Machine learning-based classification of corn seed viability using electrical impedance spectroscopy
Indonesian Journal of Electrical Engineering and Computer Science
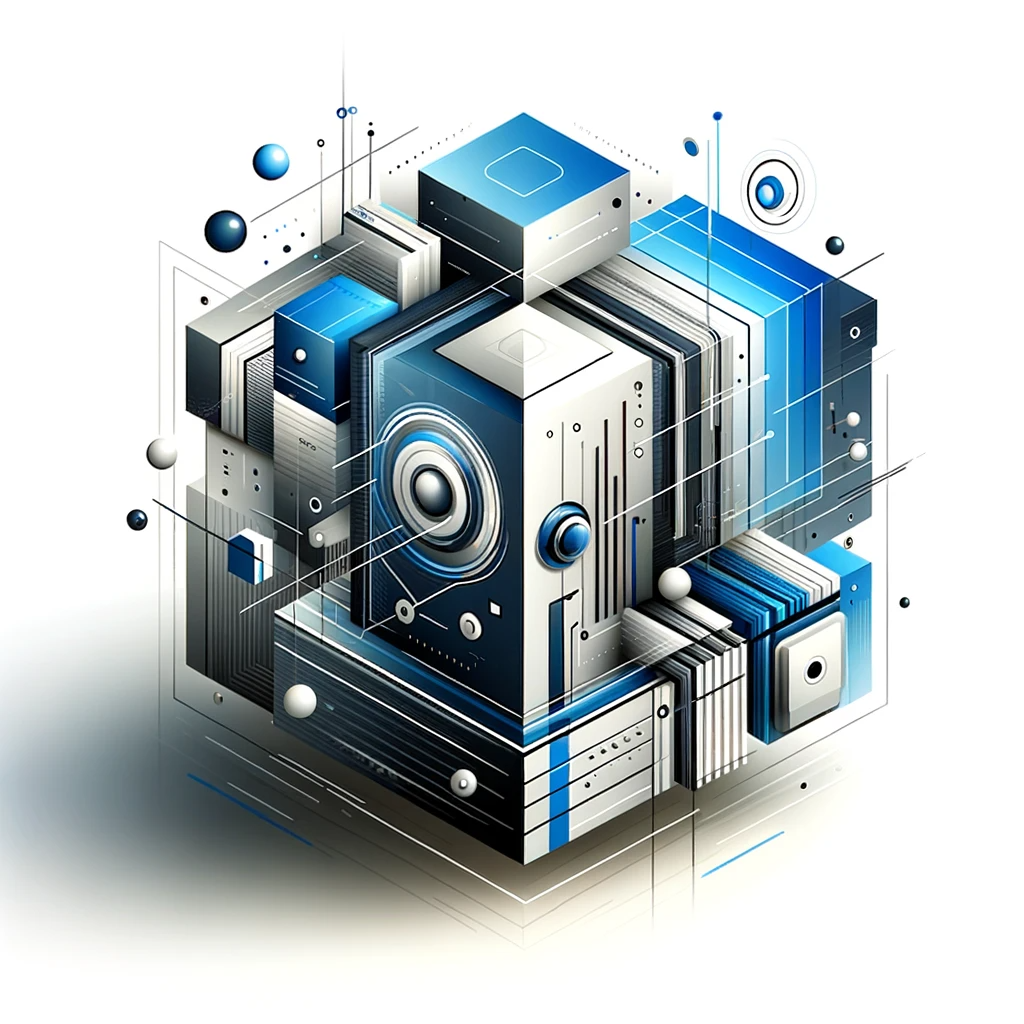
Abstract
Corn (Zea mays L.), an essential global commodity, plays an ever-increasing role in agri-food systems. To support growing demand, rapid and noninvasive methods for determining seed germination rates are crucial alongside invasive techniques such as dissection, germination paper tests, and chemical assays. This study introduces electrical impedance spectroscopy (EIS) as a novel, non-invasive approach for classifying viable and non-viable corn seeds. Non-viable corn seeds were prepared by exposing them to 100 °C convection heat for 30 minutes. Impedance spectra were measured using the EVAL-AD5933EBZ evaluation board from 400 kHz to 1 MHz frequency range within 30 seconds. Furthermore, a comparison of six optimized supervised machine learning (ML) algorithms, including shallow and deep networks, was performed, setting this apart from other studies. The trained model was deployed to assess the viability of new seed samples effectively. Key impedance metrics, including their frequencies, were extracted to train and test the algorithms. The regression tree (RTree) model outperformed deep learning classifiers, achieving 95% accuracy, 90% precision, and 100% sensitivity. The results indicated an upward trend in viable seed impedance, increasing by 0.000164 Ω/Hz, peaking at 990 kHz. This approach offers a rapid, non-invasive solution for seed viability assessment, with significant potential to enhance agricultural productivity.
