Predicting stock prices using ensemble learning techniques
International Journal of Electrical and Computer Engineering
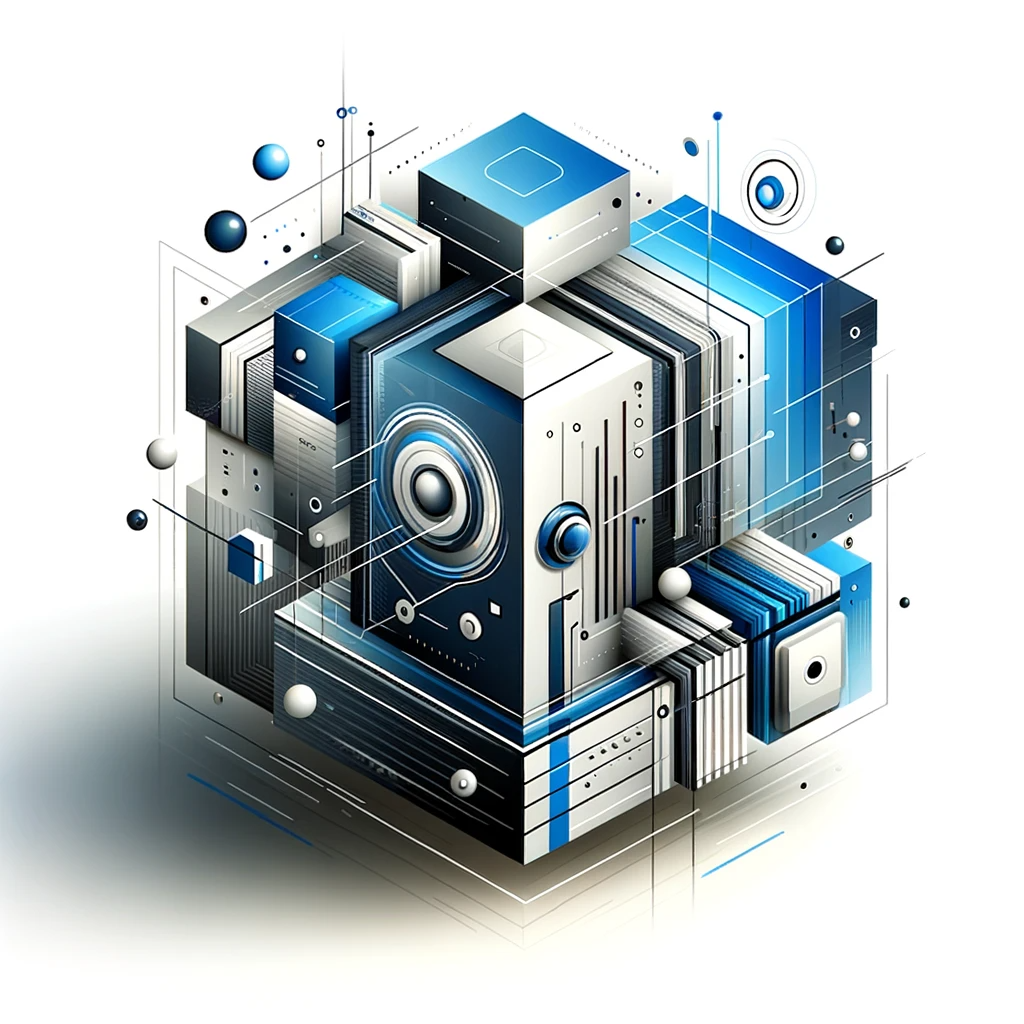
Abstract
Stock price prediction has grown in importance due to its role in determining the future worth of business shares. There are several approaches for stock price prediction that can be classified into machine learning, deep learning, and ensemble learning methods. To predict stock prices, we proposed collecting a dataset for different well-known stocks, e.g., Microsoft. The utilized datasets consist of two parts; the first part contains a set of tweets for the stocks under investigation in this study which were collected from the X social media platform and the other part contains the stock prices. Sentimental features of the tweets were extracted and merged with the stock price changes. Then, we framed the problem as a regression task. we aim to analyze the performance gap between ensemble learning and other machine learning (ML) and deep learning (DL) models for predicting stock prices based on tweets. In this context, different ensemble learning models were proposed to predict the price change of each stock. Besides, several machine learning and deep learning models were used for comparison purposes. Several evaluation metrics were utilized to evaluate the performance of the proposed models. The experimental results proved that the stacking regressor model outperformed the other models.
