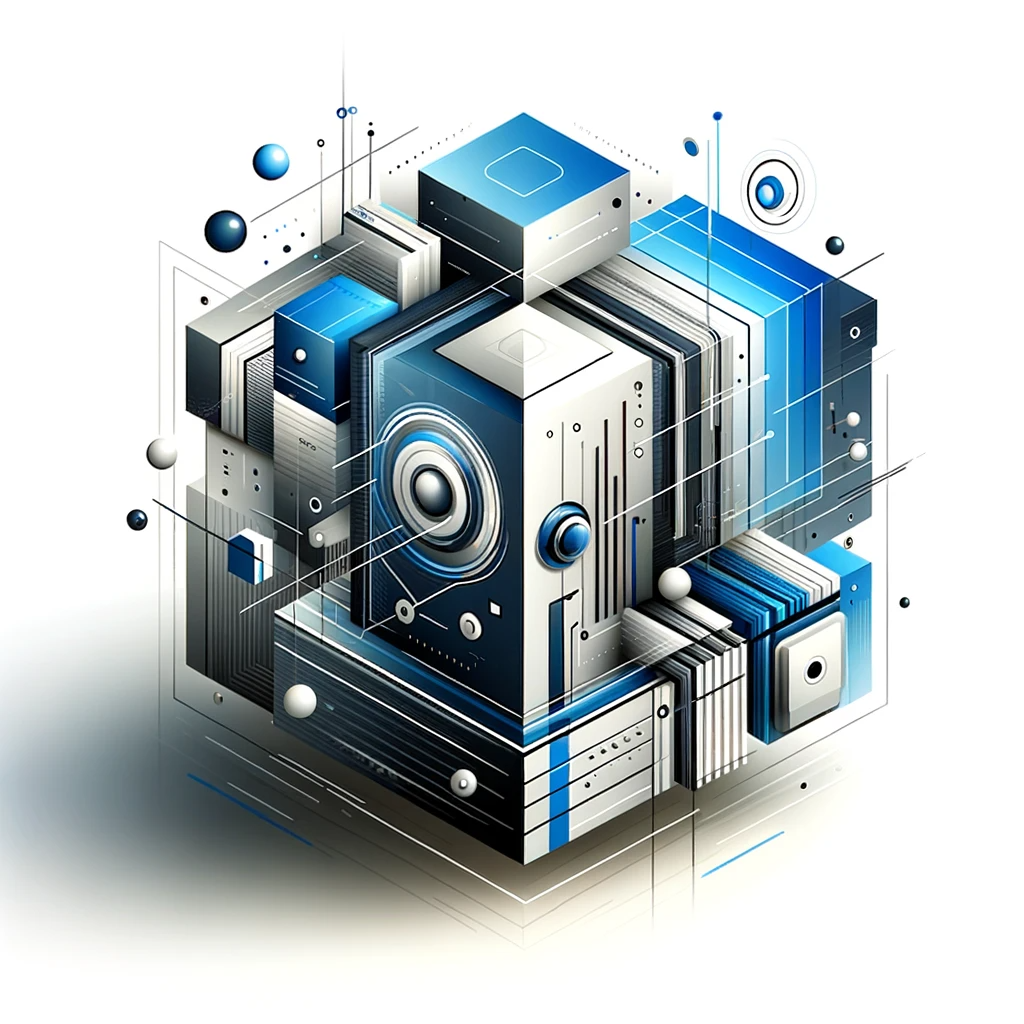
Abstract
Self-sufficient sensors scattered over different regions of the world comprise distributed wireless sensor networks (DWSNs), which track a range of environmental and physical factors such as pressure, temperature, vibration, sound, motion, and pollution. The use of data fusion becomes essential for combining information from various sensors and system performance. In this study, we suggested the multi-class support vector machine (SDF-MCSVM) with synthetic minority over-sampling techniques (SMOTE) data fusion for wireless sensor network (WSN) performance. The dataset includes 1,334 instances of hourly averaged answers for 12 variables from an AIR quality chemical multisensor device. To create a balanced dataset, the unbalanced data was first pre-processed using the SMOTE. The grey wolf optimization (GWO) approach is then used to reduce features in an effort to improve the efficacy and efficiency of feature selection procedures. This method is applied to classify the fused feature vectors into multiple categories at once to improve classification performance in WSNs and address unbalance datasets. The result shows the proposed method reaches high precision, accuracy, F1-score, recall, and specificity. The computational complexity and processing time were decreased in the study by using the proposed method. This is great potential for accurate and timely data fusion in dispersed WSNs with the successful integration of data fusion technologies.