Discover Our Library
Embark on a journey through our expansive collection of articles and let curiosity lead your path to innovation.
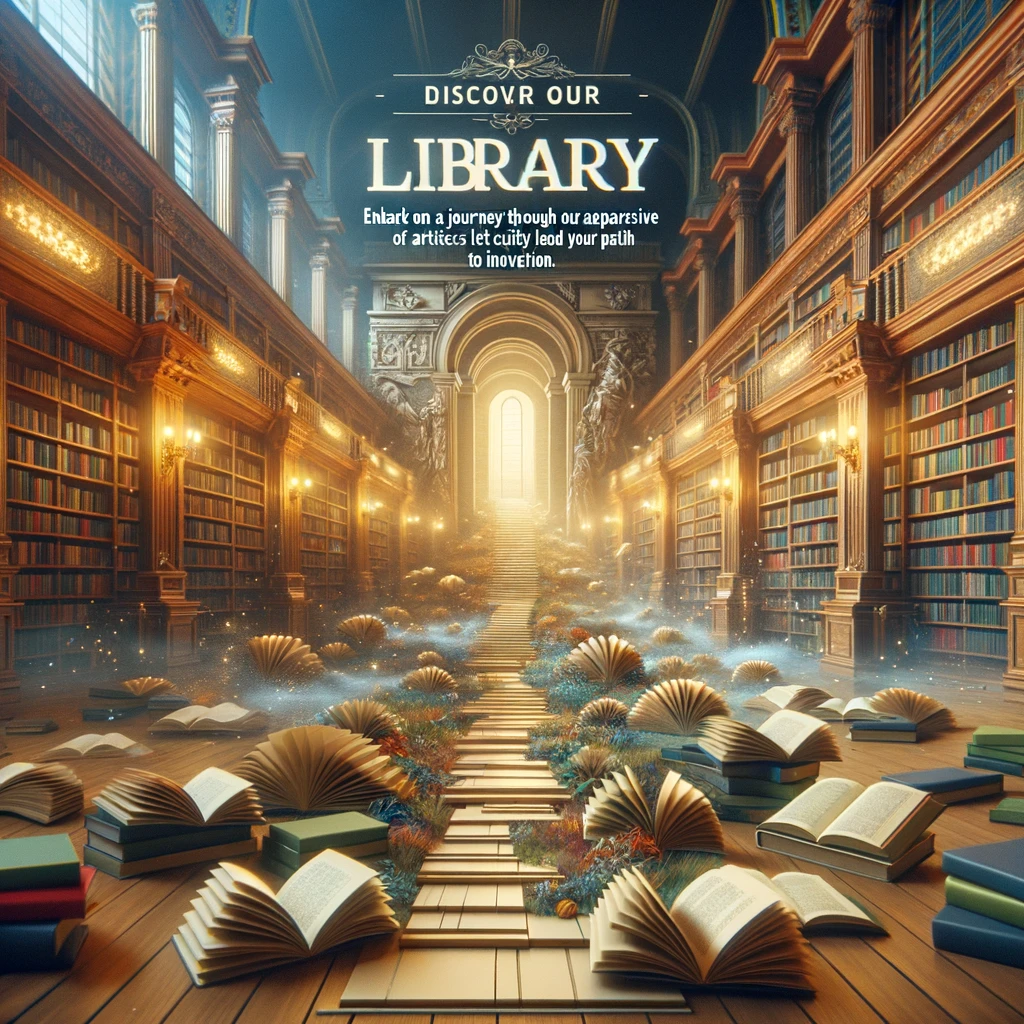