Discover Our Library
Embark on a journey through our expansive collection of articles and let curiosity lead your path to innovation.
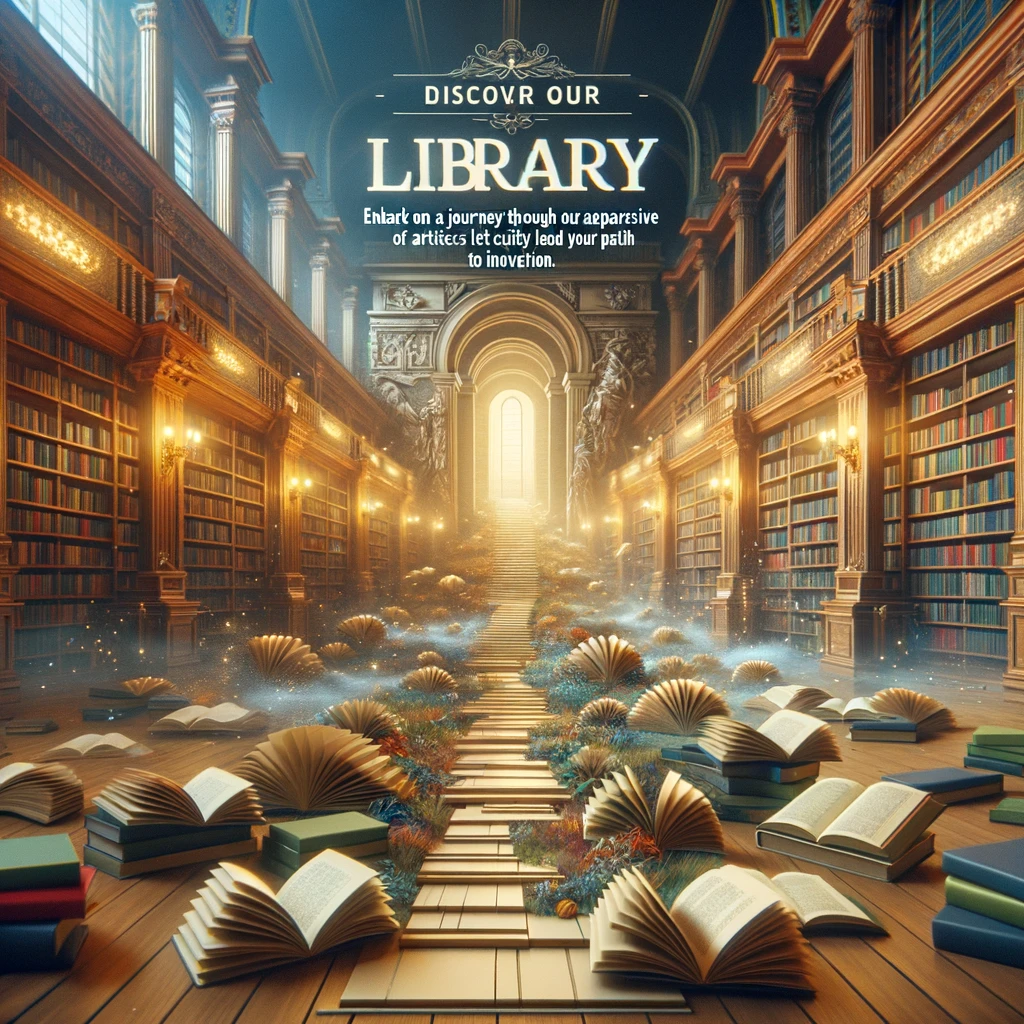