Discover Our Library
Embark on a journey through our expansive collection of articles and let curiosity lead your path to innovation.
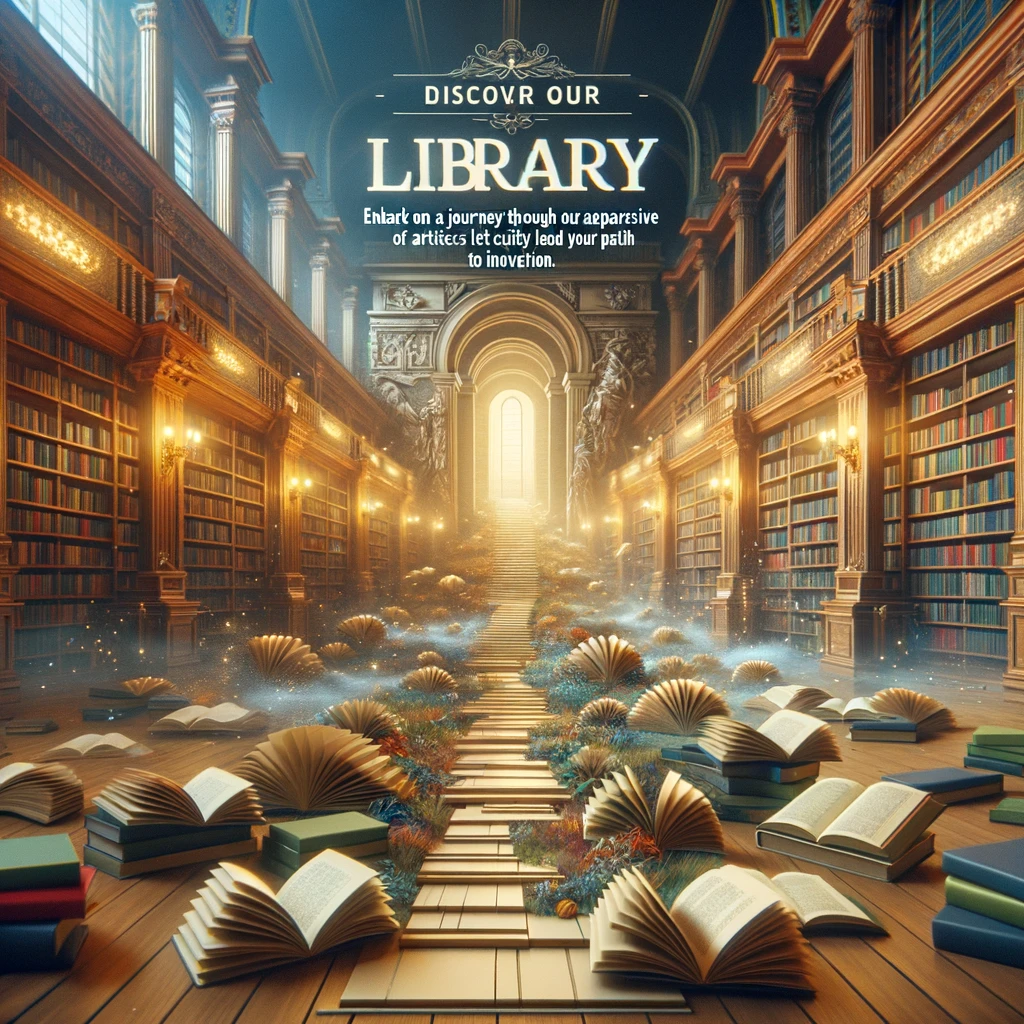